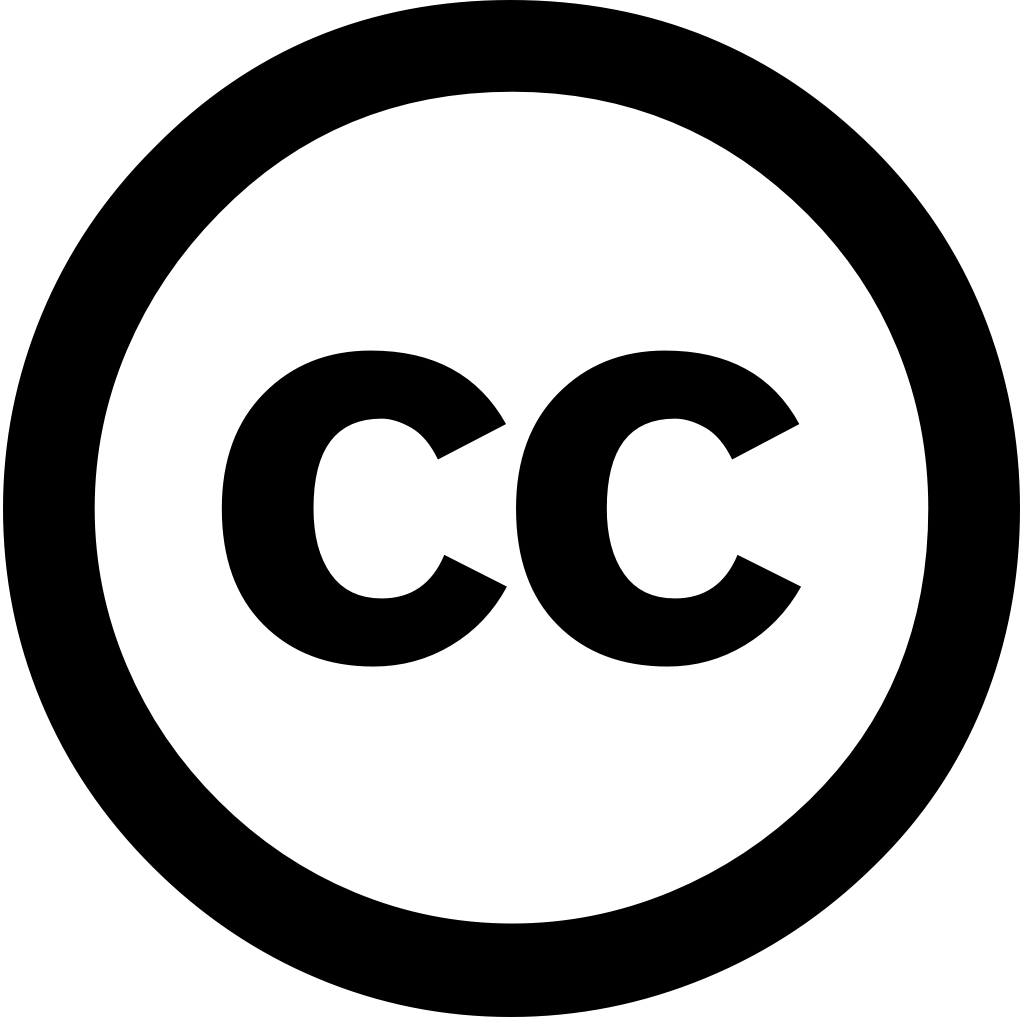
Information Processing in Agriculture, Journal Year: 2025, Volume and Issue: unknown
Published: Feb. 1, 2025
Language: Английский
Information Processing in Agriculture, Journal Year: 2025, Volume and Issue: unknown
Published: Feb. 1, 2025
Language: Английский
Symmetry, Journal Year: 2025, Volume and Issue: 17(2), P. 255 - 255
Published: Feb. 8, 2025
To address the issues of low detection accuracy and poor adaptability in complex orchard environments (such as varying lighting conditions, branch leaf occlusion, fruit overlap, small targets), this paper proposes an improved pear model based on YOLO11, called YOLO11-Pear. First, to improve model’s capability detecting occluded pears, C2PSS module is introduced replace original C2PSA module. Second, a target layer added ability detect pears. Finally, upsampling process replaced with DySample, which not only maintains high efficiency but also improves processing speed expands application range. validate effectiveness model, dataset images Qiu Yue pears Cui Guan was constructed. The experimental results showed that YOLO11-Pear achieved precision, recall, mAP50, mAP50–95 values 96.3%, 84.2%, 92.1%, 80.2%, respectively, outperforming YOLO11n by 3.6%, 1%, 2.1%, 3.2%. With 2.4% increase number parameters compared enables fast accurate environments.
Language: Английский
Citations
5Journal of the Taiwan Institute of Chemical Engineers, Journal Year: 2025, Volume and Issue: 170, P. 106032 - 106032
Published: Feb. 27, 2025
Language: Английский
Citations
4Agriculture, Journal Year: 2025, Volume and Issue: 15(7), P. 765 - 765
Published: April 2, 2025
Accurate apple yield estimation is essential for effective orchard management, market planning, and ensuring growers’ income. However, complex conditions, such as dense foliage occlusion overlapping fruits, present challenges to large-scale estimation. This study introduces APYOLO, an enhanced detection algorithm based on improved YOLOv11, integrated with the DeepSORT tracking improve both accuracy operational speed. APYOLO incorporates a multi-scale channel attention (MSCA) mechanism prior distribution intersection over union (EnMPDIoU) loss function enhance target localization recognition under environments. Experimental results demonstrate that outperforms original YOLOv11 by improving [email protected], [email protected]–0.95, accuracy, recall 2.2%, 2.1%, 0.8%, 2.3%, respectively. Additionally, combination of unique ID region line (ROL) strategy in further boosts 84.45%, surpassing performance method alone. provides more precise efficient system estimation, offering strong technical support intelligent refined management.
Language: Английский
Citations
2Published: June 20, 2024
This review systematically examines the progression of You Only Look Once (YOLO) object detection algorithms from YOLOv1 to recently unveiled YOLOv10. Employing a reverse chronological analysis, this study advancements introduced by YOLO algorithms, beginning with YOLOv10 and progressing through YOLOv9, YOLOv8, subsequent versions explore each version's contributions enhancing speed, accuracy, computational efficiency in real-time detection. The highlights transformative impact across five critical application areas: automotive safety, healthcare, industrial manufacturing, surveillance, agriculture. By detailing incremental technological that iteration brought, not only chronicles evolution but also discusses challenges limitations observed earlier versions. signifies path towards integrating multimodal, context-aware, General Artificial Intelligence (AGI) systems for next decade, promising significant implications future developments AI-driven applications.
Language: Английский
Citations
13Scientific Reports, Journal Year: 2024, Volume and Issue: 14(1)
Published: July 22, 2024
Pomegranate is an important fruit crop that usually managed manually through experience. Intelligent management systems for pomegranate orchards can improve yields and address labor shortages. Fast accurate detection of pomegranates one the key technologies this system, crucial yield scientific management. Currently, most solutions use deep learning to achieve detection, but not effective in detecting small targets large parameters, computation speed slow; therefore, there room improving task. Based on improved You Only Look Once version 5 (YOLOv5) algorithm, a lightweight growth period algorithm YOLO-Granada proposed. A ShuffleNetv2 network used as backbone extract features. Using grouped convolution reduces computational effort ordinary convolution, using channel shuffle increases interaction between different channels. In addition, attention mechanism help neural suppress less significant features channels or space, Convolutional Block Attention Module effect optimize object accuracy by contribution factor weights. The average reaches 0.922. It only than 1% lower original YOLOv5s model (0.929) brings increase compression size. 17.3% faster network. floating-point operations, size are compressed 54.7%, 51.3%, 56.3% network, respectively. detects 8.66 images per second, achieving real-time results. study, Nihui convolutional framework was further utilized develop Android-based application detection. method provides more solution intelligent devices orchards, which provide reference design networks agricultural applications.
Language: Английский
Citations
13Inorganic Chemistry Communications, Journal Year: 2024, Volume and Issue: 170, P. 113422 - 113422
Published: Oct. 31, 2024
Language: Английский
Citations
9Automation in Construction, Journal Year: 2025, Volume and Issue: 171, P. 105970 - 105970
Published: Jan. 27, 2025
Language: Английский
Citations
1Journal of Agriculture and Food Research, Journal Year: 2025, Volume and Issue: unknown, P. 101734 - 101734
Published: Feb. 1, 2025
Language: Английский
Citations
1Solar, Journal Year: 2025, Volume and Issue: 5(1), P. 6 - 6
Published: Feb. 21, 2025
The reliable operation of photovoltaic (PV) systems is essential for sustainable energy production, yet their efficiency often compromised by defects such as bird droppings, cracks, and dust accumulation. Automated defect detection critical addressing these challenges in large-scale solar farms, where manual inspections are impractical. This study evaluates three YOLO object models—YOLOv5, YOLOv8, YOLOv11—on a comprehensive dataset to identify panel defects. YOLOv5 achieved the fastest inference time (7.1 ms per image) high precision (94.1%) cracked panels. YOLOv8 excelled recall rare defects, drops (79.2%), while YOLOv11 delivered highest [email protected] (93.4%), demonstrating balanced performance across categories. Despite strong common like dusty panels ([email protected] > 98%), drop posed due imbalances. These results highlight trade-offs between accuracy computational efficiency, providing actionable insights deploying automated enhance PV system reliability scalability.
Language: Английский
Citations
1Sensors, Journal Year: 2025, Volume and Issue: 25(5), P. 1502 - 1502
Published: Feb. 28, 2025
Effective fruit identification and maturity detection are important for harvesting managing tomatoes. Current deep learning algorithms typically demand significant computational resources memory. Detecting severely stacked obscured tomatoes in unstructured natural environments is challenging because of target stacking, occlusion, illumination, background noise. The proposed method involves a new lightweight model called GPC-YOLO based on YOLOv8n tomato detection. This study proposes C2f-PC module partial convolution (PConv) less computation, which replaced the original C2f feature extraction YOLOv8n. regular was with Grouped Spatial Convolution (GSConv) by downsampling to reduce burden. neck network convolutional neural network-based cross-scale fusion (CCFF) enhance adaptability scale changes detect many small-scaled objects. Additionally, integration simple attention mechanism (SimAM) efficient intersection over union (EIoU) loss were implemented further accuracy leveraging these improvements. trained validated dataset 1249 mobile phone images Compared YOLOv8n, achieved high-performance metrics, e.g., reducing parameter number 1.2 M (by 59.9%), compressing size 2.7 57.1%), decreasing floating point operations 4.5 G 45.1%), improving 98.7% 0.3%), speed 201 FPS. showed that could effectively identify environments. has immense potential ripeness automated picking applications.
Language: Английский
Citations
1