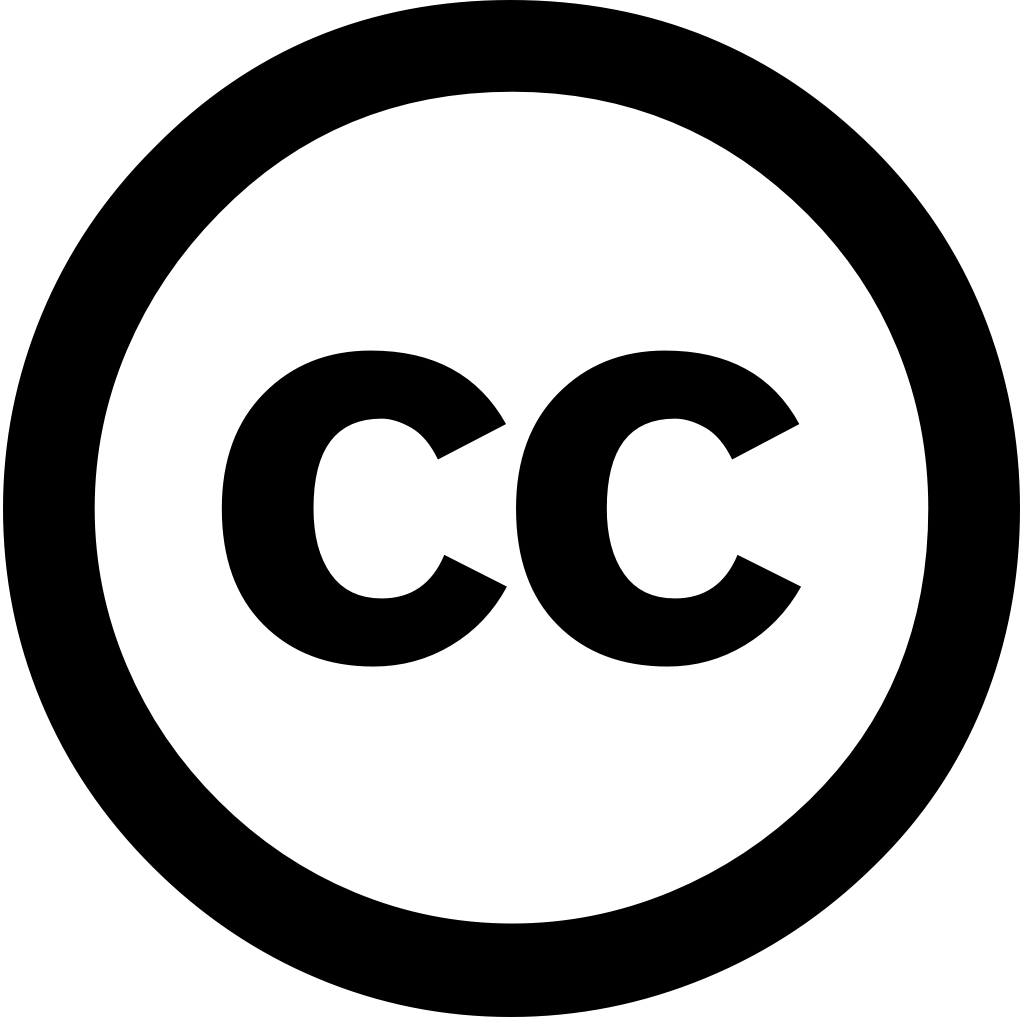
Computer Methods in Applied Mechanics and Engineering, Journal Year: 2025, Volume and Issue: 442, P. 117946 - 117946
Published: April 23, 2025
Language: Английский
Computer Methods in Applied Mechanics and Engineering, Journal Year: 2025, Volume and Issue: 442, P. 117946 - 117946
Published: April 23, 2025
Language: Английский
Computational methods in engineering & the sciences, Journal Year: 2023, Volume and Issue: unknown, P. 219 - 254
Published: Jan. 1, 2023
Language: Английский
Citations
52Annual Review of Fluid Mechanics, Journal Year: 2023, Volume and Issue: 56(1), P. 521 - 548
Published: Nov. 2, 2023
This review discusses Operator Inference, a nonintrusive reduced modeling approach that incorporates physical governing equations by defining structured polynomial form for the model, and then learns corresponding operators from simulated training data. The model of Inference is sufficiently expressive to cover wide range nonlinear dynamics found in fluid mechanics other fields science engineering, while still providing efficient computations. learning steps are rooted classical projection-based reduction; thus, some rich theory reduction can be applied models learned with Inference. connection offers pathway toward deriving error estimates gaining insights improve predictions. Furthermore, through formulations preserve Hamiltonian structures, important properties such as energy conservation guaranteed predictions beyond horizon. illustrates key computational large-scale combustion example.
Language: Английский
Citations
36Journal of Computational Physics, Journal Year: 2023, Volume and Issue: 492, P. 112420 - 112420
Published: Aug. 10, 2023
Language: Английский
Citations
27Computer Methods in Applied Mechanics and Engineering, Journal Year: 2025, Volume and Issue: 437, P. 117754 - 117754
Published: Jan. 30, 2025
Language: Английский
Citations
1AIAA SCITECH 2022 Forum, Journal Year: 2025, Volume and Issue: unknown
Published: Jan. 3, 2025
Language: Английский
Citations
1Journal of Computational and Applied Mathematics, Journal Year: 2025, Volume and Issue: unknown, P. 116584 - 116584
Published: Feb. 1, 2025
Language: Английский
Citations
1Journal of Computational Physics, Journal Year: 2025, Volume and Issue: unknown, P. 113957 - 113957
Published: March 1, 2025
Language: Английский
Citations
1Journal of Computational Physics, Journal Year: 2023, Volume and Issue: 491, P. 112356 - 112356
Published: July 13, 2023
Language: Английский
Citations
21Computer Methods in Applied Mechanics and Engineering, Journal Year: 2023, Volume and Issue: 417, P. 116402 - 116402
Published: Sept. 8, 2023
Language: Английский
Citations
18Chaos An Interdisciplinary Journal of Nonlinear Science, Journal Year: 2024, Volume and Issue: 34(3)
Published: March 1, 2024
We present a novel method for learning reduced-order models of dynamical systems using nonlinear manifolds. First, we learn the manifold by identifying structure in data through general representation problem. The proposed approach is driven embeddings low-order polynomial form. A projection onto reveals algebraic reduced-space system that governs problem interest. matrix operators model are then inferred from operator inference. Numerical experiments on number problems demonstrate generalizability methodology and increase accuracy can be obtained over modeling methods employ linear subspace approximation.
Language: Английский
Citations
8