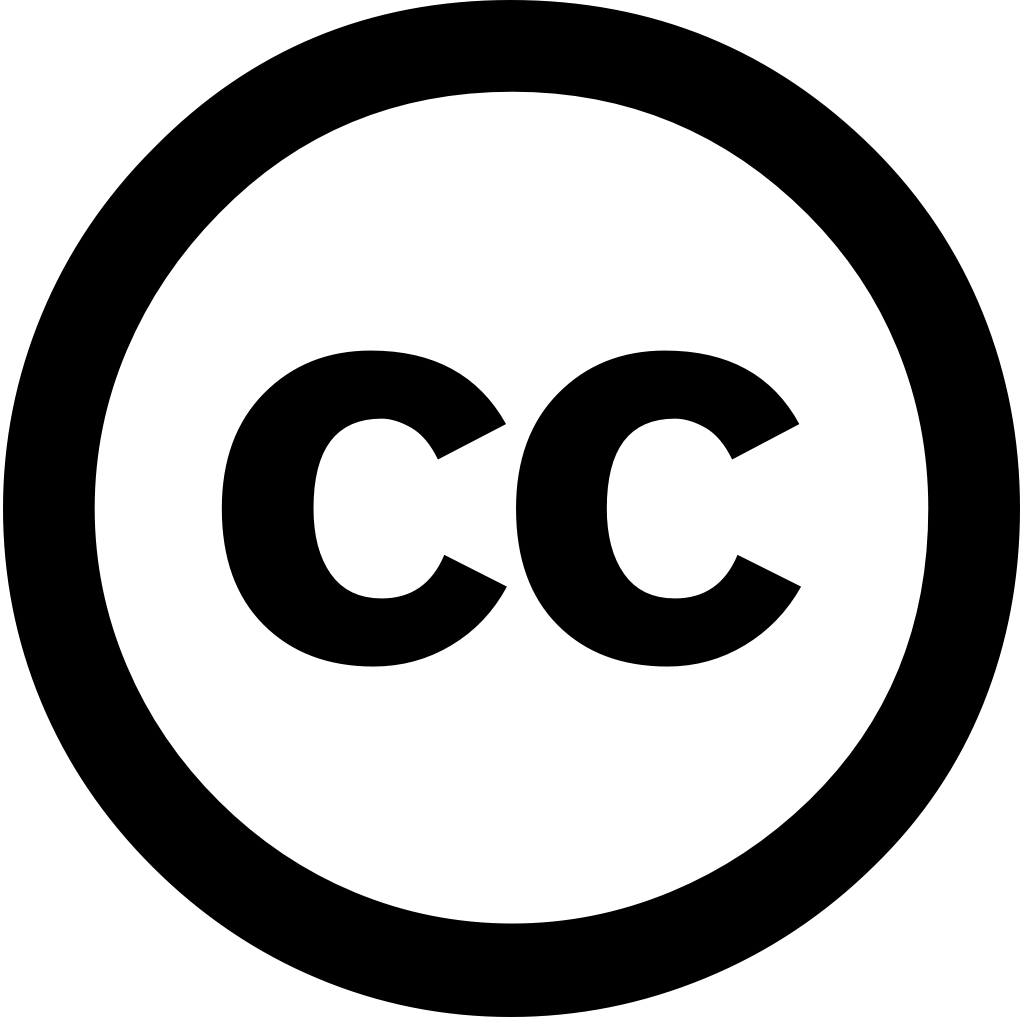
European Journal of Agronomy, Journal Year: 2025, Volume and Issue: 164, P. 127498 - 127498
Published: Jan. 15, 2025
Language: Английский
European Journal of Agronomy, Journal Year: 2025, Volume and Issue: 164, P. 127498 - 127498
Published: Jan. 15, 2025
Language: Английский
Environmental Modelling & Software, Journal Year: 2023, Volume and Issue: 167, P. 105776 - 105776
Published: July 5, 2023
Artificial Neural Networks (ANNs), sometimes also called models for deep learning, are used extensively the prediction of a range environmental variables. While potential ANNs is unquestioned, they surrounded by an air mystery and intrigue, leading to lack understanding their inner workings. This has led perpetuation number myths, resulting in misconception that applying primarily involves "throwing" large amount data at "black-box" software packages. this convenient way side-step principles applied development other types models, comes significant cost terms usefulness models. To address these issues, inroductory overview paper explodes common myths surrounding use outlines state-of-the-art approaches developing enable them be with confidence practice.
Language: Английский
Citations
55Information Fusion, Journal Year: 2024, Volume and Issue: 115, P. 102721 - 102721
Published: Oct. 9, 2024
Language: Английский
Citations
21Earth Science Informatics, Journal Year: 2024, Volume and Issue: 17(2), P. 1281 - 1299
Published: Jan. 10, 2024
Language: Английский
Citations
19Green Energy and Intelligent Transportation, Journal Year: 2025, Volume and Issue: unknown, P. 100260 - 100260
Published: Jan. 1, 2025
Language: Английский
Citations
3Trends in Food Science & Technology, Journal Year: 2023, Volume and Issue: 143, P. 104286 - 104286
Published: Dec. 4, 2023
Language: Английский
Citations
35Agriculture, Journal Year: 2024, Volume and Issue: 14(6), P. 794 - 794
Published: May 22, 2024
The accurate prediction of crop yields is crucial for enhancing agricultural efficiency and ensuring food security. This study assesses the performance CNN-LSTM-Attention model in predicting maize, rice, soybeans Northeast China compares its effectiveness with traditional models such as RF, XGBoost, CNN. Utilizing multi-source data from 2014 to 2020, which include vegetation indices, environmental variables, photosynthetically active parameters, our research examines model’s capacity capture essential spatial temporal variations. integrates Convolutional Neural Networks, Long Short-Term Memory, an attention mechanism effectively process complex datasets manage non-linear relationships within data. Notably, explores potential using kNDVI multiple crops, highlighting effectiveness. Our findings demonstrate that advanced deep-learning significantly enhance yield accuracy over methods. We advocate incorporation sophisticated technologies practices, can substantially improve production strategies.
Language: Английский
Citations
15Neural Computing and Applications, Journal Year: 2024, Volume and Issue: 36(33), P. 20723 - 20750
Published: Aug. 16, 2024
Abstract Accurately predicting crop yield is essential for optimizing agricultural practices and ensuring food security. However, existing approaches often struggle to capture the complex interactions between various environmental factors growth, leading suboptimal predictions. Consequently, identifying most important feature vital when leveraging Support Vector Regressor (SVR) prediction. In addition, manual tuning of SVR hyperparameters may not always offer high accuracy. this paper, we introduce a novel framework yields that address these challenges. Our integrates new hybrid selection approach with an optimized model enhance prediction accuracy efficiently. The proposed comprises three phases: preprocessing, selection, phases. preprocessing phase, data normalization conducted, followed by application K-means clustering in conjunction correlation-based filter (CFS) generate reduced dataset. Subsequently, FMIG-RFE proposed. Finally, phase introduces improved variant Crayfish Optimization Algorithm (COA), named ICOA, which utilized optimize thereby achieving superior along approach. Several experiments are conducted assess evaluate performance framework. results demonstrated over state-of-art approaches. Furthermore, experimental findings regarding ICOA optimization algorithm affirm its efficacy model, enhancing both computational efficiency, surpassing algorithms.
Language: Английский
Citations
15Protection and Control of Modern Power Systems, Journal Year: 2024, Volume and Issue: 9(6), P. 1 - 18
Published: Nov. 1, 2024
Supercapacitors (SCs) are widely recognized as excellent clean energy storage devices. Accurate state of health (SOH) estimation and remaining useful life (RUL) prediction essential for ensuring their safe reliable operation. This paper introduces a novel method SOH RUL prediction, based on hybrid neural network optimized by an improved honey badger algorithm (HBA). The combines the advantages convolutional (CNN) bidirectional long-short-term memory (BiLSTM) network. HBA optimizes hyperparameters CNN automatically extracts deep features from time series data reduces dimensionality, which then used input BiLSTM. Additionally, recurrent dropout is introduced in layer to reduce overfitting facilitate learning process. approach not only improves accuracy estimates forecasts but also significantly processing time. SCs under different working conditions validate proposed method. results show that model effectively features, enriches local details, enhances global perception capabilities. outperforms single models, reducing root mean square error below 1%, offers higher robustness compared other methods.
Language: Английский
Citations
15Neural Computing and Applications, Journal Year: 2024, Volume and Issue: unknown
Published: Nov. 18, 2024
Language: Английский
Citations
11Precision Agriculture, Journal Year: 2024, Volume and Issue: 25(3), P. 1127 - 1180
Published: Feb. 27, 2024
Abstract The estimation of pre-harvest fruit quality and maturity is essential for growers to determine the harvest timing, storage requirements profitability crop yield. In-field indicators are highly variable require high spatiotemporal resolution data, which can be obtained from contemporary precision agriculture systems. Such systems exploit various state-of-the-art sensors, increasingly relying on spectrometry imaging techniques in association with advanced Artificial Intelligence (AI) and, particular, Machine Learning (ML) algorithms. This article presents a critical review estimation, focus destructive non-destructive measurement approaches, applications ML domain. A analysis advantages disadvantages different conducted by surveying recent articles methods discern trends performance applicability. Advanced data-fusion combining information multiple sensors being used develop more accurate representations entire field. achieved incorporating AI algorithms, such as support vector machines, k-nearest neighbour, neural networks, clustering. Based an extensive survey recently published research, also identifies most effective indices, namely: sugar content, acidity firmness. concludes highlighting outstanding technical challenges promising areas future research. Hence, this research has potential provide valuable resource growers, allowing them familiarize themselves Smart Agricultural methodologies currently use. These practices gradually incorporated their perspective, taking into account availability use efficient indices.
Language: Английский
Citations
10