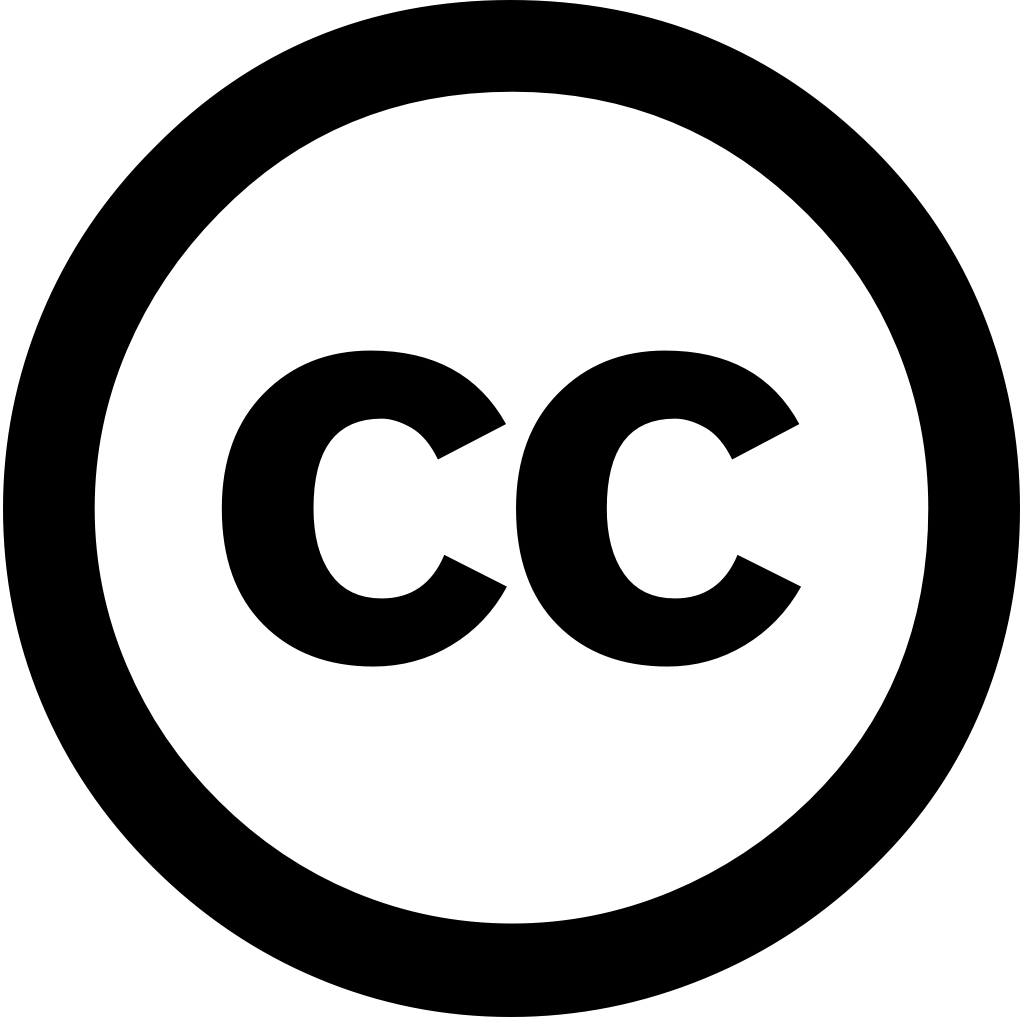
iScience, Journal Year: 2024, Volume and Issue: 27(12), P. 111230 - 111230
Published: Oct. 23, 2024
Language: Английский
iScience, Journal Year: 2024, Volume and Issue: 27(12), P. 111230 - 111230
Published: Oct. 23, 2024
Language: Английский
Military Medical Research, Journal Year: 2023, Volume and Issue: 10(1)
Published: Sept. 26, 2023
Abstract Auscultation is crucial for the diagnosis of respiratory system diseases. However, traditional stethoscopes have inherent limitations, such as inter-listener variability and subjectivity, they cannot record sounds offline/retrospective or remote prescriptions in telemedicine. The emergence digital has overcome these limitations by allowing physicians to store share consultation education. On this basis, machine learning, particularly deep enables fully-automatic analysis lung that may pave way intelligent stethoscopes. This review thus aims provide a comprehensive overview learning algorithms used sound emphasize significance artificial intelligence (AI) field. We focus on each component learning-based systems, including task categories, public datasets, denoising methods, and, most importantly, existing i.e., state-of-the-art approaches convert into two-dimensional (2D) spectrograms use convolutional neural networks end-to-end recognition diseases abnormal sounds. Additionally, highlights current challenges field, variety devices, noise sensitivity, poor interpretability models. To address reproducibility also provides scalable flexible open-source framework standardize algorithmic workflow solid basis replication future extension: https://github.com/contactless-healthcare/Deep-Learning-for-Lung-Sound-Analysis .
Language: Английский
Citations
26Structures, Journal Year: 2025, Volume and Issue: 72, P. 108245 - 108245
Published: Jan. 28, 2025
Language: Английский
Citations
1Sensors, Journal Year: 2024, Volume and Issue: 24(2), P. 682 - 682
Published: Jan. 21, 2024
Early identification of respiratory irregularities is critical for improving lung health and reducing global mortality rates. The analysis sounds plays a significant role in characterizing the system's condition identifying abnormalities. main contribution this study to investigate performance when input data, represented by cochleogram, used feed Vision Transformer architecture, since classifier combination first time it has been applied adventitious sound classification our knowledge. Although ViT shown promising results audio tasks applying self attention spectrogram patches, we extend approach which captures specific spectro-temporal features sounds. proposed methodology evaluated on ICBHI dataset. We compare with other state art CNN approaches using spectrogram, Mel frequency cepstral coefficients, constant Q transform, cochleogram as data. Our confirm superior combining ViT, highlighting potential reliable classification. This contributes ongoing efforts developing automatic intelligent techniques aim significantly augment speed effectiveness disease detection, thereby addressing need medical field.
Language: Английский
Citations
6Energy, Journal Year: 2024, Volume and Issue: 308, P. 132721 - 132721
Published: Aug. 10, 2024
Language: Английский
Citations
6Multimedia Tools and Applications, Journal Year: 2024, Volume and Issue: 83(35), P. 82871 - 82903
Published: March 12, 2024
Language: Английский
Citations
4Applied Soft Computing, Journal Year: 2025, Volume and Issue: 170, P. 112678 - 112678
Published: Jan. 9, 2025
Language: Английский
Citations
0Applied Intelligence, Journal Year: 2025, Volume and Issue: 55(6)
Published: March 20, 2025
Language: Английский
Citations
0Applied Sciences, Journal Year: 2025, Volume and Issue: 15(10), P. 5313 - 5313
Published: May 9, 2025
Accurate classification of biomedical signals is essential for advancing non-invasive diagnostic techniques and improving clinical decision-making. This study introduces a deep learning-augmented spectrogram analysis framework classifying into eight anatomically distinct regions, thereby addressing significant deficiency in automated signal interpretation. The proposed approach leverages fine-tuned ResNet50 model, pre-trained on ImageNet, adapted single-channel input to ensure robust feature extraction high accuracy. Spectrograms derived from palpation percussion were preprocessed grayscale images optimized through data augmentation hyperparameter tuning enhance the model’s generalization. experimental results demonstrate accuracy 93.37%, surpassing that conventional methods highlighting effectiveness learning processing. bridges gap between machine applications, enabling an interpretable region-specific system enhances precision. Future work will explore cross-domain generalization, multi-modal medical integration, real-time deployment applications. findings establish advancement diagnostics, demonstrating potential refine automate practice.
Language: Английский
Citations
0International Journal of Power Electronics and Drive Systems/International Journal of Electrical and Computer Engineering, Journal Year: 2023, Volume and Issue: 13(4), P. 3977 - 3977
Published: April 3, 2023
<span lang="EN-US">This paper presents a study on an embedded spirometer using the low-cost MPX5100DP pressure sensor and Arduino Uno board to measure air exhaled flow rate calculate force vital capacity (FVC), forced expiratory volume in 1 s (FEV1), FEV1/FVC ratio of human lungs volume. The was measured from differential sections mouthpiece tube venturi effect equation. This constructed resulted 96.27% FVC reading accuracy with deviation 0.09 L 98.05% FEV1 0.05 compared spirometry. integrates HC-05 Bluetooth module for spirometry data transceiving smartphone display recording Android application further chronic obstructive pulmonary disease (COPD) diagnosis.</span>
Language: Английский
Citations
6Biomedical Signal Processing and Control, Journal Year: 2024, Volume and Issue: 93, P. 106191 - 106191
Published: March 7, 2024
Language: Английский
Citations
2