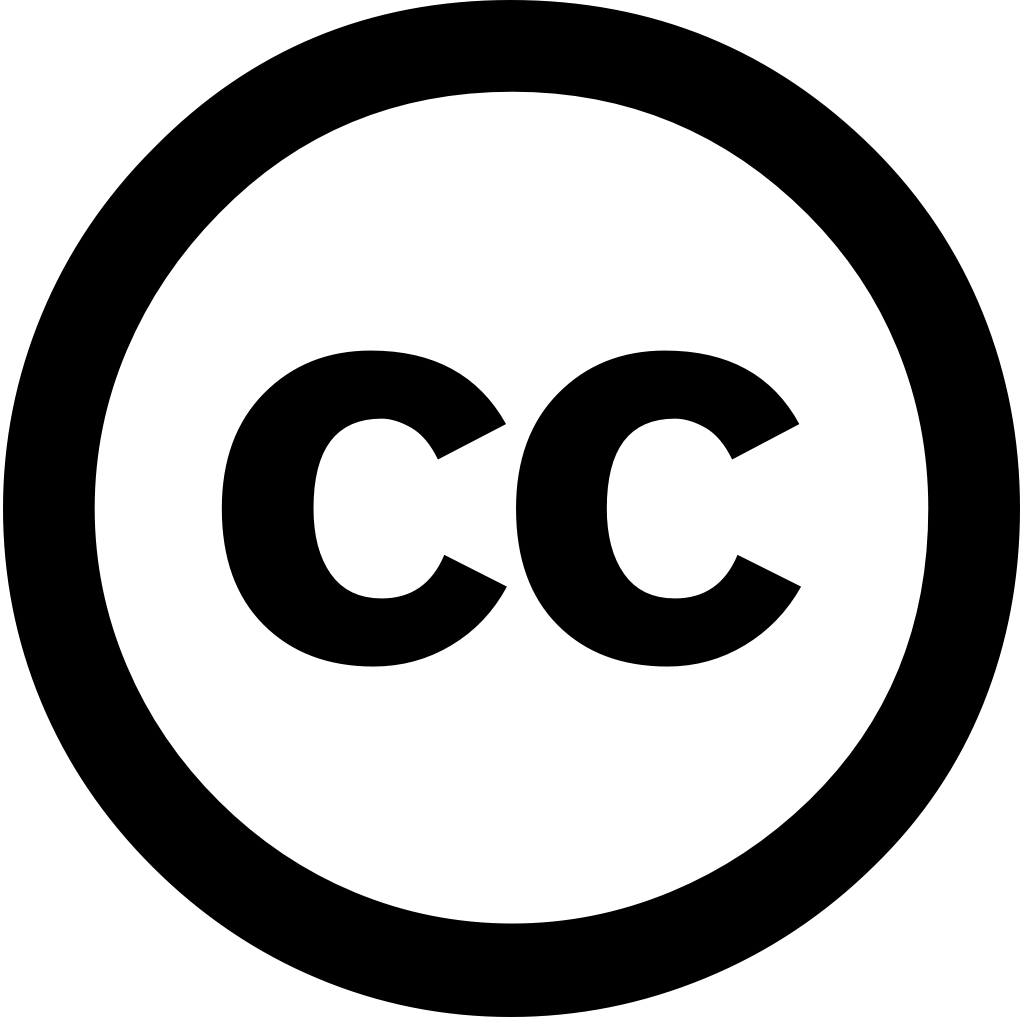
Computer Methods in Applied Mechanics and Engineering, Journal Year: 2025, Volume and Issue: 442, P. 117946 - 117946
Published: April 23, 2025
Language: Английский
Computer Methods in Applied Mechanics and Engineering, Journal Year: 2025, Volume and Issue: 442, P. 117946 - 117946
Published: April 23, 2025
Language: Английский
Computer Methods in Applied Mechanics and Engineering, Journal Year: 2024, Volume and Issue: 430, P. 117214 - 117214
Published: July 19, 2024
Language: Английский
Citations
5Computer Methods in Applied Mechanics and Engineering, Journal Year: 2023, Volume and Issue: 416, P. 116334 - 116334
Published: Aug. 28, 2023
Language: Английский
Citations
12Chaos An Interdisciplinary Journal of Nonlinear Science, Journal Year: 2023, Volume and Issue: 33(11)
Published: Nov. 1, 2023
Recently developed reduced-order modeling techniques aim to approximate nonlinear dynamical systems on low-dimensional manifolds learned from data. This is an effective approach for dynamics in a post-transient regime where the effects of initial conditions and other disturbances have decayed. However, transient near underlying manifold, as needed real-time control forecasting applications, complicated by fast nonnormal sensitivity mechanisms. To begin address these issues, we introduce parametric class projections described constrained autoencoder neural networks which both manifold projection fibers are Our architecture uses invertible activation functions biorthogonal weight matrices ensure that encoder left inverse decoder. We also new dynamics-aware cost promote learning oblique account nonnormality. demonstrate methods specific challenges they address, provide detailed case study three-state model vortex shedding wake bluff body immersed fluid, has two-dimensional slow can be computed analytically. In anticipation future applications high-dimensional systems, propose several constructing computationally efficient models using our proposed framework. includes novel sparsity-promoting penalty avoids detrimental matrix shrinkage via computation Grassmann manifold.
Language: Английский
Citations
12ESAIM. Mathematical modelling and numerical analysis, Journal Year: 2024, Volume and Issue: 59(1), P. 101 - 135
Published: June 21, 2024
This paper deals with the derivation of Non-Intrusive Reduced Basis (NIRB) techniques for sensitivity analysis, more specifically direct and adjoint state methods. For highly complex parametric problems, these two approaches may become too costly ans thus Methods (RBMs) be a viable option. We propose new NIRB two-grid algorithms both methods in context parabolic equations. The method uses HF code solely as “black-box”, requiring no modification. Like other RBMs, it is based on an offline-online decomposition. offline stage time-consuming, but only executed once, whereas online employs coarser grids thus, significantly less expensive than fine evaluation. On method, we prove classical model problem, heat equation, that evaluations sensitivities reach optimal convergence rate L ∞ (0, T ; H 1 0 (Ω)), then establish rates are recovered by approximation. These results supported numerical simulations. procedure further reduces computational costs step while computing coarse solution algorithm solutions. All run problem well namely Brusselator system.
Language: Английский
Citations
4SIAM Journal on Applied Dynamical Systems, Journal Year: 2024, Volume and Issue: 23(4), P. 3052 - 3076
Published: Dec. 11, 2024
Language: Английский
Citations
4Computer Physics Communications, Journal Year: 2025, Volume and Issue: 310, P. 109522 - 109522
Published: Jan. 31, 2025
Language: Английский
Citations
0Proceedings of the Royal Society A Mathematical Physical and Engineering Sciences, Journal Year: 2025, Volume and Issue: 481(2306)
Published: Jan. 1, 2025
The modelling and simulation of high-dimensional multiscale systems is a critical challenge across all areas science engineering. It broadly believed that even with today’s computer advances resolving spatio-temporal scales described by the governing equations remains remote target. This realization has prompted intense efforts to develop model-order reduction techniques. In recent years, techniques based on deep recurrent neural networks (RNNs) have produced promising results for complex spatiotemporal offer large flexibility in model development as they can incorporate experimental computational data. However, lack interpretability, which limits their utility generalizability systems. Here, we propose novel framework interpretable learning effective dynamics (iLED) offers comparable accuracy state-of-the-art RNN-based approaches while providing added benefit interpretability. iLED motivated Mori–Zwanzig Koopman operator (KO) theory, justifies choice specific architecture. We demonstrate effectiveness proposed simulations three benchmark Our show generate accurate predictions obtain dynamics, making it approach solving
Language: Английский
Citations
0Journal of Computational Physics, Journal Year: 2025, Volume and Issue: unknown, P. 113817 - 113817
Published: Feb. 1, 2025
Language: Английский
Citations
0Published: Jan. 1, 2025
Language: Английский
Citations
0Physica D Nonlinear Phenomena, Journal Year: 2025, Volume and Issue: unknown, P. 134572 - 134572
Published: Feb. 1, 2025
Language: Английский
Citations
0