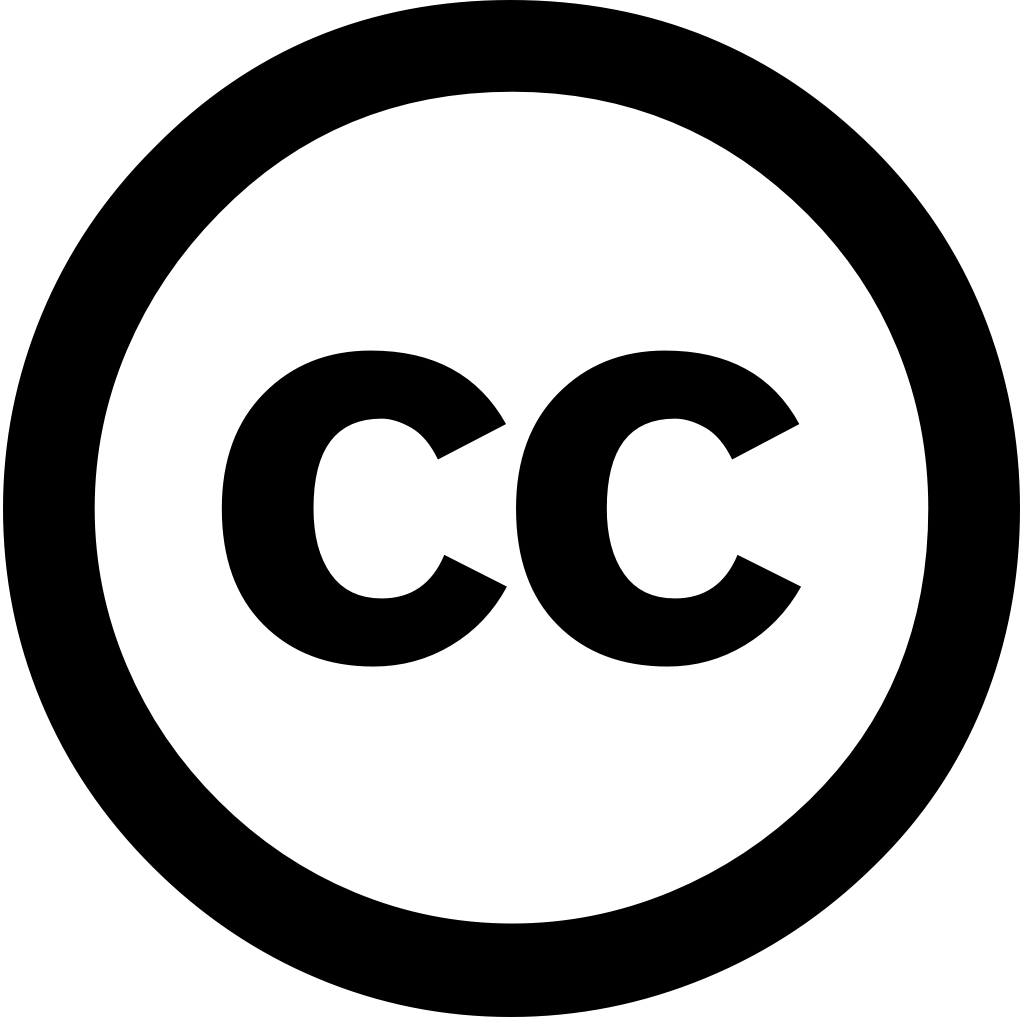
Fuel, Journal Year: 2024, Volume and Issue: 380, P. 133182 - 133182
Published: Sept. 21, 2024
Language: Английский
Fuel, Journal Year: 2024, Volume and Issue: 380, P. 133182 - 133182
Published: Sept. 21, 2024
Language: Английский
Chemical Engineering Journal, Journal Year: 2024, Volume and Issue: 485, P. 150059 - 150059
Published: Feb. 28, 2024
Language: Английский
Citations
25Results in Engineering, Journal Year: 2024, Volume and Issue: 21, P. 102002 - 102002
Published: March 1, 2024
The
efficient
design
of
heat
sinks
is
a
severe
challenge
in
thermo-fluid
engineering.
A
creative
and
innovative
way
applying
lateral
perforations
to
parallel
finned
sinks.
significance
achieving
an
optimal
for
perforated
(PFHSs)
has
inspired
the
present
authors
introduce
novel
hybrid
designing
approach
that
combines
computational
fluid
dynamics
(CFD),
machine
learning
(ML),
multi-objective
optimization
(MOO),
multi-criteria
decision-making
(MCDM).
variables
considered
include
size
(0.25<φ
<
0.5)
shape
(square,
circular,
hexagonal)
perforations,
as
well
airflow
Reynolds
number
(2000
Language: Английский
Citations
Tribology International,
Journal Year:
2023,
Volume and Issue:
187, P. 108759 - 108759
Published: July 5, 2023
Language: Английский
Citations
Results in Engineering,
Journal Year:
2024,
Volume and Issue:
24, P. 102858 - 102858
Published: Sept. 7, 2024
Language: Английский
Citations
International Communications in Heat and Mass Transfer,
Journal Year:
2024,
Volume and Issue:
155, P. 107535 - 107535
Published: May 7, 2024
Language: Английский
Citations
Fuel,
Journal Year:
2024,
Volume and Issue:
374, P. 132431 - 132431
Published: July 8, 2024
Language: Английский
Citations
Scientific Reports,
Journal Year:
2024,
Volume and Issue:
14(1) Published: Aug. 31, 2024
Abstract
Suspensions
containing
microencapsulated
phase
change
materials
(MPCMs)
play
a
crucial
role
in
thermal
energy
storage
(TES)
systems
and
have
applications
building
materials,
textiles,
cooling
systems.
This
study
focuses
on
accurately
predicting
the
dynamic
viscosity,
critical
thermophysical
property,
of
suspensions
MPCMs
MXene
particles
using
Gaussian
process
regression
(GPR).
Twelve
hyperparameters
(HPs)
GPR
are
analyzed
separately
classified
into
three
groups
based
their
importance.
Three
metaheuristic
algorithms,
namely
genetic
algorithm
(GA),
particle
swarm
optimization
(PSO),
marine
predators
(MPA),
employed
to
optimize
HPs.
Optimizing
four
most
significant
(covariance
function,
basis
standardization,
sigma)
within
first
group
any
algorithms
resulted
excellent
outcomes.
All
achieved
reasonable
R-value
(0.9983),
demonstrating
effectiveness
this
context.
The
second
explored
impact
including
additional,
moderate-significant
HPs,
such
as
fit
method,
predict
method
optimizer.
While
resulting
models
showed
some
improvement
over
group,
PSO-based
model
exhibited
noteworthy
enhancement,
achieving
higher
(0.99834).
Finally,
third
was
examine
potential
interactions
between
all
twelve
comprehensive
approach,
employing
GA,
yielded
an
optimized
with
highest
level
target
compliance,
reflected
by
impressive
0.999224.
developed
cost-effective
efficient
solution
reduce
laboratory
costs
for
various
systems,
from
TES
management.
Language: Английский
Citations
International Communications in Heat and Mass Transfer,
Journal Year:
2025,
Volume and Issue:
162, P. 108537 - 108537
Published: Jan. 5, 2025
Language: Английский
Citations
Energy,
Journal Year:
2024,
Volume and Issue:
293, P. 130606 - 130606
Published: Feb. 13, 2024
Language: Английский
Citations
Renewable Energy,
Journal Year:
2024,
Volume and Issue:
235, P. 121347 - 121347
Published: Sept. 10, 2024
Language: Английский
Citations
Using different machine learning algorithms to predict the rheological behavior of oil SAE40-based nano-lubricant in the presence of MWCNT and MgO nanoparticles
Enhancing Solar Energy Conversion Efficiency: Thermophysical Property Predicting of MXene/Graphene Hybrid Nanofluids via Bayesian-Optimized Artificial Neural Networks
A novel insight into the design of perforated-finned heat sinks based on a hybrid procedure: Computational fluid dynamics, machine learning, multi-objective optimization, and multi-criteria decision-making
Insights into water-lubricated transport of heavy and extra-heavy oils: Application of CFD, RSM, and metaheuristic optimized machine learning models
Optimizing Gaussian process regression (GPR) hyperparameters with three metaheuristic algorithms for viscosity prediction of suspensions containing microencapsulated PCMs
Harnessing meta-heuristic, Bayesian, and search-based techniques in optimizing machine learning models for improved energy storage with microencapsulated PCMs
Research and application of a Model selection forecasting system for wind speed and theoretical power generation in wind farms based on classification and wind conversion
Optimization and Prediction of Biogas Yield from Pretreated Ulva Intestinalis Linnaeus Applying Statistical-Based Regression Approach and Machine Learning Algorithms.