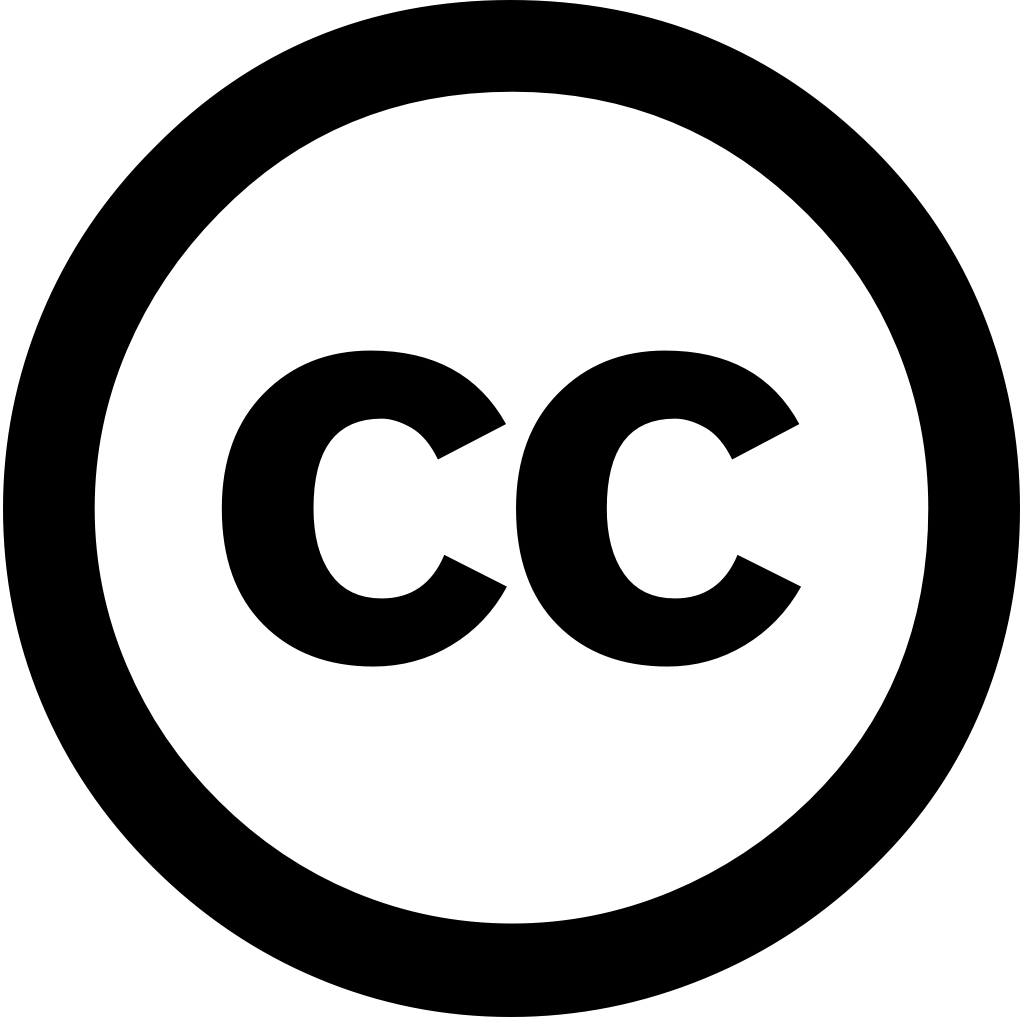
Building and Environment, Journal Year: 2024, Volume and Issue: 270, P. 112516 - 112516
Published: Dec. 30, 2024
Language: Английский
Building and Environment, Journal Year: 2024, Volume and Issue: 270, P. 112516 - 112516
Published: Dec. 30, 2024
Language: Английский
Building and Environment, Journal Year: 2025, Volume and Issue: unknown, P. 113057 - 113057
Published: April 1, 2025
Language: Английский
Citations
0Forests, Journal Year: 2025, Volume and Issue: 16(5), P. 836 - 836
Published: May 18, 2025
PM2.5 emissions significantly impact atmospheric environments and human health in the context of forest fires. However, research on from fires remains insufficient. This study systematically investigated emission characteristics broadleaf tree combustion through controlled experiments examining three key factors: species variation (Acer tegmentosum [AT], Acer ukurunduense [AU], pictum [AP], Tilia amurensis [TA], Phellodendron amurense [PA], Ulmus davidiana [UD], laciniata [UL], Prunus padus [PP], maackii [PM]), moisture content (0%–20%), phenological stages (budding [A], growing [B], defoliation [C]). The results demonstrated: (1) Significant interspecies differences, with UL showing lowest, PM highest emissions; (2) A unimodal moisture—emission relationship peaking at 15% across most species, while AT, exhibited unique linear responses; (3) Distinct patterns, including triphasic fluctuations during phases. LightGBM model effectively predicted (R2 = 0.97), identifying (36.2% importance) (21.6%) as dominant factors. These findings provide critical data for wildfire modeling highlight need species-specific parameters air quality forecasts.
Language: Английский
Citations
0Building and Environment, Journal Year: 2024, Volume and Issue: unknown, P. 112233 - 112233
Published: Oct. 1, 2024
Language: Английский
Citations
2Building and Environment, Journal Year: 2024, Volume and Issue: 267, P. 112305 - 112305
Published: Nov. 10, 2024
Language: Английский
Citations
1Buildings, Journal Year: 2024, Volume and Issue: 14(12), P. 4024 - 4024
Published: Dec. 18, 2024
The relationship between coronary heart disease (CHD) and complex urban built environments remains a subject of considerable uncertainty. development predictive models via machine learning to explore the underlying mechanisms this association, as well formulation intervention policies planning strategies, has emerged pivotal area research. A cross-sectional dataset hospital admissions for CHD over course year from in Dalian City, China, was assembled matched with multi-source environment data residential addresses. This study evaluates five models, including decision tree (DT), random forest (RF), eXtreme gradient boosting (XGBoost), multi-layer perceptron (MLP), support vector (SVM), compares them multiple linear regression models. results show that DT, RF, XGBoost exhibit superior capabilities, all R2 values exceeding 0.70. DT model performed best, an value 0.818, best performance based on metrics such MAE MSE. Additionally, using explainable AI techniques, reveals contribution different factors identifies significant influencing cold regions, ranked age, Digital Elevation Model (DEM), house price (HP), sky view factor (SVF), interaction factors. Stratified analyses by age gender variations groups: those under 60 years old, Road Density is most influential factor; 61–70 group, top 71–80 81 building height leading males, GDP females, factor. explores feasibility predicting risk regions provides comprehensive methodology workflow cardiovascular refined neighborhood-level factors, offering scientific construction sustainable healthy cities.
Language: Английский
Citations
0Building and Environment, Journal Year: 2024, Volume and Issue: 270, P. 112516 - 112516
Published: Dec. 30, 2024
Language: Английский
Citations
0