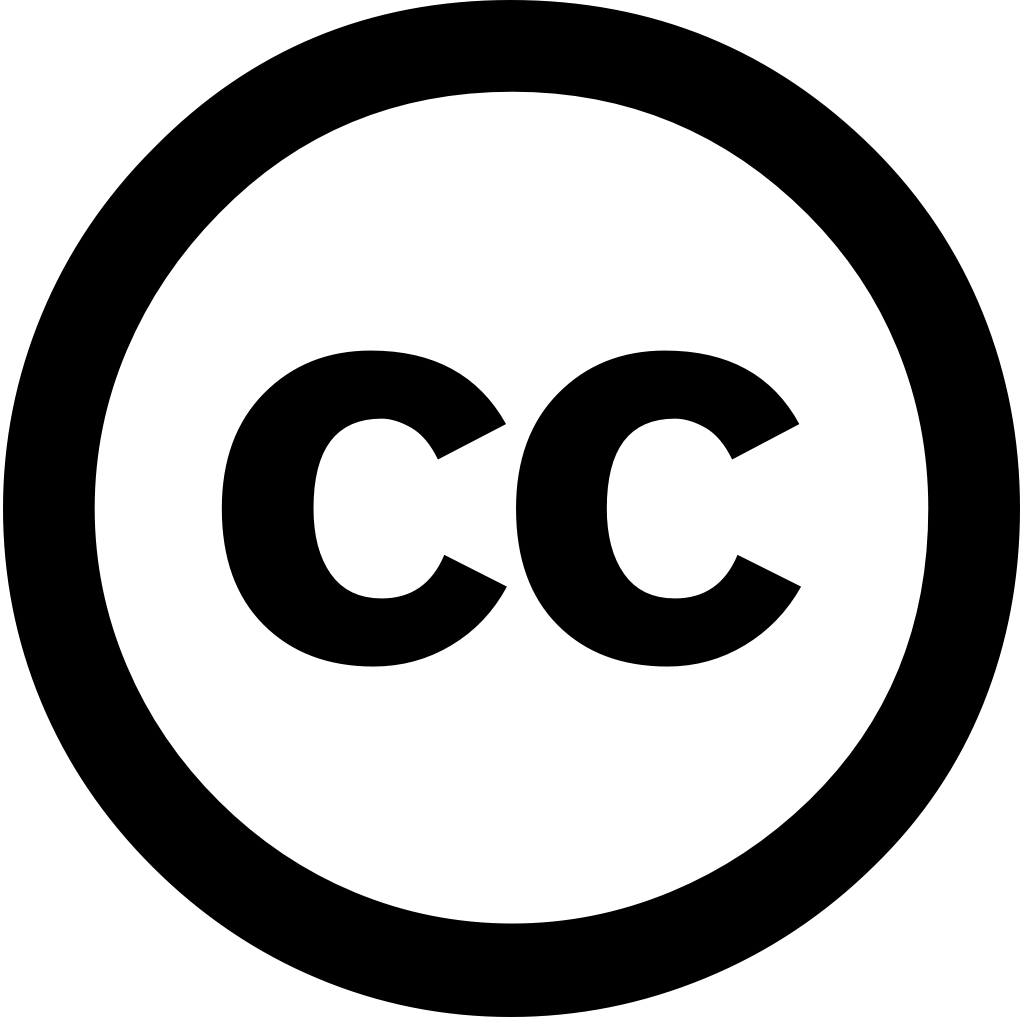
Scientific Reports, Journal Year: 2024, Volume and Issue: 14(1)
Published: Dec. 28, 2024
Language: Английский
Scientific Reports, Journal Year: 2024, Volume and Issue: 14(1)
Published: Dec. 28, 2024
Language: Английский
SoftwareX, Journal Year: 2025, Volume and Issue: 30, P. 102083 - 102083
Published: Feb. 11, 2025
Language: Английский
Citations
2Smart Agricultural Technology, Journal Year: 2025, Volume and Issue: unknown, P. 100878 - 100878
Published: March 1, 2025
Language: Английский
Citations
1Earth Science Informatics, Journal Year: 2025, Volume and Issue: 18(2)
Published: Jan. 16, 2025
Language: Английский
Citations
0Sustainability, Journal Year: 2025, Volume and Issue: 17(5), P. 2250 - 2250
Published: March 5, 2025
Hydrology relates to many complex challenges due climate variability, limited resources, and especially, increased demands on sustainable management of water soil. Conventional approaches often cannot respond the integrated complexity continuous change inherent in system; hence, researchers have explored advanced data-driven solutions. This review paper revisits how artificial intelligence (AI) is dramatically changing most important facets hydrological research, including soil land surface modeling, streamflow, groundwater forecasting, quality assessment, remote sensing applications resources. In AI techniques could further enhance accuracy texture analysis, moisture estimation, erosion prediction for better management. Advanced models also be used as a tool forecast streamflow levels, therefore providing valuable lead times flood preparedness resource planning transboundary basins. quality, AI-driven methods improve contamination risk enable detection anomalies, track pollutants assist treatment processes regulatory practices. combined with open new perspectives monitoring resources at spatial scale, from forecasting storage variations. paper’s synthesis emphasizes AI’s immense potential hydrology; it covers latest advances future prospects field ensure
Language: Английский
Citations
0Agronomy, Journal Year: 2025, Volume and Issue: 15(3), P. 696 - 696
Published: March 13, 2025
Droughts, intensified by climate change and human activities, pose a significant threat to winter wheat cultivation in the Huang-Huai-Hai (HHH) region. Soil moisture drought indices are crucial for monitoring agricultural droughts, while challenges such as data accessibility soil heterogeneous necessitate use of numerical simulations their effective regional-scale applications. The existing simulation methods like physical process models machine learning (ML) algorithms have limitations: struggle with parameter acquisition at regional scales, ML face difficulties settings due presence crops. As more advanced complex branch ML, deep even greater limitations related crop growth management. To address these challenges, this study proposed novel hybrid system that merged model. Initially, we employed Random Forest (RF) regression model integrated multi-source environmental factors estimate prior sowing wheat, achieving an average coefficient determination (R2) 0.8618, root mean square error (RMSE) 0.0182 m3 m−3, absolute (MAE) 0.0148 m−3 across eight depths. RF provided vital parameters operation Water Balance Winter Wheat (WBWW) scale, enabling assessments combined Moisture Anomaly Percentage Index (SMAPI). Subsequent comparative analyses between system-generated results actual disaster records during two events highlighted its efficacy. Finally, utilized examine spatiotemporal variations patterns HHH region over past decades. findings revealed overall intensification conditions decline SMAPI rate −0.021% per year. Concurrently, there has been shift patterns, characterized increase both frequency extremity events, duration intensity individual decreased majority Additionally, identified northeastern, western, southern areas requiring concentrated attention targeted intervention strategies. These efforts signify notable application fusion techniques integration within big context, thereby facilitating prevention, management, mitigation
Language: Английский
Citations
0Theoretical and Applied Climatology, Journal Year: 2025, Volume and Issue: 156(4)
Published: March 19, 2025
Language: Английский
Citations
0Agronomy, Journal Year: 2025, Volume and Issue: 15(3), P. 759 - 759
Published: March 20, 2025
The rapid and accurate acquisition of soil moisture (SM) information is essential. Although Unmanned Aerial Vehicle (UAV) remote sensing technology has made significant advancements in SM monitoring, existing studies predominantly focus on developing models tailored to specific regions. transferability these across different regions remains a considerable challenge. Therefore, this study proposes transfer learning-based framework, using two representative small agricultural watersheds (Hongxing region Woniutu region) Northeast China as case studies. This framework involves pre-training model source domain fine-tuning it with limited set target samples achieve high-precision inversion. evaluates the performance three algorithms: Random Forest (RF), Convolutional Neural Network (CNN), Long Short-Term Memory (LSTM) network. Results show that fine-tuned significantly mitigates decline prediction accuracy caused by regional differences. LSTM achieved highest retrieval accuracy, following results: 10% (R = 0.615, RRMSE 15.583%), 30% 0.682, 13.97%), 50% 0.767, 16.321%). Among models, exhibited most improvement best transferability. underscores potential learning for enhancing cross-regional monitoring providing valuable insights future UAV-based monitoring.
Language: Английский
Citations
0Smart Agricultural Technology, Journal Year: 2025, Volume and Issue: unknown, P. 100905 - 100905
Published: March 1, 2025
Language: Английский
Citations
0Sustainability, Journal Year: 2025, Volume and Issue: 17(7), P. 3190 - 3190
Published: April 3, 2025
Lettuce, a vital economic crop, benefits significantly from intelligent advancements in its production, which are crucial for sustainable agriculture. Deep learning, core technology smart agriculture, has revolutionized the lettuce industry through powerful computer vision techniques like convolutional neural networks (CNNs) and YOLO-based models. This review systematically examines deep learning applications including pest disease diagnosis, precision spraying, pesticide residue detection, crop condition monitoring, growth stage classification, yield prediction, weed management, irrigation fertilization management. Notwithstanding significant contributions, several critical challenges persist, constrained model generalizability dynamic settings, exorbitant computational requirements, paucity of meticulously annotated datasets. Addressing these is essential improving efficiency, adaptability, sustainability learning-driven solutions production. By enhancing resource reducing chemical inputs, optimizing cultivation practices, contributes to broader goal explores research progress, optimization strategies, future directions strengthen learning’s role fostering farming.
Language: Английский
Citations
0Journal of the Indian Society of Remote Sensing, Journal Year: 2025, Volume and Issue: unknown
Published: April 9, 2025
Language: Английский
Citations
0