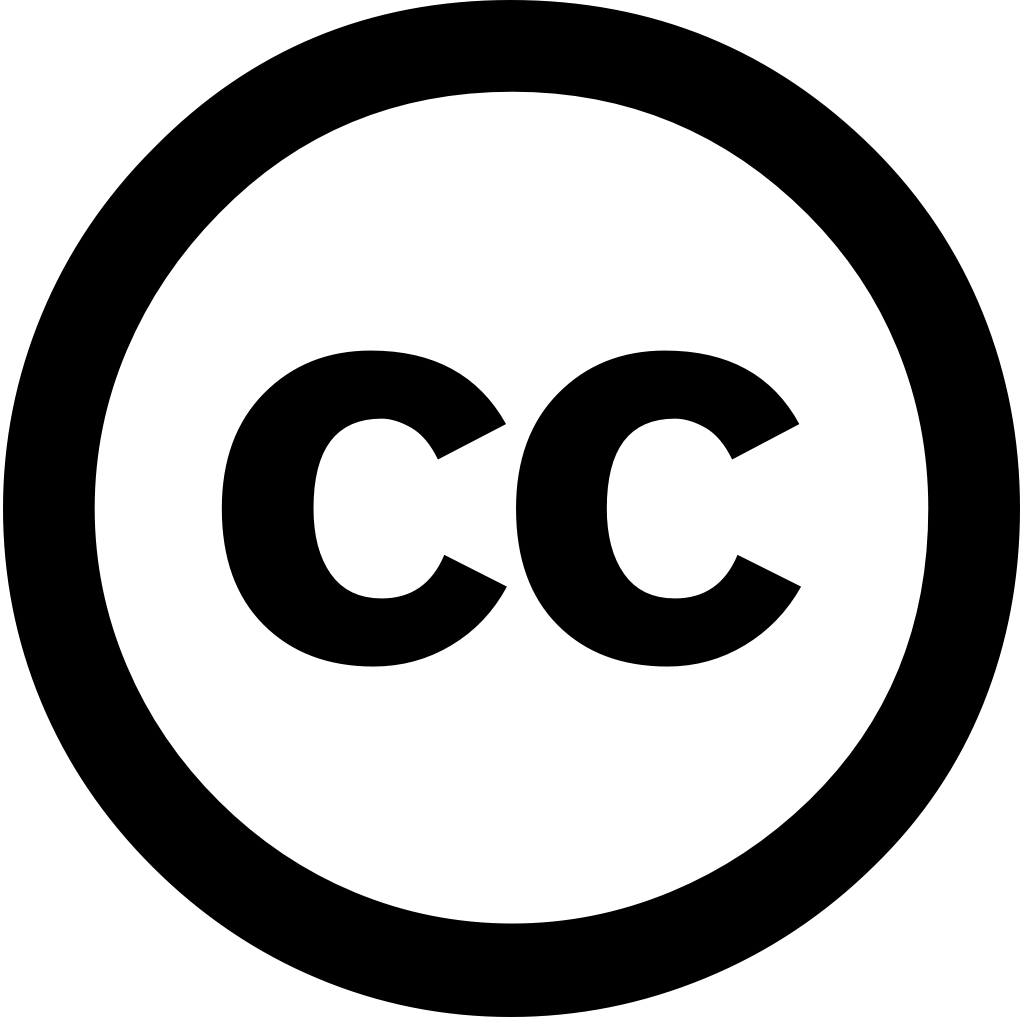
Smart Agricultural Technology, Journal Year: 2024, Volume and Issue: unknown, P. 100654 - 100654
Published: Nov. 1, 2024
Language: Английский
Smart Agricultural Technology, Journal Year: 2024, Volume and Issue: unknown, P. 100654 - 100654
Published: Nov. 1, 2024
Language: Английский
Computers and Electronics in Agriculture, Journal Year: 2025, Volume and Issue: 232, P. 110057 - 110057
Published: Feb. 7, 2025
Language: Английский
Citations
2Computers and Electronics in Agriculture, Journal Year: 2025, Volume and Issue: 230, P. 109889 - 109889
Published: Jan. 2, 2025
Language: Английский
Citations
1Social Responsibility Journal, Journal Year: 2025, Volume and Issue: 21(4), P. 725 - 750
Published: Jan. 2, 2025
Purpose This study addresses the growing challenges of food security, livelihood generation and sustainable urban living in context increasing urbanization developing countries. As populations are projected to rise significantly coming decades, agriculture emerges as a potential solution support dietary needs economic empowerment. However, farming practices countries face unique socio-economic, cultural technological compared developed nations. paper aims analyze global practices, examine success stories from propose an adaptable, inclusive model tailored Design/methodology/approach The research systematically reviews emphasizing successful implementations highlights gaps areas opportunity by comparing these with socio-economic contexts Focus group interviews were conducted among farmers India finalize key variables second phase involves construction context-specific for proposing interventions. A comparative method is devised identify country’s agricultural policies derive optimal Findings findings reveal that countries, motivated urge become self-sustainable maintain connection community shaped different environmental factors. In prime motive generate secondary income source ensure security. Still, they limited access, insufficient policy socio-cultural barriers. Technology-supported infrastructure government other stakeholders would be implement integrative solutions. To this, adaptive required bridge gap current system. Practical implications offers practical policymakers, planners It emphasizes importance designing address resource constraints, such land availability financial access promote techniques. recommends creating supportive frameworks empower farmers, including subsidies, training programs efficient market mechanisms. Integrating into city planning can foster green spaces, enhance security drive development. These actionable recommendations aim facilitate growth practice Social Participation social cohesiveness aspects provide beneficial accessibility concept all, ensuring equality accessing quality food. role addressing inequalities, particularly By engaging communities create collaborative networks, healthier lifestyles improve fresh produce. also underscores empowering through targeted interventions farming. reinforce value enhancing overall life. Originality/value makes contribution focusing on contextual adaptation models While has been extensively studied nations, regions’ specific opportunities. comprehensive bridges gaps, this provides novel framework integrating landscape originality lies its approach, leveraging best while tailoring solutions local contexts, thereby advancing discourse agriculture.
Language: Английский
Citations
0Food systems, Journal Year: 2025, Volume and Issue: 7(4), P. 543 - 550
Published: Feb. 1, 2025
One of the frontiers science is development a digital twin for food product to predict composition and properties future product. Today, however, computer simulation (modeling) used predicting The aim our research compare levels nutritional value parameters from model real assess adequacy obtained data. objects were emulsified meat-and-plant based on traditional meal “Mukhamar” (computer simulator) recipe meat-andplant By example “Mukhamar”, stages are shown. It was demonstrated that it incorrect use without supporting with data (numerical values) apparatus, sensors. calculated compared empirically (as result laboratory experiment) in three blocks: physicochemical indicators, vitamins minerals. Simulation calculation absolute relative errors performed program environment R Studio. Differences between empirical can be explained, firstly, by average values databases. As now, databases contain averaged data, which do not take into account individual characteristics animal plant raw materials. Secondly, necessary consideration coefficient losses (or preservation) nutrients during thermal treatment food. has been established only precise regard all will help trace quality at each stage production, allow reacting timely deviations improving final
Language: Английский
Citations
0Computers and Electronics in Agriculture, Journal Year: 2025, Volume and Issue: 231, P. 110001 - 110001
Published: Feb. 13, 2025
Language: Английский
Citations
0Smart Agricultural Technology, Journal Year: 2025, Volume and Issue: unknown, P. 100830 - 100830
Published: Feb. 1, 2025
Language: Английский
Citations
0Computers and Electronics in Agriculture, Journal Year: 2025, Volume and Issue: 232, P. 110127 - 110127
Published: Feb. 16, 2025
Language: Английский
Citations
0Agronomy, Journal Year: 2025, Volume and Issue: 15(4), P. 791 - 791
Published: March 24, 2025
Accurate soybean pod counting remains a significant challenge in field-based phenotyping due to complex factors such as occlusion, dense distributions, and background interference. We present SmartPod, an advanced deep learning framework that addresses these challenges through three key innovations: (1) novel vision Transformer architecture for enhanced feature representation, (2) efficient attention mechanism the improved detection of overlapping pods, (3) semi-supervised strategy maximizes performance with limited annotated data. Extensive evaluations demonstrate SmartPod achieves state-of-the-art Average Precision at IoU threshold 0.5 (AP@IoU = 0.5) 94.1%, outperforming existing methods by 1.7–4.6% across various field conditions. This improvement, combined framework’s robustness environments, positions transformative tool large-scale precision breeding applications.
Language: Английский
Citations
0Computers and Electronics in Agriculture, Journal Year: 2025, Volume and Issue: 234, P. 110225 - 110225
Published: March 27, 2025
Language: Английский
Citations
0Frontiers in Plant Science, Journal Year: 2025, Volume and Issue: 16
Published: March 27, 2025
High-quality 3D reconstruction and accurate organ segmentation of plants are crucial prerequisites for automatically extracting phenotypic traits. In this study, we first extract a dense point cloud from implicit representations, which derives reconstructing the maize in by using Nerfacto neural radiance field model. Second, propose lightweight network (PointSegNet) specifically stem leaf segmentation. This includes Global-Local Set Abstraction (GLSA) module to integrate local global features an Edge-Aware Feature Propagation (EAFP) enhance edge-awareness. Experimental results show that our PointSegNet achieves impressive performance compared five other state-of-the-art deep learning networks, reaching 93.73%, 97.25%, 96.21%, 96.73% terms mean Intersection over Union (mIoU), precision, recall, F1-score, respectively. Even when dealing with tomato soybean plants, complex structures, also best metrics. Meanwhile, based on principal component analysis (PCA), further optimize method obtain parameters such as length width PCA vectors. Finally, thickness, height, length, obtained measurements manual test results, yielding R 2 values 0.99, 0.84, 0.94, 0.87, These indicate has high accuracy reliability parameter extraction. study throughout entire process extraction, provides reliable objective acquiring plant will boost development smart agriculture.
Language: Английский
Citations
0