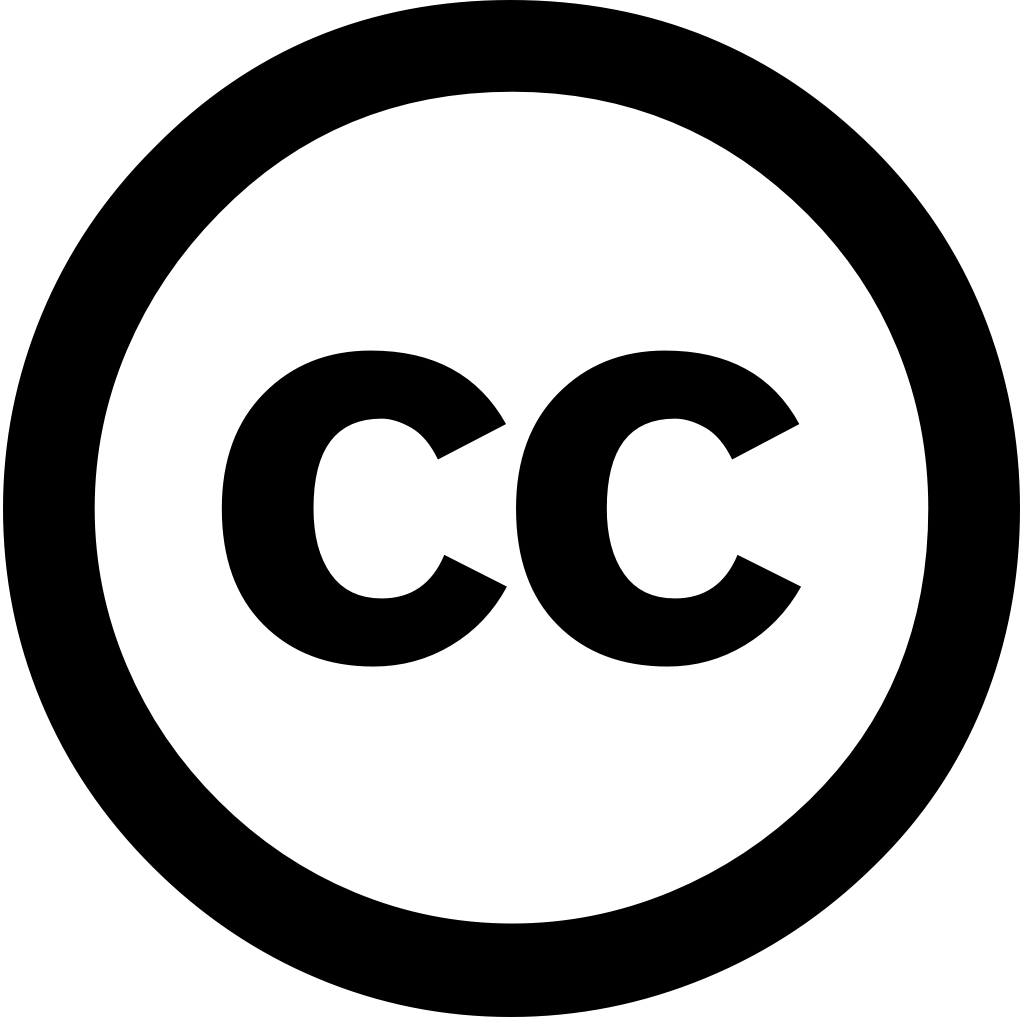
Bioengineering, Journal Year: 2023, Volume and Issue: 10(7), P. 850 - 850
Published: July 18, 2023
Artificial neural networks (ANNs) ability to learn, correct errors, and transform a large amount of raw data into beneficial medical decisions for treatment care has increased in popularity enhanced patient safety quality care. Therefore, this paper reviews the critical role ANNs providing valuable insights patients’ healthcare efficient disease diagnosis. We study different types existing literature that advance ANNs’ adaptation complex applications. Specifically, we investigate advances predicting viral, cancer, skin, COVID-19 diseases. Furthermore, propose deep convolutional network (CNN) model called ConXNet, based on chest radiography images, improve detection accuracy disease. ConXNet is trained tested using image dataset obtained from Kaggle, achieving more than 97% 98% precision, which better other state-of-the-art models, such as DeTraC, U-Net, COVID MTNet, COVID-Net, having 93.1%, 94.10%, 84.76%, 90% 94%, 95%, 85%, 92% respectively. The results show performed significantly well relatively compared with aforementioned models. Moreover, reduces time complexity by dropout layers batch normalization techniques. Finally, highlight future research directions challenges, algorithms, insufficient available data, privacy security, integration biosensing ANNs. These require considerable attention improving scope diagnostic
Language: Английский