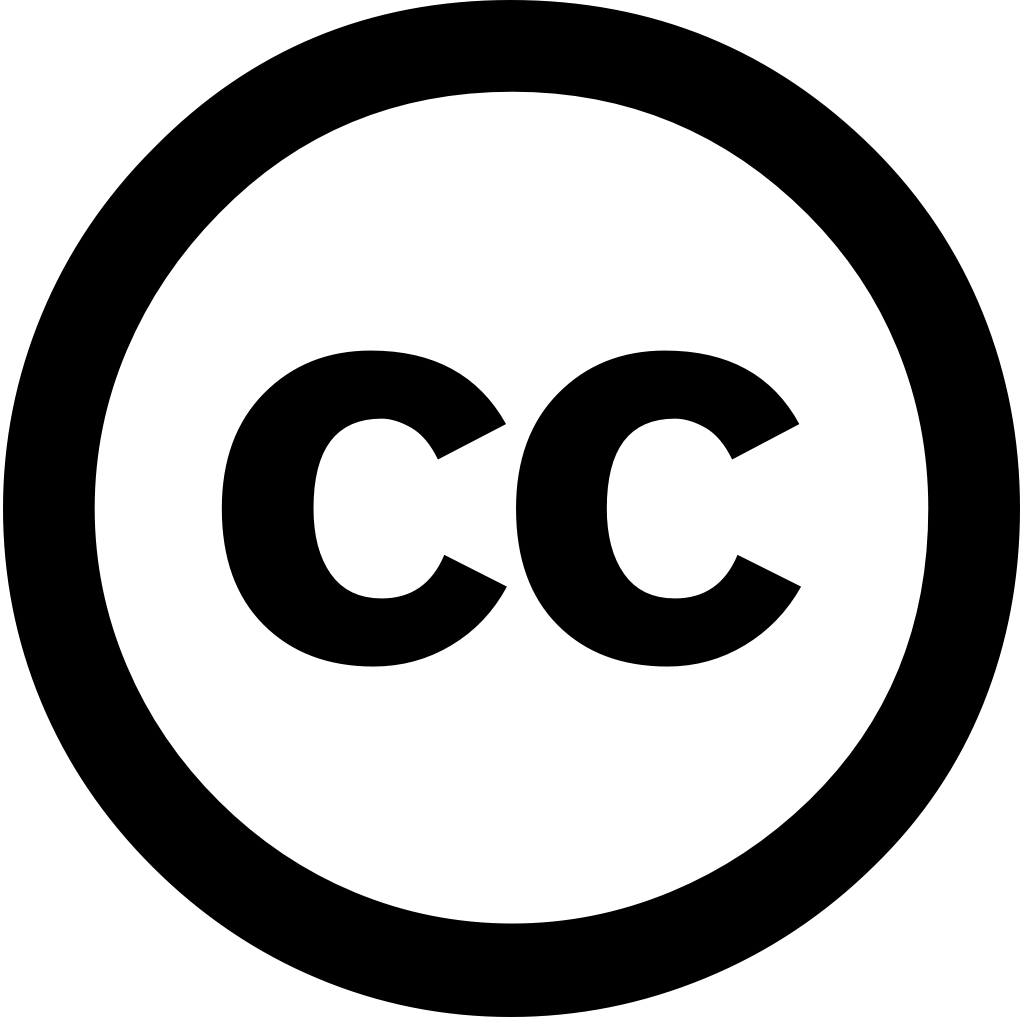
Remote Sensing, Journal Year: 2024, Volume and Issue: 16(19), P. 3671 - 3671
Published: Oct. 1, 2024
Texture features have been consistently overlooked in digital soil mapping, especially salinization mapping. This study aims to clarify how leverage texture information for monitoring through remote sensing techniques. We propose a novel method estimating salinity content (SSC) that combines spectral and from unmanned aerial vehicle (UAV) images. Reflectance, index, one-dimensional (OD) were extracted UAV Building on the features, we constructed two-dimensional (TD) three-dimensional (THD) indices. The technique of Recursive Feature Elimination (RFE) was used feature selection. Models estimation built using three distinct methodologies: Random Forest (RF), Partial Least Squares Regression (PLSR), Convolutional Neural Network (CNN). Spatial distribution maps then generated each model. effectiveness proposed is confirmed utilization 240 surface samples gathered an arid region northwest China, specifically Xinjiang, characterized by sparse vegetation. Among all indices, TDTeI1 has highest correlation with SSC (|r| = 0.86). After adding multidimensional information, R2 RF model increased 0.76 0.90, improvement 18%. models, outperforms PLSR CNN. model, which (SOTT), achieves RMSE 5.13 g kg−1, RPD 3.12. contributes 44.8% prediction, contributions TD THD indices 19.3% 20.2%, respectively. confirms great potential introducing semi-arid regions.
Language: Английский