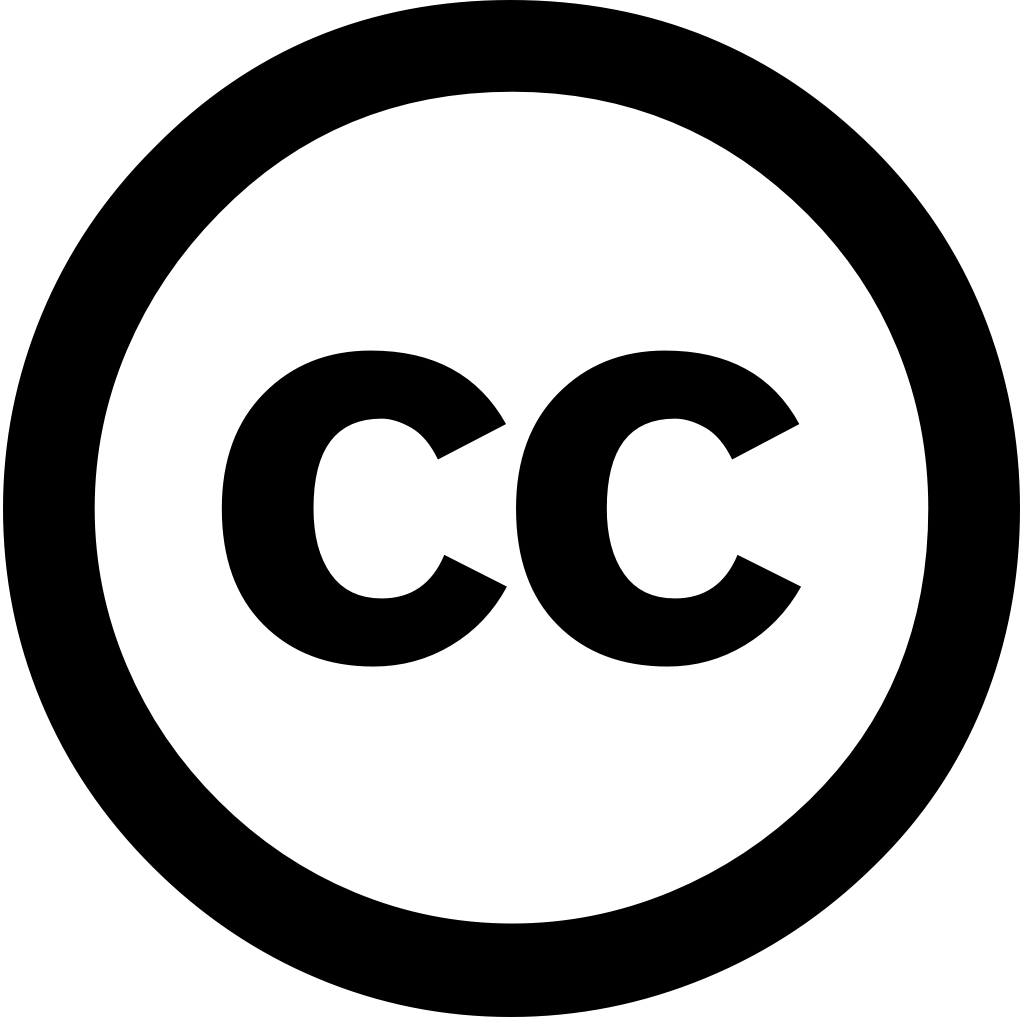
Published: Nov. 16, 2023
<p>publicly available ultrasound data and labelling </p>
Language: Английский
Published: Nov. 16, 2023
<p>publicly available ultrasound data and labelling </p>
Language: Английский
Meta-Radiology, Journal Year: 2023, Volume and Issue: 1(3), P. 100046 - 100046
Published: Nov. 1, 2023
Recent years have shown great merits in utilizing neuroimaging data to understand brain structural and functional changes, as well its relationship different neurodegenerative diseases other clinical phenotypes. Brain networks, derived from modalities, attracted increasing attention due their potential gain system-level insights characterize dynamics abnormalities neurological conditions. Traditional methods aim pre-define multiple topological features of networks relate these measures or demographical variables. With the enormous successes deep learning techniques, graph played significant roles network analysis. In this survey, we first provide a brief overview neuroimaging-derived networks. Then, focus on presenting comprehensive both traditional state-of-the-art deep-learning for mining. Major models, objectives are reviewed within paper. Finally, discuss several promising research directions field.
Language: Английский
Citations
10Deleted Journal, Journal Year: 2024, Volume and Issue: 37(3), P. 1067 - 1085
Published: Feb. 15, 2024
Abstract This study proposes a novel approach for breast tumor classification from ultrasound images into benign and malignant by converting the region of interest (ROI) 2D image 3D representation using point-e system, allowing in-depth analysis underlying characteristics. Instead relying solely on imaging features, this method extracts mesh features that describe patterns more precisely. Ten informative medically relevant are extracted assessed with two feature selection techniques. Additionally, pattern has been conducted to determine feature’s significance. A table dimensions 445 × 12 is generated graph constructed, considering rows as nodes relationships among edges. The Spearman correlation coefficient employed identify edges between strongly connected (with score greater than or equal 0.7), resulting in containing 56,054 nodes. attention network (GAT) proposed task model optimized an ablation study, highest accuracy 99.34%. performance compared ten machine learning (ML) models one-dimensional convolutional neural where test these ranges 73 91%. Our mesh-based approach, coupled GAT, yields promising classification, outperforming traditional models, potential reduce time effort radiologists providing reliable diagnostic system.
Language: Английский
Citations
3Biomedical Signal Processing and Control, Journal Year: 2023, Volume and Issue: 85, P. 104905 - 104905
Published: March 22, 2023
Language: Английский
Citations
8Published: Jan. 1, 2024
Download This Paper Open PDF in Browser Add to My Library Share: Permalink Using these links will ensure access this page indefinitely Copy URL DOI
Language: Английский
Citations
3Biomedical Signal Processing and Control, Journal Year: 2024, Volume and Issue: 91, P. 105912 - 105912
Published: Jan. 5, 2024
Language: Английский
Citations
2Mathematics, Journal Year: 2024, Volume and Issue: 12(7), P. 940 - 940
Published: March 22, 2024
The hippocampus is a crucial brain structure involved in memory formation, spatial navigation, emotional regulation, and learning. An accurate MRI image segmentation of the human plays an important role multiple neuro-imaging research clinical practice, such as diagnosing neurological diseases guiding surgical interventions. While most studies focus on using T1-weighted or T2-weighted scans, we explore use diffusion-weighted (dMRI), which offers unique insights into microstructural properties hippocampus. Particularly, utilize various anisotropy measures derived from diffusion including fractional anisotropy, mean diffusivity, axial radial for multi-contrast deep learning approach to segmentation. To exploit benefits offered by contrasts dMRI images segmentation, introduce innovative multimodal architecture integrating cross-attention mechanisms. Our proposed framework comprises multi-head encoder designed transform each contrast distinct latent spaces, generating separate feature maps. Subsequently, employ gated unit following encoder, facilitates creation attention maps between every pair contrasts. These serve enrich maps, thereby enhancing their effectiveness task. In final stage, decoder employed produce predictions utilizing attention-enhanced experimental outcomes demonstrate efficacy our highlight over single-contrast
Language: Английский
Citations
1Journal of Biomedical Informatics, Journal Year: 2023, Volume and Issue: 143, P. 104407 - 104407
Published: June 3, 2023
Language: Английский
Citations
2International Journal of Advanced Computer Science and Applications, Journal Year: 2024, Volume and Issue: 15(4)
Published: Jan. 1, 2024
The constant need for robust and efficient COVID-19 detection methodologies has prompted the exploration of advanced techniques in medical imaging analysis. This paper presents a novel framework that leverages Graph Convolutional Neural Networks (GCNNs) to enhance from CT scan X-Ray images. Hence, GCNN parameters were tuned by hybrid optimization gain more exact detection. Therefore, technique known as Hybrid NADAM Prediction (NAGNP). is designed achieve efficiency through strategy. methodology involves constructing graph representations Chest X-ray or images, where nodes encapsulate critical image patches regions interest. These graphs are fed into architectures tailored graph-based data, facilitating intricate feature extraction information aggregation. A approach employed optimize model's performance, encompassing fine-tuning hyperparameters strategic model techniques. Through rigorous evaluation validation using diverse datasets, our demonstrates promising results accurate diagnosis. Integrating GCNNs viable pathway toward reliable practical diagnostic tools combating ongoing pandemic.
Language: Английский
Citations
0Operations Research Forum, Journal Year: 2024, Volume and Issue: 5(3)
Published: Sept. 11, 2024
Language: Английский
Citations
0Frontiers in Big Data, Journal Year: 2023, Volume and Issue: 6
Published: March 2, 2023
The accurate segmentation of nuclei is crucial for cancer diagnosis and further clinical treatments. To successfully train a network in fully-supervised manner particular type organ or cancer, we need the dataset with ground-truth annotations. However, such well-annotated datasets are highly rare, manually labeling an unannotated expensive, time-consuming, tedious process. Consequently, require to discover way training unlabeled dataset. In this paper, propose model named NuSegUDA on (target domain). It achieved by applying Unsupervised Domain Adaptation (UDA) technique help another labeled (source domain) that may come from different organ, source. We apply UDA at both feature space output space. additionally utilize reconstruction incorporate adversarial learning into it so source-domain images can be accurately translated target-domain network. validate our proposed two public datasets, obtain significant improvement as compared baseline methods. Extensive experiments also verify contribution newly image loss, target-translated source supervised loss performance boost NuSegUDA. Finally, considering scenario when have small number annotations available target domain, extend work NuSegSSDA, Semi-Supervised (SSDA) based approach.
Language: Английский
Citations
1