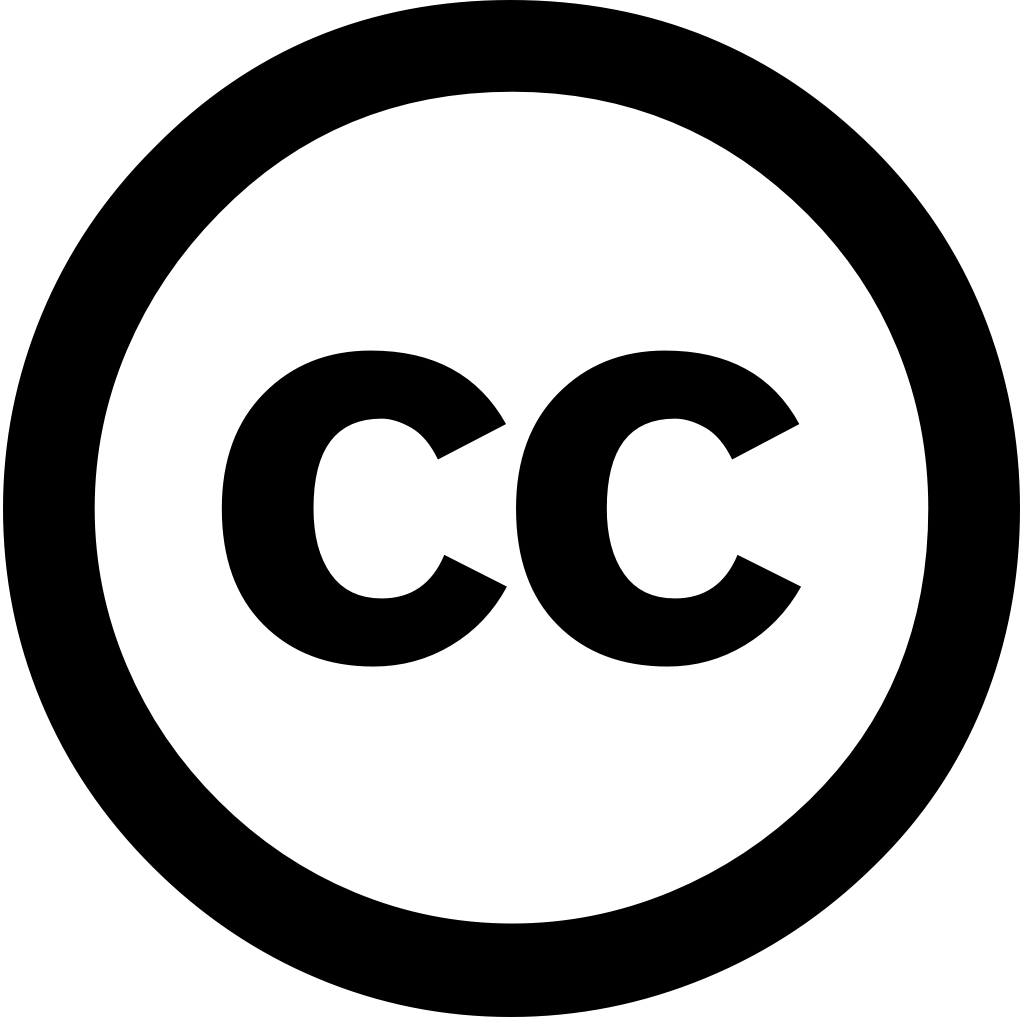
Agriculture, Journal Year: 2025, Volume and Issue: 15(2), P. 205 - 205
Published: Jan. 18, 2025
Accurate safflower recognition is a critical research challenge in the field of automated harvesting. The growing environment safflowers, including factors such as variable weather conditions unstructured environments, shooting distances, and diverse morphological characteristics, presents significant difficulties for detection. To address these challenges enable precise target complex this study proposes an improved detection model, WED-YOLO, based on YOLOv8n. Firstly, original bounding box loss function replaced with dynamic non-monotonic focusing mechanism Wise Intersection over Union (WIoU), which enhances model’s fitting ability accelerates network convergence. Then, upsampling module network’s neck substituted more efficient versatile module, DySample, to improve precision feature map upsampling. Meanwhile, EMA attention integrated into C2f backbone strengthen extraction capabilities. Finally, small-target layer incorporated head, enabling model focus small targets. trained validated using custom-built dataset. experimental results demonstrate that achieves Precision (P), Recall (R), mean Average (mAP), F1 score values 93.15%, 86.71%, 95.03%, 89.64%, respectively. These represent improvements 2.9%, 6.69%, 4.5%, 6.22% baseline model. Compared Faster R-CNN, YOLOv5, YOLOv7, YOLOv10, WED-YOLO achieved highest mAP value. It outperforms mentioned by 13.06%, 4.85%, 4.86%, 4.82%, enhanced exhibits superior lower miss rates tasks, providing robust algorithmic foundation intelligent harvesting safflowers.
Language: Английский