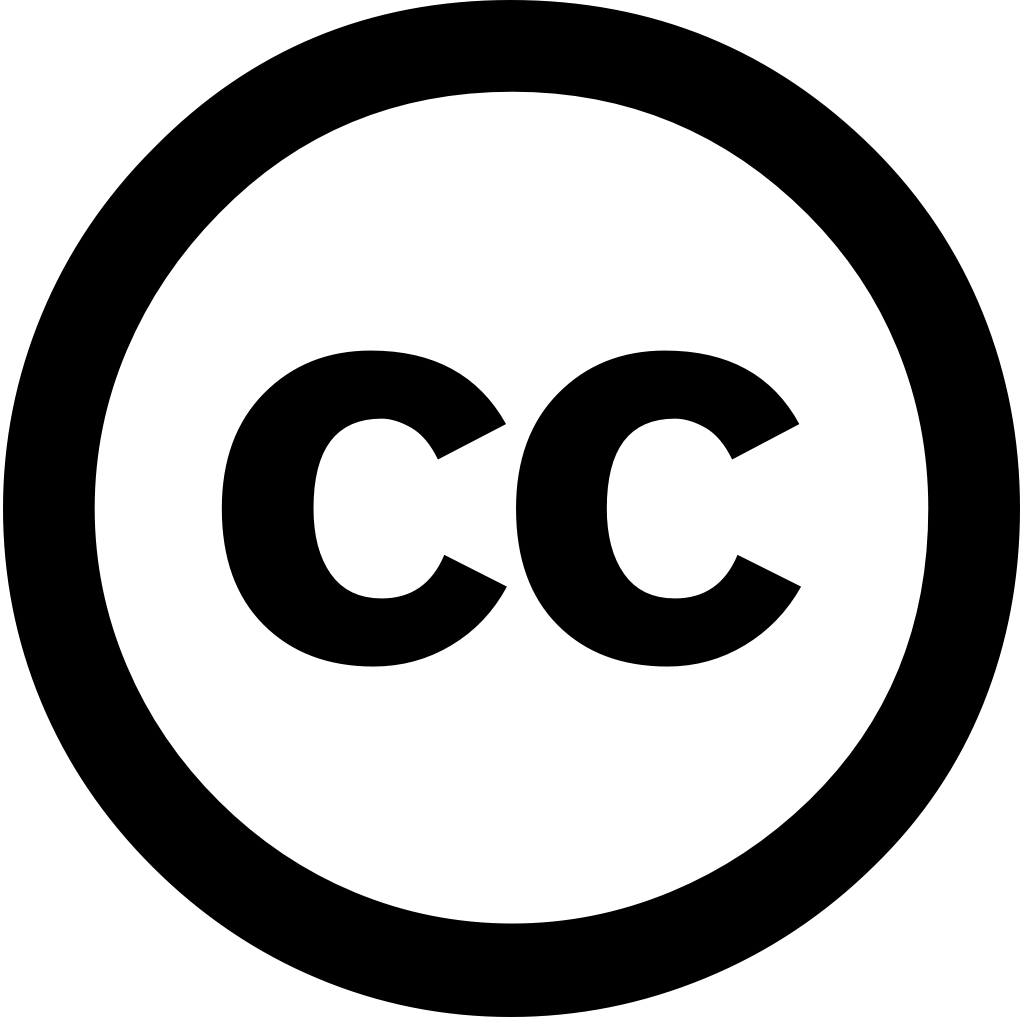
Thin-Walled Structures, Journal Year: 2025, Volume and Issue: unknown, P. 113494 - 113494
Published: May 1, 2025
Language: Английский
Thin-Walled Structures, Journal Year: 2025, Volume and Issue: unknown, P. 113494 - 113494
Published: May 1, 2025
Language: Английский
Computers in Biology and Medicine, Journal Year: 2023, Volume and Issue: 168, P. 107723 - 107723
Published: Nov. 19, 2023
Language: Английский
Citations
50Expert Systems with Applications, Journal Year: 2024, Volume and Issue: 249, P. 123747 - 123747
Published: March 20, 2024
Language: Английский
Citations
19Scientific Reports, Journal Year: 2025, Volume and Issue: 15(1)
Published: Jan. 6, 2025
This study utilizes the Breast Ultrasound Image (BUSI) dataset to present a deep learning technique for breast tumor segmentation based on modified UNet architecture. To improve accuracy, model integrates attention mechanisms, such as Convolutional Block Attention Module (CBAM) and Non-Local Attention, with advanced encoder architectures, including ResNet, DenseNet, EfficientNet. These mechanisms enable focus more effectively relevant areas, resulting in significant performance improvements. Models incorporating outperformed those without, reflected superior evaluation metrics. The effects of Dice Loss Binary Cross-Entropy (BCE) model's were also analyzed. maximized overlap between predicted actual masks, leading precise boundary delineation, while BCE achieved higher recall, improving detection areas. Grad-CAM visualizations further demonstrated that attention-based models enhanced interpretability by accurately highlighting findings denote combining framework can yield reliable accurate segmentation. Future research will explore use multi-modal imaging, real-time deployment clinical applications, performance.
Language: Английский
Citations
9Multimedia Tools and Applications, Journal Year: 2025, Volume and Issue: unknown
Published: Jan. 17, 2025
Language: Английский
Citations
3Journal of Magnetic Resonance Imaging, Journal Year: 2025, Volume and Issue: unknown
Published: Jan. 9, 2025
Breast cancer continues to be a major health concern, and early detection is vital for enhancing survival rates. Magnetic resonance imaging (MRI) key tool due its substantial sensitivity invasive breast cancers. Computer‐aided (CADe) systems enhance the effectiveness of MRI by identifying potential lesions, aiding radiologists in focusing on areas interest, extracting quantitative features, integrating with computer‐aided diagnosis (CADx) pipelines. This review aims provide comprehensive overview current state CADe MRI, technical details pipelines segmentation models including classical intensity‐based methods, supervised unsupervised machine learning (ML) approaches, latest deep (DL) architectures. It highlights recent advancements from traditional algorithms sophisticated DL such as U‐Nets, emphasizing implementation multi‐parametric acquisitions. Despite these advancements, face challenges like variable false‐positive negative rates, complexity interpreting extensive data, variability system performance, lack large‐scale studies multicentric models, limiting generalizability suitability clinical implementation. Technical issues, image artefacts need reproducible explainable algorithms, remain significant hurdles. Future directions emphasize developing more robust generalizable AI improve transparency trust among clinicians, multi‐purpose systems, incorporating large language diagnostic reporting patient management. Additionally, efforts standardize streamline protocols aim increase accessibility reduce costs, optimizing use practice. Level Evidence NA Efficacy Stage 2
Language: Английский
Citations
2Bioengineering, Journal Year: 2023, Volume and Issue: 10(4), P. 495 - 495
Published: April 20, 2023
In recent years, there has been a growing interest in developing next point-of-interest (POI) recommendation systems both industry and academia. However, current POI strategies suffer from the lack of sufficient mixing details features related to individual users their corresponding contexts. To overcome this issue, we propose deep learning model based on an attention mechanism study. The suggested technique employs that focuses pattern’s friendship, which is responsible for concentrating relevant users. compute context-aware similarities among diverse users, our six each user as inputs, including ID, hour, month, day, minute, second visiting time, explore influences spatial temporal addition, incorporate geographical information into by creating eccentricity score. Specifically, map trajectory shape, such circle, triangle, or rectangle, different value. This attention-based evaluated two widely used datasets, experimental outcomes prove noteworthy improvement over state-of-the-art recommendation.
Language: Английский
Citations
38Computers in Biology and Medicine, Journal Year: 2023, Volume and Issue: 164, P. 107255 - 107255
Published: July 10, 2023
Language: Английский
Citations
28Soft Computing, Journal Year: 2023, Volume and Issue: unknown
Published: July 22, 2023
Language: Английский
Citations
24Signal Image and Video Processing, Journal Year: 2023, Volume and Issue: 18(2), P. 1161 - 1173
Published: Oct. 29, 2023
Language: Английский
Citations
24Agricultural Water Management, Journal Year: 2024, Volume and Issue: 296, P. 108807 - 108807
Published: April 2, 2024
The reference evapotranspiration (ETo) is a key parameter in achieving sustainable use of agricultural water resources. To accurately acquire ETo under limited conditions, this study combined the northern goshawk optimization algorithm (NGO) with extreme gradient boosting (XGBoost) model to propose novel NGO-XGBoost model. performance was evaluated using meteorological data from 30 stations North China Plain and compared XGBoost, random forest (RF), k nearest neighbor (KNN) models. An ensemble embedded feature selection (EEFS) method results RF, adaptive (AdaBoost), categorical (CatBoost) models used obtain importance factors estimating ETo, thereby determine optimal combination inputs indicated that by top 3, 4, 5 important as input combinations, all achieved high estimation accuracy. It worth noting there were significant spatial differences precisions four models, but exhibited consistently precisions, global indicator (GPI) rankings 1st, range coefficient determination (R2), nash efficiency (NSE), root mean square error (RMSE), absolute (MAE) bias (MBE) 0.920–0.998, 0.902–0.998, 0.078–0.623 mm d−1, 0.058–0.430 −0.254–0.062 respectively. Furthermore, accuracy varied across different seasons, which more significantly affected humidity wind speed winter. When target station insufficient, trained historical neighboring still maintained precision. Overall, recommends reliable for provides calculating absence data.
Language: Английский
Citations
11