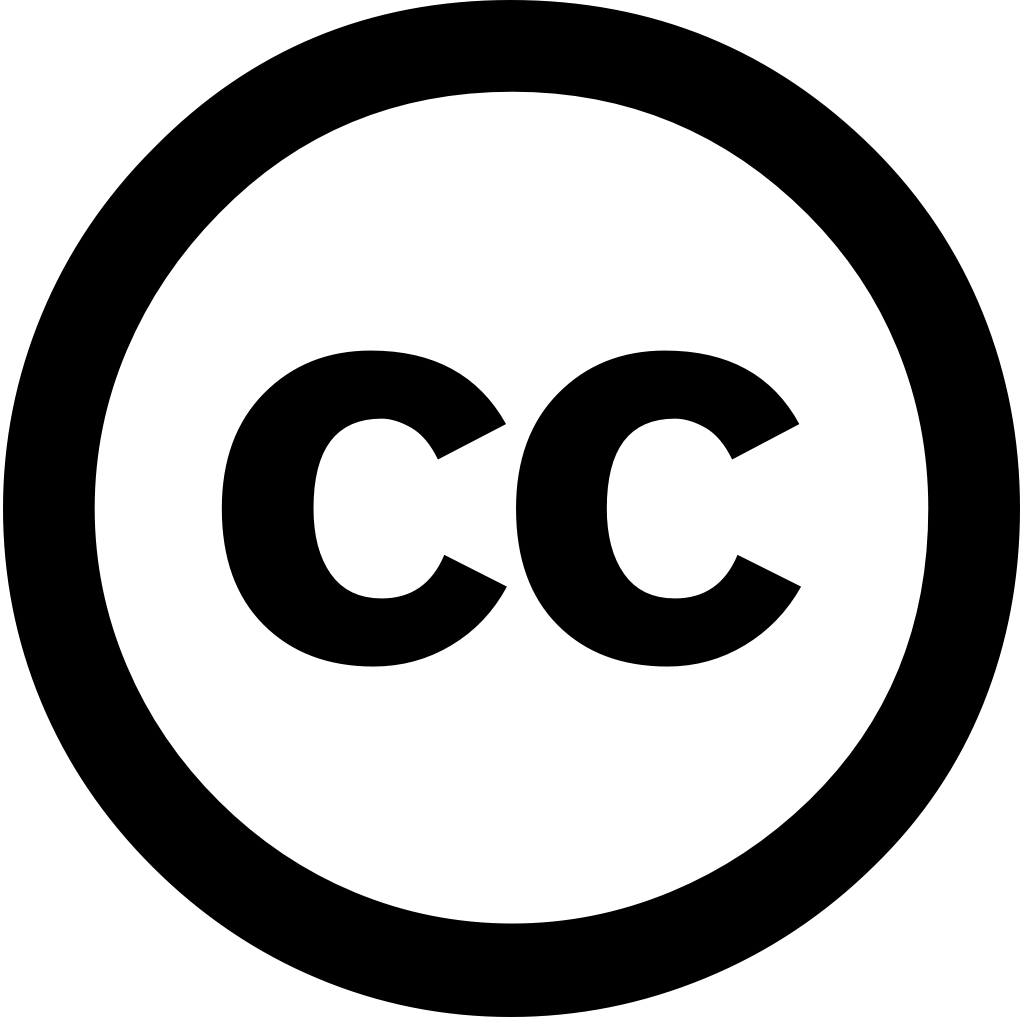
Health Science Reports, Journal Year: 2025, Volume and Issue: 8(5)
Published: May 1, 2025
ABSTRACT Purpose Alzheimer's disease (AD) is a severe neurological that significantly impairs brain function. Timely identification of AD essential for appropriate treatment and care. This comprehensive review intends to examine current developments in deep learning (DL) approaches with neuroimaging diagnosis, where popular imaging types, reviews well‐known online accessible data sets, describes different algorithms used DL the correct initial evaluation are presented. Significance Conventional diagnostic techniques, including medical evaluations cognitive assessments, usually not identify stages Alzheimer's. Neuroimaging methods, when integrated have demonstrated considerable potential enhancing diagnosis categorization AD. models received significant interest due their capability its early phases automatically, which reduces mortality rate cost Method An extensive literature search was performed leading scientific databases, concentrating on papers published from 2021 2025. Research leveraging techniques such as magnetic resonance (MRI), positron emission tomography, functional (fMRI), so forth. The complies Preferred Reporting Items Systematic Reviews Meta‐Analyses (PRISMA) guidelines. Results Current show CNN‐based especially those utilizing hybrid transfer frameworks, outperform conventional methods. employing combination multimodal has enhanced precision. Still, challenges method interpretability, heterogeneity, limited exist issues. Conclusion considerably improved accuracy reliability neuroimaging. Regardless issues accessibility adaptability, studies into interpretability fusion provide clinical application. Further research should concentrate standardized rigorous validation architectures, understandable AI methodologies enhance effectiveness methods prediction.
Language: Английский