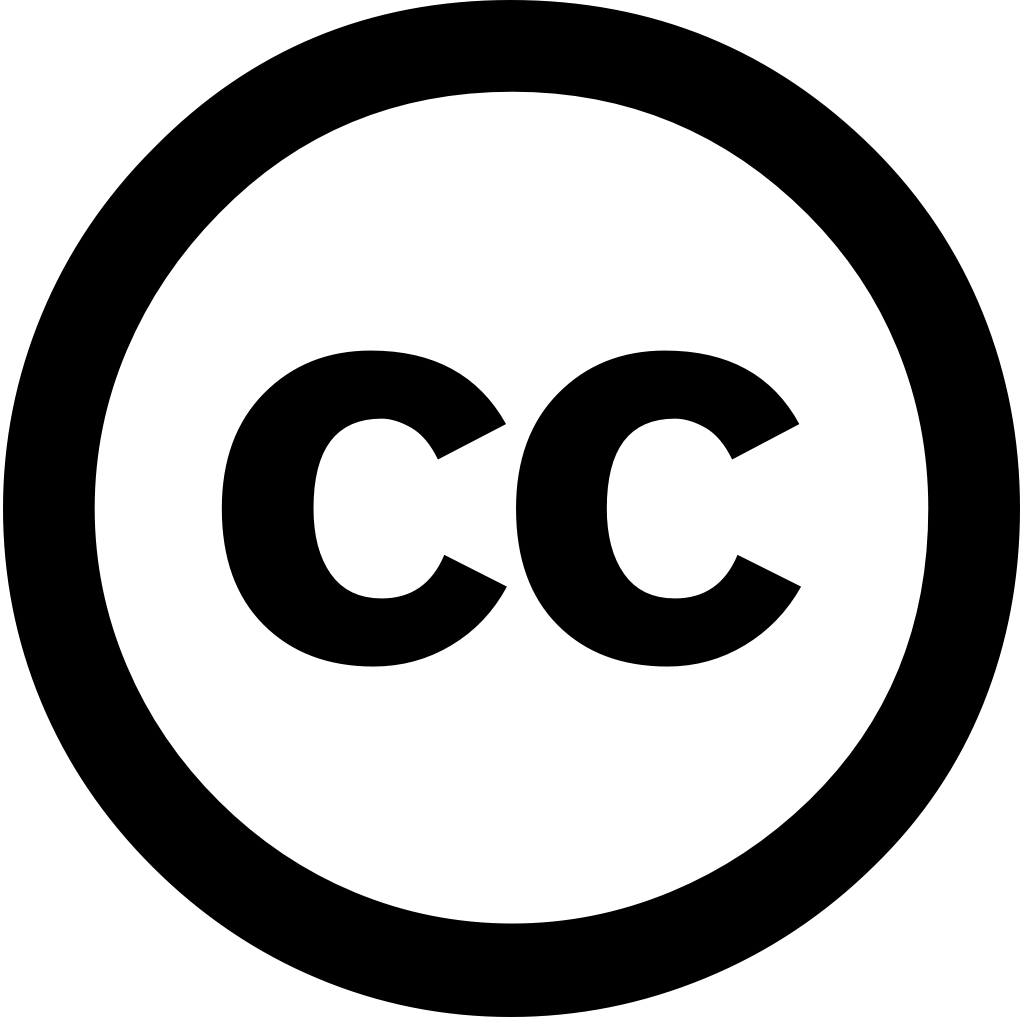
PeerJ Computer Science, Journal Year: 2024, Volume and Issue: 10, P. e2564 - e2564
Published: Dec. 23, 2024
The complex environments and unpredictable states within transportation networks have a significant impact on their operations. To enhance the level of intelligence in networks, we propose visual scene feature clustering analysis method based 3D sensors adaptive fuzzy control to address various encountered. Firstly, construct extraction framework for scenes using employ series processing operators repair cracks noise images. Subsequently, introduce aggregation approach an algorithm carefully screen preprocessed features. Finally, by designing similarity matrix network environment, obtain recognition results current environment state. Experimental demonstrate that our outperforms competitive approaches with mean average precision (mAP) value 0.776, serving as theoretical foundation perception enhancing intelligence.
Language: Английский