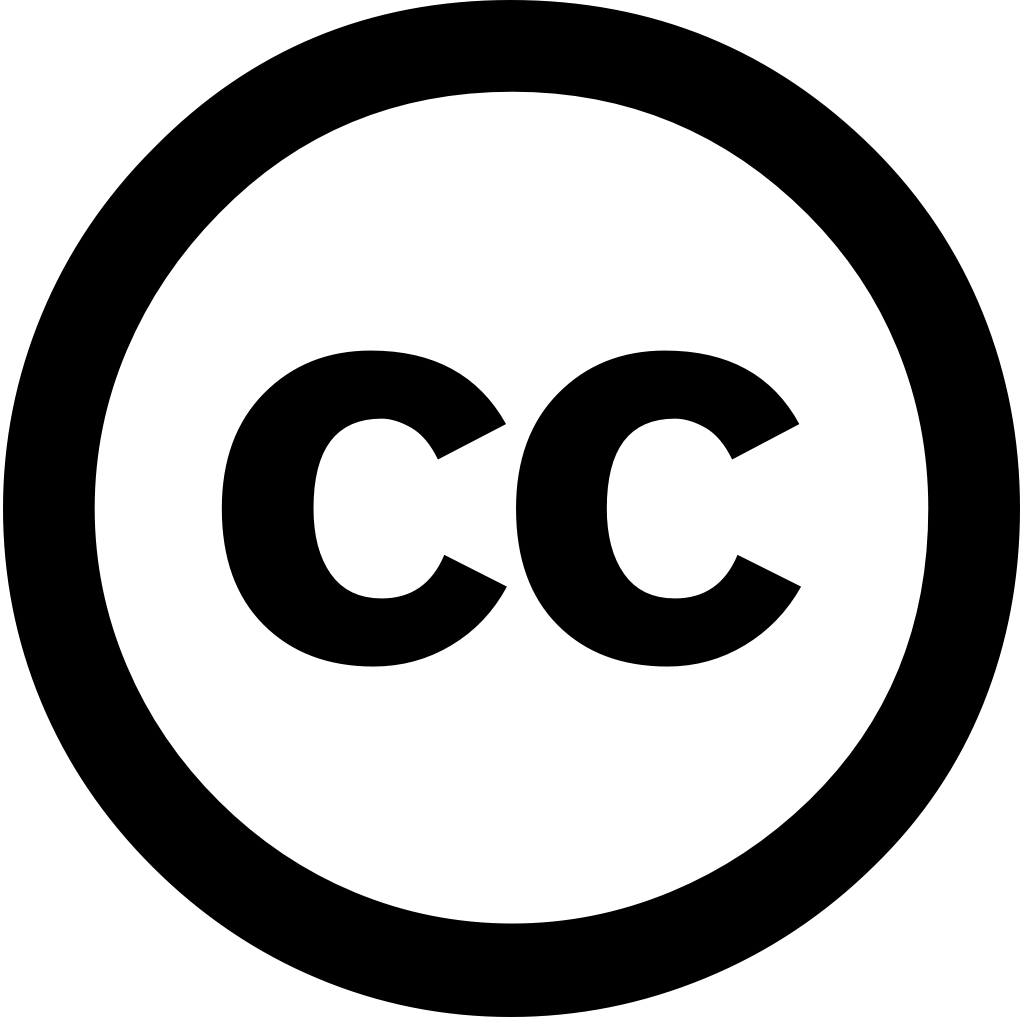
International Journal of Medical Informatics, Journal Year: 2024, Volume and Issue: 195, P. 105741 - 105741
Published: Dec. 2, 2024
Language: Английский
International Journal of Medical Informatics, Journal Year: 2024, Volume and Issue: 195, P. 105741 - 105741
Published: Dec. 2, 2024
Language: Английский
Archives of Computational Methods in Engineering, Journal Year: 2025, Volume and Issue: unknown
Published: March 1, 2025
Language: Английский
Citations
1Computer Methods and Programs in Biomedicine, Journal Year: 2025, Volume and Issue: unknown, P. 108702 - 108702
Published: March 1, 2025
Language: Английский
Citations
0Structural Control and Health Monitoring, Journal Year: 2025, Volume and Issue: 2025(1)
Published: Jan. 1, 2025
To ensure a safe environment for occupants, evaluating the physical status and service performance of existing buildings is essential. However, large‐scale building condition assessment usually relies on expertise judgment inspectors, which can be costly laborious due to unclear priorities, ambiguous procedures, ineffective operations. address these challenges, this study proposes an explainable machine learning‐based screening model anomalous safety among buildings, narrowing down scope requiring further detailed inspection monitoring. Initially, imbalanced dataset 18,090 survey reports unsafe labels collected. Then, synthetic minority oversampling technique (SMOTE) conducted balance dataset. Subsequently, seven learning models are trained utilizing 10‐fold cross‐validation with grid search. Findings reveal that, based balanced dataset, ensemble significantly better than that individual models. Specifically, XGBoost achieves highest performance, macro‐F1 98.49%, G‐mean value accuracy 98.49%. The final predictive (the SMOTE‐based model) explained using SHapley Additive exPlanations (SHAP). Service year, structure, location three most important features influencing structural safety. This represents promising approach automated optimizing resource allocation, enhancing effectiveness in decision‐making construction maintenance.
Language: Английский
Citations
0Journal of Translational Medicine, Journal Year: 2025, Volume and Issue: 23(1)
Published: April 2, 2025
The global burden of cardiovascular diseases continues to rise, making their prevention, diagnosis and treatment increasingly critical. With advancements breakthroughs in omics technologies such as high-throughput sequencing, multi-omics approaches can offer a closer reflection the complex physiological pathological changes body from molecular perspective, providing new microscopic insights into research. However, due vast volume complexity data, accurately describing, utilising, translating these biomedical data demands substantial effort. Researchers clinicians are actively developing artificial intelligence (AI) methods for data-driven knowledge discovery causal inference using various data. These AI approaches, integrated with research, have shown promising outcomes studies. In this review, we outline integrating machine learning, one most successful applications AI, summarise representative models developed that leverage facilitate exploration underlying mechanisms clinical practice. Particular emphasis is placed on effectiveness extract potential information address current gaps. We discuss challenges opportunities routine diagnostic therapeutic practices anticipate future development novel wider application field diseases.
Language: Английский
Citations
0Orthopaedic Surgery, Journal Year: 2024, Volume and Issue: 16(8), P. 2066 - 2080
Published: July 1, 2024
Objective Total hip arthroplasty (THA) remains the primary treatment option for femoral neck fractures in elderly patients. This study aims to explore risk factors associated with allogeneic blood transfusion after surgery and develop a dynamic prediction model predict post‐operative requirements. will provide more accurate guidance perioperative humoral management rational allocation of medical resources. Methods We retrospectively analyzed data from 829 patients who underwent total at three third‐class hospitals between January 2017 August 2023. Patient one hospital were used development, whereas other two external validation. Logistic regression analysis was screen characteristic subsets related transfusion. Various machine learning algorithms, including logistic regression, SVA (support vector machine), K‐NN (k‐nearest neighbors), MLP (multilayer perceptron), naive Bayes, decision tree, random forest, gradient boosting, process construct models. A 10‐fold cross‐validation algorithm facilitated comparison predictive performance models, resulting selection best‐performing development an open‐source computing program. Results BMI (body mass index), surgical duration, IBL (intraoperative loss), anticoagulant history, utilization rate tranexamic acid, Pre‐Hb, Pre‐ALB included as well independent factors. The average area under curve (AUC) values each follows: (0.98); (0.91); k‐NN (0.87) MLP, (0.96); Bayes (0.97); tree (0.87); forest boosting (0.97). web calculator based on best is available at: ( https://nomo99.shinyapps.io/dynnomapp/ ). Conclusion Utilizing computer algorithm, high discrimination accuracy (AUC > 0.5) developed. demonstrated superior differentiation reliability, thereby successfully passing model's strong generalizability applicability have significant implications clinicians, aiding identification postoperative
Language: Английский
Citations
0Heliyon, Journal Year: 2024, Volume and Issue: 10(20), P. e39609 - e39609
Published: Oct. 1, 2024
Language: Английский
Citations
0International Journal of Medical Informatics, Journal Year: 2024, Volume and Issue: 195, P. 105741 - 105741
Published: Dec. 2, 2024
Language: Английский
Citations
0