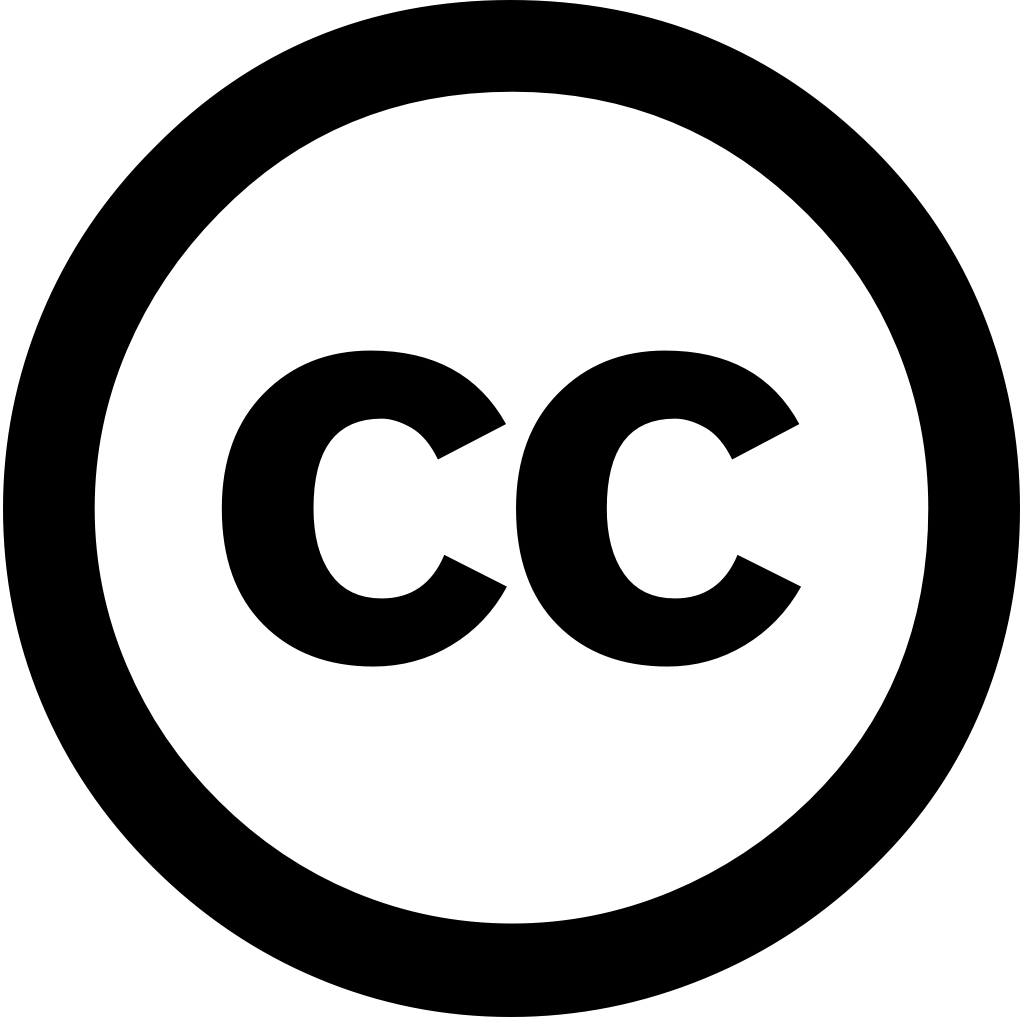
BenchCouncil Transactions on Benchmarks Standards and Evaluations, Journal Year: 2025, Volume and Issue: unknown, P. 100198 - 100198
Published: March 1, 2025
Language: Английский
BenchCouncil Transactions on Benchmarks Standards and Evaluations, Journal Year: 2025, Volume and Issue: unknown, P. 100198 - 100198
Published: March 1, 2025
Language: Английский
Measurement Sensors, Journal Year: 2022, Volume and Issue: 24, P. 100506 - 100506
Published: Oct. 5, 2022
Alzheimer's disease (AD) is one of the most prevalent types dementia, which primarily affects people over age 60. In clinical practice, it a challenging task to identify AD in its early stages, and there are currently very few reliable diagnostic systems available for identification. Additionally, studies medications have high risk failure, currently, no confirmed cure. There various stages AD: mild demented, mild, moderate. It these due demented case worsens results complete health loss along with weak memory makes unable perform daily tasks without assistance others. Early identification cases can help patients guide additional medical care stop disease's progression avoid brain damage. Recently, has been substantial amount interest applying deep learning (DL) recognition. The limitations algorithms that they cannot detect changes networks functional working networks. However, growth, scientists researchers striving build methods by using MRI images. this article, diagnoses AD, two datasets containing 6400 6330 images used, DL algorithm utilized neural network classifier VGG16 feature extractor diagnosis outcome form accuracy, precision, recall, AUC F1-score as (90.4%, 0.905, 0.904, 0.969, 0.904), (71.1%, 0.71, 0.711, 0.85, 0.71) dataset 1 2, respectively. Furthermore, compared previous studies, concluded proposed model performs better. Lastly, article applicable machine (ML) approaches be study stage
Language: Английский
Citations
113Journal of King Saud University - Computer and Information Sciences, Journal Year: 2024, Volume and Issue: 36(2), P. 101940 - 101940
Published: Jan. 24, 2024
Alzheimer's Disease (AD) is a worldwide concern impacting millions of people, with no effective treatment known to date. Unlike cancer, which has seen improvement in preventing its progression, early detection remains critical managing the burden AD. This paper suggests novel AD-DL approach for detecting AD using Deep Learning (DL) Techniques. The dataset consists pictures brain magnetic resonance imaging (MRI) used evaluate and validate suggested model. method includes stages pre-processing, DL model training, evaluation. Five models autonomous feature extraction binary classification are shown. divided into two categories: without Data Augmentation (without-Aug), CNN-without-AUG, (with-Aug), CNNs-with-Aug, CNNs-LSTM-with-Aug, CNNs-SVM-with-Aug, Transfer learning VGG16-SVM-with-Aug. main goal build best accuracy, recall, precision, F1 score, training time, testing time. recommended methodology, showing encouraging results. experimental results show that CNN-LSTM superior, an accuracy percentage 99.92%. outcomes this study lay groundwork future DL-based research identification.
Language: Английский
Citations
38Machine Learning and Knowledge Extraction, Journal Year: 2024, Volume and Issue: 6(1), P. 464 - 505
Published: Feb. 21, 2024
Alzheimer’s disease (AD) is a pressing global issue, demanding effective diagnostic approaches. This systematic review surveys the recent literature (2018 onwards) to illuminate current landscape of AD detection via deep learning. Focusing on neuroimaging, this study explores single- and multi-modality investigations, delving into biomarkers, features, preprocessing techniques. Various models, including convolutional neural networks (CNNs), recurrent (RNNs), generative are evaluated for their performance. Challenges such as limited datasets training procedures persist. Emphasis placed need differentiate from similar brain patterns, necessitating discriminative feature representations. highlights learning’s potential limitations in detection, underscoring dataset importance. Future directions involve benchmark platform development streamlined comparisons. In conclusion, while learning holds promise accurate refining models methods crucial tackle challenges enhance precision.
Language: Английский
Citations
36Expert Systems with Applications, Journal Year: 2024, Volume and Issue: 255, P. 124780 - 124780
Published: July 14, 2024
Language: Английский
Citations
19Computers in Biology and Medicine, Journal Year: 2023, Volume and Issue: 162, P. 107050 - 107050
Published: May 23, 2023
Language: Английский
Citations
42Computers in Biology and Medicine, Journal Year: 2023, Volume and Issue: 164, P. 107304 - 107304
Published: July 31, 2023
Language: Английский
Citations
34Artificial Intelligence in Medicine, Journal Year: 2024, Volume and Issue: 154, P. 102928 - 102928
Published: July 3, 2024
Language: Английский
Citations
13IEEE Transactions on Fuzzy Systems, Journal Year: 2024, Volume and Issue: 32(10), P. 5477 - 5492
Published: June 18, 2024
Language: Английский
Citations
12Aging and Disease, Journal Year: 2024, Volume and Issue: unknown, P. 0 - 0
Published: Jan. 1, 2024
Neurodegenerative diseases, particularly Alzheimer's disease and other dementias as well Parkinson's disease, are emerging profoundly significant challenges burdens to global health, a trend highlighted by the most recent Global Burden of Disease (GBD) 2021 studies. This growing impact is closely linked demographic shift toward an aging population potential long-term repercussions COVID-19 pandemic, both which have intensified prevalence severity these conditions. In this review, we explore several critical aspects complex issue, including increasing burden neurodegenerative unmet medical social needs within current care systems, unique amplified posed strategies for enhancing healthcare policy practice. We underscore urgent need cohesive, multidisciplinary approaches across medical, research, domains effectively address thereby improving quality life patients their caregivers.
Language: Английский
Citations
10Archives of Computational Methods in Engineering, Journal Year: 2025, Volume and Issue: unknown
Published: March 1, 2025
Language: Английский
Citations
1