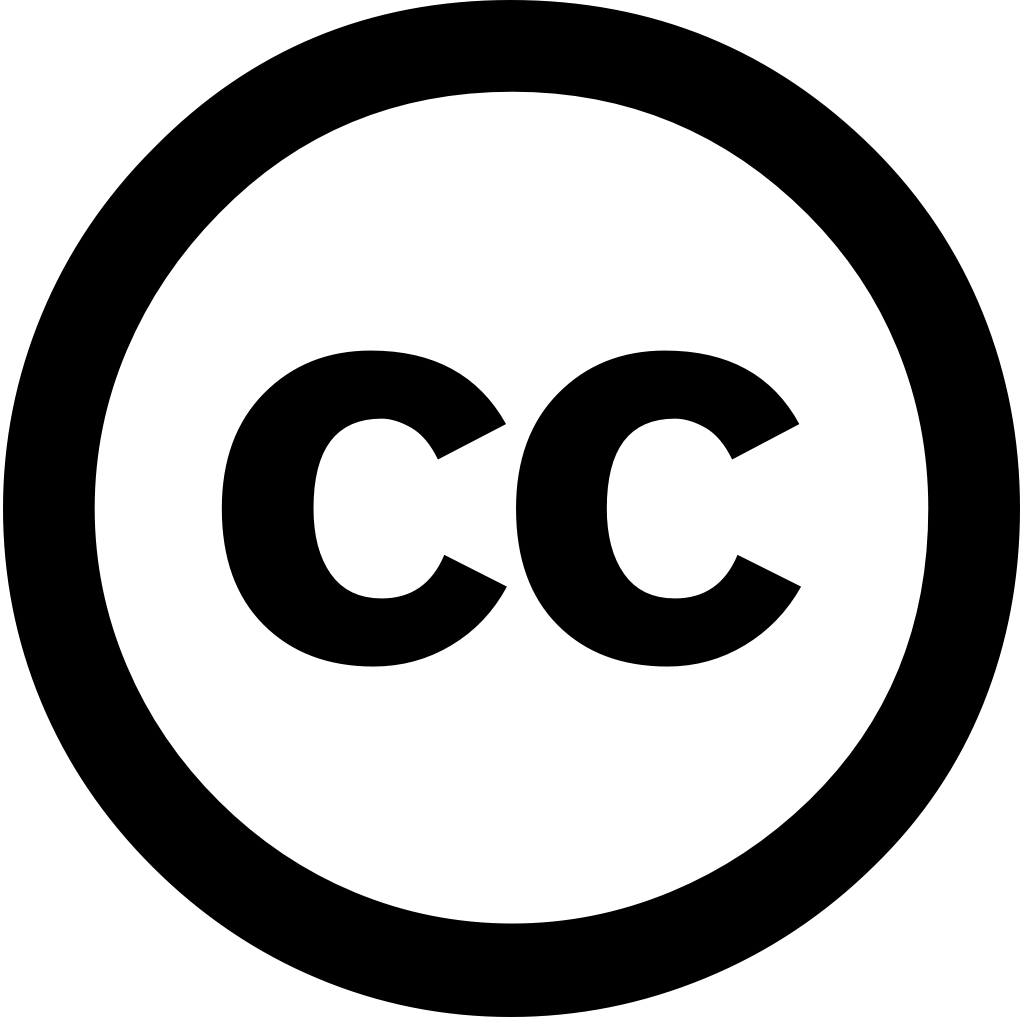
Journal Of Big Data, Journal Year: 2024, Volume and Issue: 11(1)
Published: Oct. 4, 2024
Language: Английский
Journal Of Big Data, Journal Year: 2024, Volume and Issue: 11(1)
Published: Oct. 4, 2024
Language: Английский
Journal of Computational Design and Engineering, Journal Year: 2024, Volume and Issue: 11(3), P. 111 - 136
Published: March 22, 2024
Abstract In the context of increasing data scale, contemporary optimization algorithms struggle with cost and complexity in addressing feature selection (FS) problem. This paper introduces a Harris hawks (HHO) variant, enhanced multi-strategy augmentation (CXSHHO), for FS. The CXSHHO incorporates communication collaboration strategy (CC) into baseline HHO, facilitating better information exchange among individuals, thereby expediting algorithmic convergence. Additionally, directional crossover (DX) component refines algorithm's ability to thoroughly explore space. Furthermore, soft-rime (SR) broadens population diversity, enabling stochastic exploration an extensive decision space reducing risk local optima entrapment. CXSHHO's global efficacy is demonstrated through experiments on 30 functions from CEC2017, where it outperforms 15 established algorithms. Moreover, presents novel FS method based CXSHHO, validated across 18 varied datasets UCI. results confirm effectiveness identifying subsets features conducive classification tasks.
Language: Английский
Citations
0Applied Soft Computing, Journal Year: 2024, Volume and Issue: 163, P. 111836 - 111836
Published: June 18, 2024
Language: Английский
Citations
0Journal of Computational Design and Engineering, Journal Year: 2024, Volume and Issue: 11(5), P. 1 - 28
Published: Aug. 8, 2024
Abstract In mining mineral resources, it is vital to monitor the stability of rock body in real time, reasonably regulate area ground pressure concentration, and guarantee safety personnel equipment. The microseismic signals generated by monitoring rupture can effectively predict disaster, but current technology not ideal. order address issue deep wells, this research suggests a machine learning-based model for predicting phenomena. First, work presents random spare, double adaptive weight, Gaussian–Cauchy fusion strategies as additions multi-verse optimizer (MVO) an enhanced MVO algorithm (RDGMVO). Subsequently, RDGMVO-Fuzzy K-Nearest Neighbours (RDGMVO-FKNN) prediction presented combining with FKNN classifier. experimental section compares 12 traditional recently algorithms RDGMVO, demonstrating latter’s excellent benchmark optimization performance remarkable improvement effect. Next, comparison experiment, classical classifier dataset feature selection experiment confirm precision RDGMVO-FKNN problem. According results, has accuracy above 89%, indicating that reliable accurate method classifying occurrences. Code been available at https://github.com/GuaipiXiao/RDGMVO.
Language: Английский
Citations
0Journal Of Big Data, Journal Year: 2024, Volume and Issue: 11(1)
Published: Oct. 4, 2024
Language: Английский
Citations
0