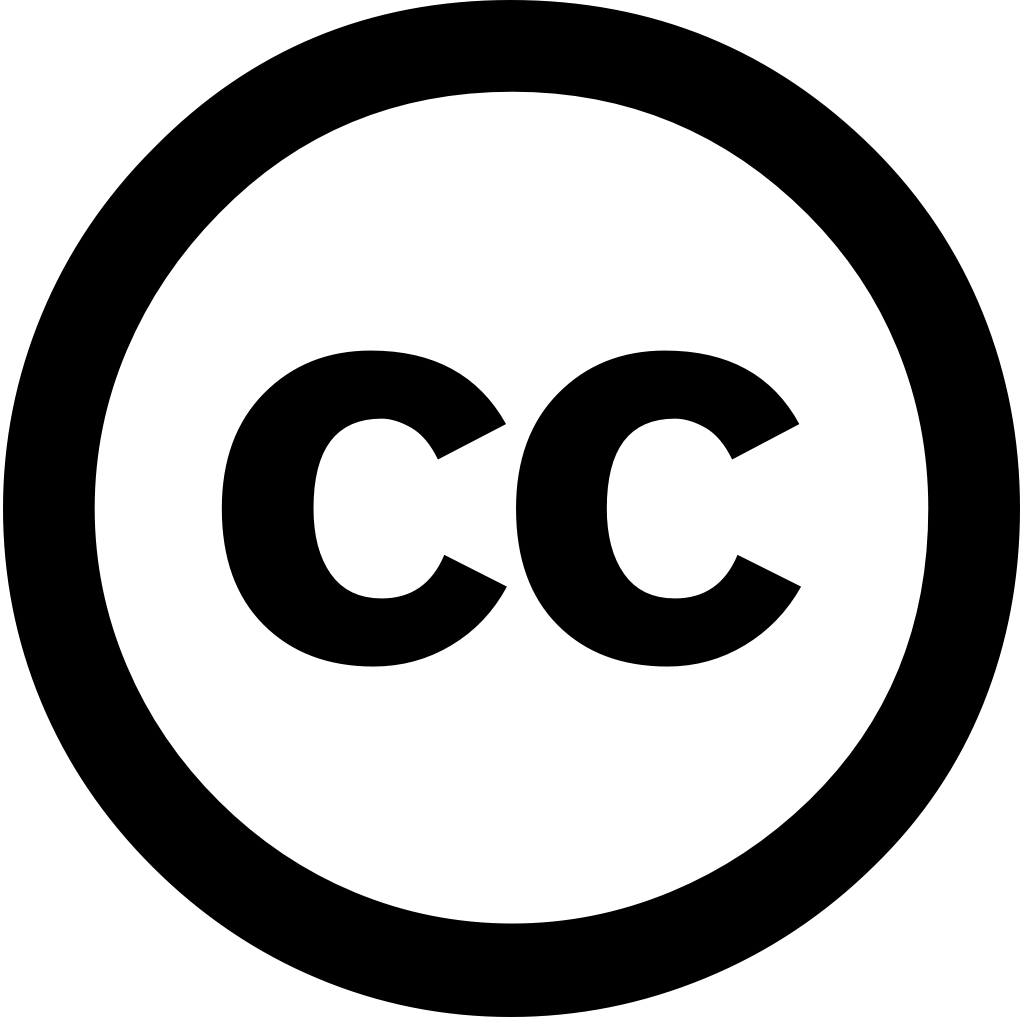
Electrochimica Acta, Journal Year: 2024, Volume and Issue: unknown, P. 145236 - 145236
Published: Oct. 1, 2024
Language: Английский
Electrochimica Acta, Journal Year: 2024, Volume and Issue: unknown, P. 145236 - 145236
Published: Oct. 1, 2024
Language: Английский
Sustainability, Journal Year: 2024, Volume and Issue: 16(21), P. 9555 - 9555
Published: Nov. 2, 2024
For decades, fossil fuels have been the backbone of reliable energy systems, offering unmatched density and flexibility. However, as world shifts toward renewable energy, overcoming limitations intermittent power sources requires a bold reimagining storage integration. Power-to-X (PtX) technologies, which convert excess electricity into storable carriers, offer promising solution for long-term sector coupling. Recent advancements in machine learning (ML) revolutionized PtX systems by enhancing efficiency, scalability, sustainability. This review provides detailed analysis how ML techniques, such deep reinforcement learning, data-driven optimization, predictive diagnostics, are driving innovation Power-to-Gas (PtG), Power-to-Liquid (PtL), Power-to-Heat (PtH) systems. example, has improved real-time decision-making PtG reducing operational costs improving grid stability. Additionally, diagnostics powered increased system reliability identifying early failures critical components proton exchange membrane fuel cells (PEMFCs). Despite these advancements, challenges data quality, processing, scalability remain, presenting future research opportunities. These to decarbonizing hard-to-electrify sectors, heavy industry, transportation, aviation, aligning with global sustainability goals.
Language: Английский
Citations
0Electrochimica Acta, Journal Year: 2024, Volume and Issue: unknown, P. 145236 - 145236
Published: Oct. 1, 2024
Language: Английский
Citations
0