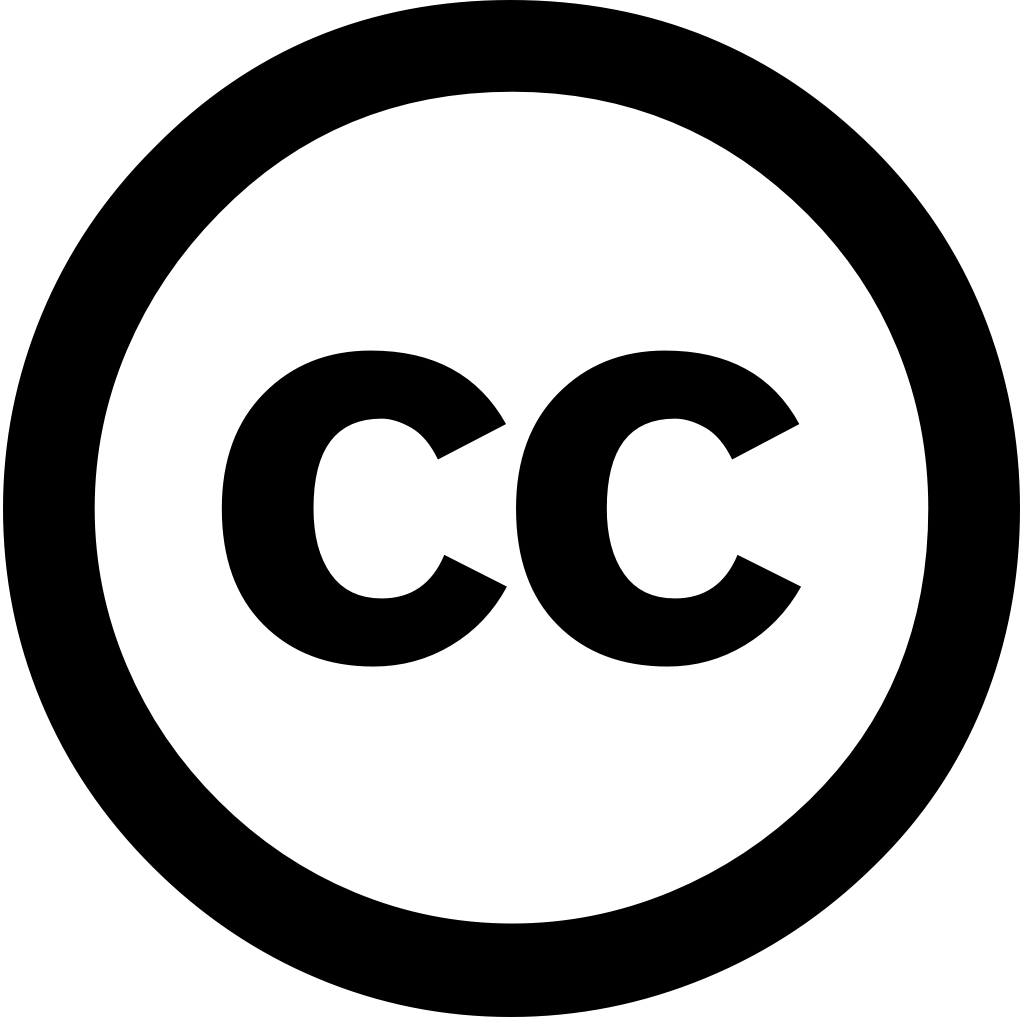
Waste Management, Journal Year: 2024, Volume and Issue: 191, P. 70 - 80
Published: Nov. 7, 2024
Language: Английский
Waste Management, Journal Year: 2024, Volume and Issue: 191, P. 70 - 80
Published: Nov. 7, 2024
Language: Английский
Minerals, Journal Year: 2025, Volume and Issue: 15(5), P. 483 - 483
Published: May 5, 2025
The performance of image processing is crucial for accurately sorting antimony ore, yet several challenges persist. Existing segmentation methods struggle with X-ray ore images that contain high noise and interference. Additionally, traditional classification primarily utilize single physical properties, such as the R-value, leading to low accuracy. To address issues, this paper proposes an improved method based on concave detection. This involves obtaining a binary through adaptive threshold segmentation, extracting contour, detecting points using advanced techniques. influence interfering minimized three-wire method, while are reduced morphological operations area calculations. results in accurate adherent ore. For classification, introduces training combines transfer learning shallow partial initialization. Transfer employed mitigate limited datasets when deep models. pre-trained model then partially re-initialized according tailored strategy. Finally, fine-tuning performed dataset achieve optimal results. Experimental show proposed achieves (96.27% correct rate). can effectively release some redundant parameters pre-training model, has better target (86.76% accuracy). Both superior methods.
Language: Английский
Citations
0Remote Sensing, Journal Year: 2023, Volume and Issue: 15(18), P. 4463 - 4463
Published: Sept. 11, 2023
Floods are natural events that can have a significant impacts on the economy and society of affected regions. To mitigate their effects, it is crucial to conduct rapid accurate assessment damage take measures restore critical infrastructure as quickly possible. Remote sensing monitoring using artificial intelligence promising tool for estimating extent flooded areas. However, flood still presents some challenges due varying weather conditions cloud cover limit use visible satellite data. Additionally, observations may not always correspond peak, essential estimate both volume flood. address these challenges, we propose methodology combines multispectral radar data utilizes deep neural network pipeline analyze available remote different dates. This approach allows us depth calculate its volume. Our study uses Sentinel-1, Sentinel-2 data, Digital Elevation Model (DEM) measurements provide reliable results. validate developed approach, consider event occurred in 2021 Ushmun. As result, succeeded evaluate at 0.0087 km3. Overall, our proposed offers simple yet effective networks. It has potential improve accuracy speed assessments, which aid timely response recovery efforts
Language: Английский
Citations
8Computers & Geosciences, Journal Year: 2024, Volume and Issue: 184, P. 105532 - 105532
Published: Jan. 11, 2024
Language: Английский
Citations
2Minerals, Journal Year: 2024, Volume and Issue: 14(2), P. 186 - 186
Published: Feb. 10, 2024
This article presents developed hardware and software solutions based on the application of machine vision technology. The were created in order to generate a database HSV-color value for main ore types, host rocks, minerals define criteria in-process identification Khibiny apatite testing walls blasting boreholes. ensures multi-parametric assessment optical characteristics samples located their surface. designed solution allows user control measurement process; systematize description textural structural features sample under study; process images core resultant ores rocks massif constituent will provide an opportunity search mineral mixture.
Language: Английский
Citations
1Published: May 7, 2024
Deep learning has increasingly been employed to identify minerals. However, deep can only be used minerals in the distribution of training set, while any mineral outside spectrum set is inevitably categorized erroneously within a predetermined class from set. To solve this problem, study introduces approach that amalgamates One-Class Support Vector Machines (OCSVM) with ResNet architecture for out-of-distribution detection. Initially, undergoes using comprising well-defined Subsequently, earlier layers trained are extract discriminative features under consideration. These extracted then become input OCSVM. When OCSVM discerns set's distribution, it triggers subsequent ResNet, facilitating accurate classification into one predefined categories encompassing known In event identifies out unequivocally as an unclassified or 'unknown' mineral. Empirical results substantiate method's capability concurrently maintaining commendably high accuracy rate 36 in-distribution
Language: Английский
Citations
1Geoinformatika, Journal Year: 2024, Volume and Issue: 3, P. 53 - 63
Published: Sept. 27, 2024
The article discusses technologies that can radically change the development of many areas at once: artificial intelligence, quantum technologies, big data, wireless communication distributed registry systems. authors consider a number promising near future currently have prospects for application in Earth sciences.
Language: Английский
Citations
1International Geology Review, Journal Year: 2024, Volume and Issue: unknown, P. 1 - 34
Published: Nov. 30, 2024
Language: Английский
Citations
1Minerals, Journal Year: 2024, Volume and Issue: 14(6), P. 627 - 627
Published: June 20, 2024
Deep learning has increasingly been used to identify minerals. However, deep can only be minerals within the distribution of training set, while any mineral outside spectrum set is inevitably categorized erroneously a predetermined class from set. To solve this problem, study introduces approach that combines One-Class Support Vector Machine (OCSVM) with ResNet architecture for out-of-distribution detection. Initially, undergoes using comprising well-defined Subsequently, first two layers obtained trained are employed extract discriminative features under consideration. These extracted then become input OCSVM. When OCSVM discerns in set’s distribution, it triggers subsequent ResNet, facilitating accurate classification into one predefined categories encompassing known In event identifies as an unclassified or ‘unknown’ mineral. Empirical results substantiate method’s capability concurrently maintaining commendably high accuracy rate 36 in-distribution
Language: Английский
Citations
0Waste Management, Journal Year: 2024, Volume and Issue: 191, P. 70 - 80
Published: Nov. 7, 2024
Language: Английский
Citations
0