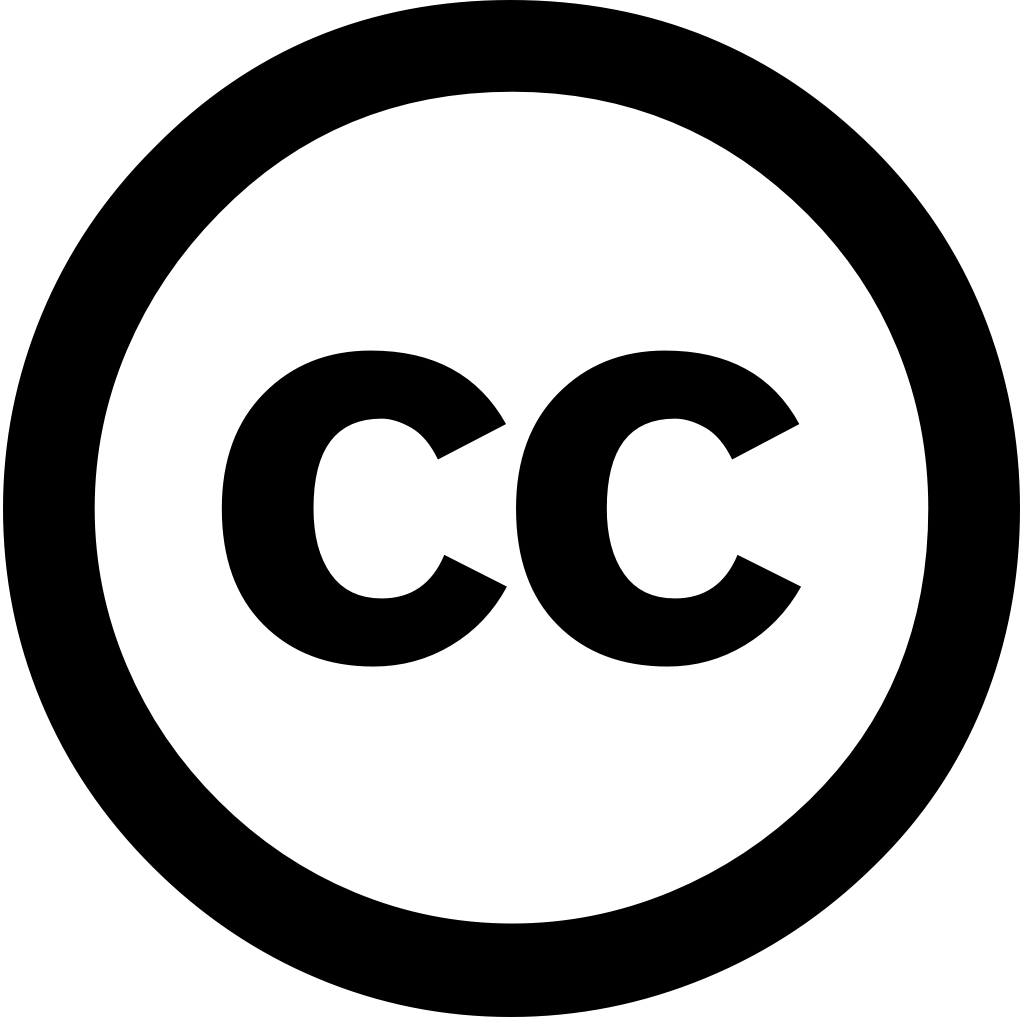
Sensors, Journal Year: 2025, Volume and Issue: 25(9), P. 2691 - 2691
Published: April 24, 2025
This study focused on addressing the issue of delayed root system development in mulberry trees during aerosol cultivation, which is attributed to asynchronous growth branches and buds. To tackle this challenge, we propose an intelligent foliar fertilizer spraying based deep learning. The incorporates a parallel robotic arm device employs trinocular vision capture image datasets tree branches. After comparing YOLOv8n with other YOLO versions, made several enhancements model. These improvements included introduction Asymptotic Feature Pyramid Network (AFPN), optimization feature extraction using MSBlock module, adoption dynamic ATSS label assignment strategy, replacement CIoU loss function Focal_XIoU function. Furthermore, artificial neural network was utilized calculate coordinates arm. experimental results demonstrate that enhanced model achieved average precision 94.48%, representing 6.05% improvement over original Additionally, prediction error for maintained at ≤1.3%. effectively enables precise location directional fertilization exhibiting lagging growth, thereby significantly promoting synchronous seedlings.
Language: Английский