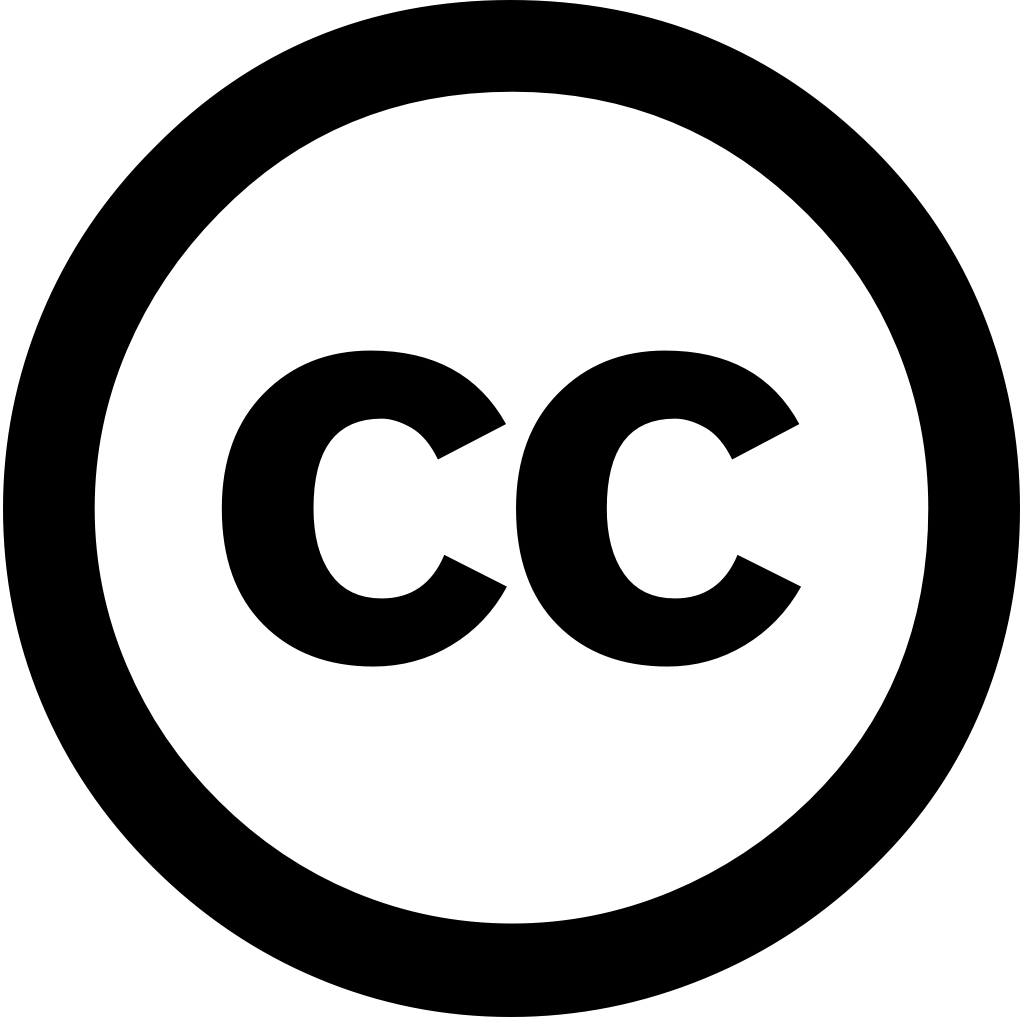
Published: Nov. 16, 2023
<p>publicly available ultrasound data and labelling </p>
Language: Английский
Published: Nov. 16, 2023
<p>publicly available ultrasound data and labelling </p>
Language: Английский
IEEE Access, Journal Year: 2024, Volume and Issue: 12, P. 15145 - 15170
Published: Jan. 1, 2024
Graph neural network (GNN) is a formidable deep learning framework that enables the analysis and modeling of intricate relationships present in data structured as graphs. In recent years, burgeoning interest has arisen exploiting latent capabilities GNN for healthcare-based applications, capitalizing on their aptitude complex unearthing profound insights from graph-structured data. However, to best our knowledge, no study systemically reviewed studies conducted healthcare domain. This furnished an all-encompassing erudite overview prevailing cutting-edge research healthcare. Through assimilation studies, current trends, recurrent challenges, promising future opportunities applications have been identified. China emerged leading country conduct GNN-based domain, followed by USA, UK, Turkey. Among various aspects healthcare, disease prediction drug discovery emerge most prominent areas focus application, indicating potential advancing diagnostic therapeutic approaches. proposed questions regarding diverse domain addressed them through in-depth analysis. can provide practitioners researchers with into landscape guide institutes, researchers, governments demonstrating ways which contribute development effective efficient systems.
Language: Английский
Citations
17IEEE Access, Journal Year: 2024, Volume and Issue: 12, P. 17273 - 17289
Published: Jan. 1, 2024
In the context of biomedical data, an anomaly could refer to a rare or new type disease, aberration from normal behavior, unexpected observation requiring immediate attention. The detection anomalies in data has direct impact on health and safety individuals. However, anomalous events are rare, diverse, infrequent. Often, collection may involve significant loss human life healthcare costs. Therefore, traditional supervised machine deep learning algorithms not be directly applicable such problems. Biomedical often collected form images, electronic records, time series. Typically, autoencoder (AE) its corresponding variant is trained identified as deviation these based reconstruction error other metrics. An Ensemble AEs (EoAEs) can serve robust approach for by combining diverse accurate views data. EoAE provide superior single encoder; however, performance depend various factors, including diversity created accuracy individual AEs, combination their outcomes. Herein, we perform comprehensive narrative literature review use EoAEs when using different types Such ensemble provides promising offering potential improvement leveraging strengths AEs. several challenges remain, need specification determination optimal number ensemble. By addressing challenges, researchers enhance effectiveness Furthermore, through this review, highlight significance evaluating comparing with that establishing agreed-upon evaluation metrics investigating normalization techniques scores. We conclude presenting open questions field future research.
Language: Английский
Citations
17Biomedical Signal Processing and Control, Journal Year: 2024, Volume and Issue: 91, P. 105970 - 105970
Published: Feb. 3, 2024
Language: Английский
Citations
5Frontiers in Big Data, Journal Year: 2023, Volume and Issue: 5
Published: Jan. 6, 2023
As one of the popular deep learning methods, convolutional neural networks (DCNNs) have been widely adopted in segmentation tasks and received positive feedback. However, tasks, DCNN-based frameworks are known for their incompetence dealing with global relations within imaging features. Although several techniques proposed to enhance reasoning DCNN, these models either not able gain satisfying performances compared traditional fully-convolutional structures or capable utilizing basic advantages CNN-based (namely ability local reasoning). In this study, current attempts combine FCNs we fully extracted self-attention by designing a novel attention mechanism 3D computation new framework (named 3DTU) three-dimensional medical image tasks. This processes images an end-to-end manner executes on both encoder side (which contains transformer) decoder is based DCNN). We tested our two independent datasets that consist MRI CT images. Experimental results clearly demonstrate method outperforms state-of-the-art methods various metrics.
Language: Английский
Citations
12Evolving Systems, Journal Year: 2023, Volume and Issue: 15(2), P. 505 - 521
Published: Dec. 23, 2023
Language: Английский
Citations
11Displays, Journal Year: 2025, Volume and Issue: unknown, P. 102971 - 102971
Published: Jan. 1, 2025
Language: Английский
Citations
0Computational and Structural Biotechnology Journal, Journal Year: 2025, Volume and Issue: 27, P. 744 - 752
Published: Jan. 1, 2025
In recent years, the application of deep convolutional neural networks (DCNNs) to medical image segmentation has shown significant promise in computer-aided detection and diagnosis (CAD). Leveraging features from different spaces (i.e. Euclidean, non-Euclidean, spectrum spaces) multi-modalities data have potential improve information available CAD system, enhancing both effectiveness efficiency. However, directly acquiring across is often prohibitively expensive time-consuming. Consequently, most current techniques are confined spatial domain, which limited utilizing scanned images MRI, CT, PET, etc. Here, we introduce an innovative Joint Spatial-Spectral Information Fusion method requires no additional collection for CAD. We translate existing single-modality into a new domain extract alternative space. Specifically, apply Discrete Cosine Transformation (DCT) enter thereby accessing supplementary feature alternate Recognizing that typically necessitates complex alignment modules, contrastive loss function achieving before synchronizing spaces. Our empirical results illustrate greater our model harnessing spectrum-based space affirm its superior performance against influential state-of-the-art baselines. The code at https://github.com/Auroradsy/SIN-Seg.
Language: Английский
Citations
0Alexandria Engineering Journal, Journal Year: 2025, Volume and Issue: 120, P. 547 - 560
Published: Feb. 24, 2025
Language: Английский
Citations
0Scientific Data, Journal Year: 2025, Volume and Issue: 12(1)
Published: March 7, 2025
Abstract The field of supervised automated medical imaging segmentation suffers from relatively small datasets with ground truth labels. This is especially true for challenging problems that target structures low contrast and ambiguous boundaries, such as glass opacities consolidation in chest computed tomography images. In this work, we make available the first public dataset opacity lungs Long COVID patients. Iowa-UNICAMP (LongCIU) was built by three independent expert annotators, blindly segmenting same 90 selected axial slices manually, without using any initialization. includes final consensus addition to individual each annotator (360 total). a valuable resource training validating new methods studying interrater uncertainty lung tomography.
Language: Английский
Citations
0Published: April 10, 2025
Language: Английский
Citations
0