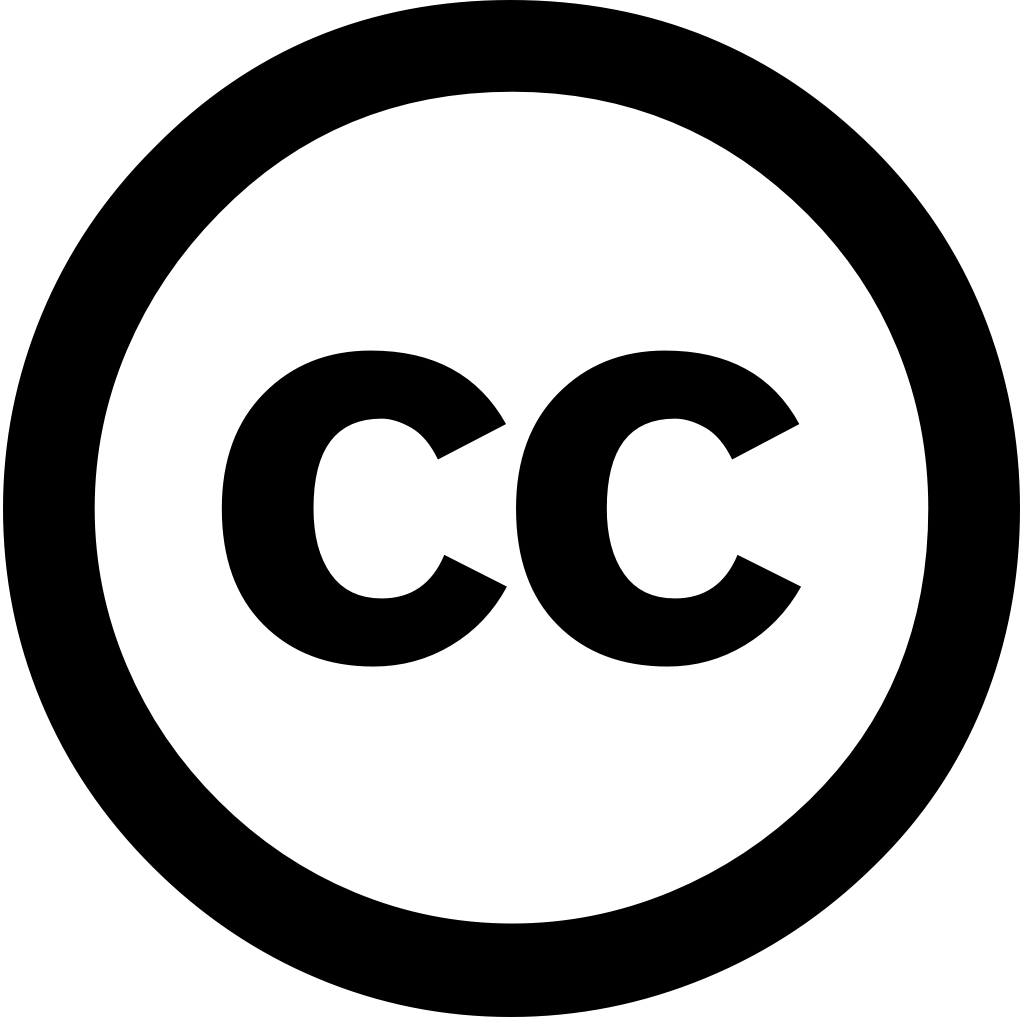
Agronomy, Journal Year: 2025, Volume and Issue: 15(3), P. 759 - 759
Published: March 20, 2025
The rapid and accurate acquisition of soil moisture (SM) information is essential. Although Unmanned Aerial Vehicle (UAV) remote sensing technology has made significant advancements in SM monitoring, existing studies predominantly focus on developing models tailored to specific regions. transferability these across different regions remains a considerable challenge. Therefore, this study proposes transfer learning-based framework, using two representative small agricultural watersheds (Hongxing region Woniutu region) Northeast China as case studies. This framework involves pre-training model source domain fine-tuning it with limited set target samples achieve high-precision inversion. evaluates the performance three algorithms: Random Forest (RF), Convolutional Neural Network (CNN), Long Short-Term Memory (LSTM) network. Results show that fine-tuned significantly mitigates decline prediction accuracy caused by regional differences. LSTM achieved highest retrieval accuracy, following results: 10% (R = 0.615, RRMSE 15.583%), 30% 0.682, 13.97%), 50% 0.767, 16.321%). Among models, exhibited most improvement best transferability. underscores potential learning for enhancing cross-regional monitoring providing valuable insights future UAV-based monitoring.
Language: Английский