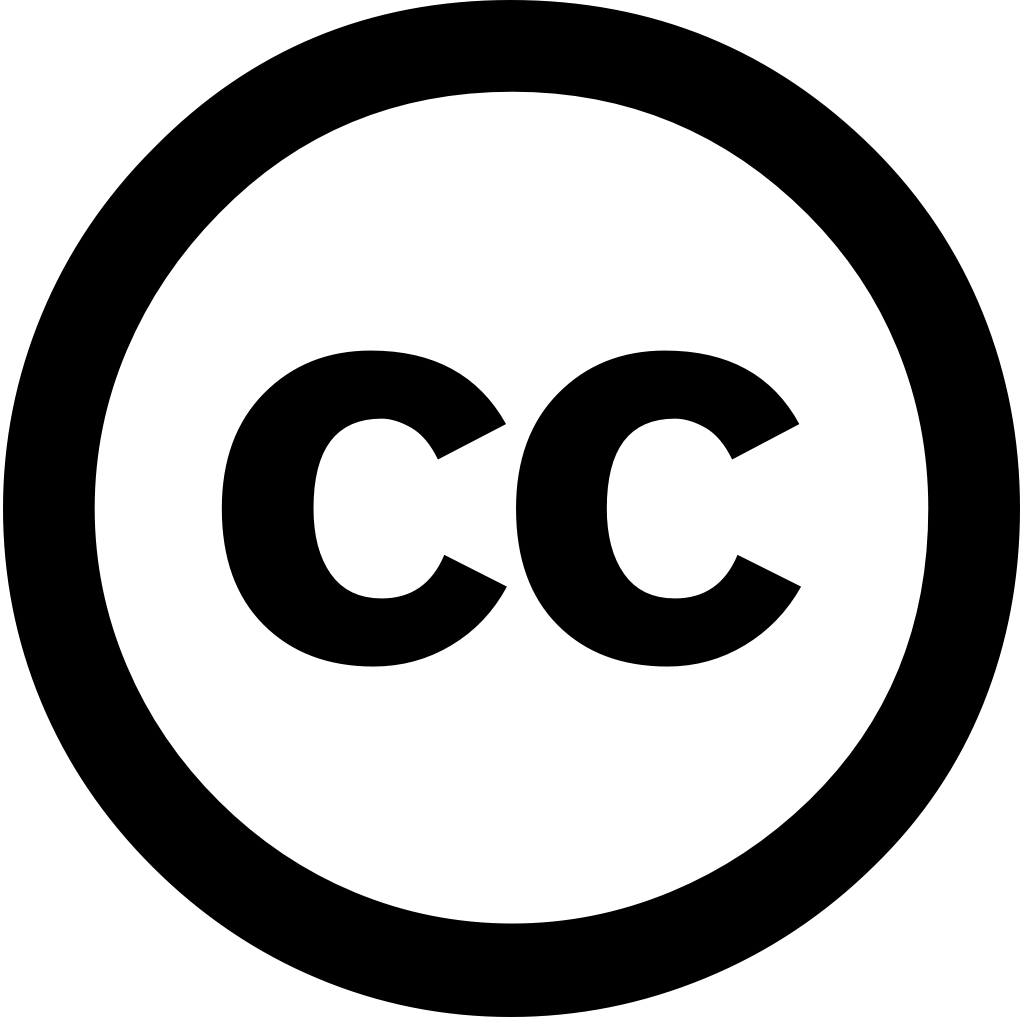
Frontiers in Environmental Science, Journal Year: 2025, Volume and Issue: 13
Published: May 13, 2025
Introduction The increasing frequency of slope disasters in urban and recreational public spaces, driven by climate change, presents significant risks to safety sustainable design. Conventional stability monitoring systems rely heavily on static models manual interventions, often lacking adaptability real-time predictive capacity. Earlier methods, including rule-based empirical approaches, use fixed thresholds assess risk factors such as soil moisture, angle, seismic activity. Although machine learning like decision trees support vector machines have improved predictions using historical data, their scalability remain constrained due the need for intensive feature engineering limited ability model complex nonlinear dynamics. Methods This study introduces a novel framework utilizing Deep Learning techniques enable intelligent, early warning disasters. Adaptive Spatial Design Model (ASDM) incorporates geospatial user behavior analytics, environmental sensing dynamically risk. It employs convolutional recurrent neural networks geo-hazard prediction, graph-theoretic optimization decision-making, adaptive spatial strategies enhance accuracy responsiveness changing environments. Results Experimental validation real-world datasets shows that proposed system effectively reduces false alarms improves response times 35% compared traditional methods. integration network-based prediction with planning enhances both precision timeliness disaster warnings. Discussion offers transformative, safe, functional approach management dynamic spaces. advances sustainability resilience optimizing design human-environment interactions. model's changes represents improvement mitigation strategies.
Language: Английский