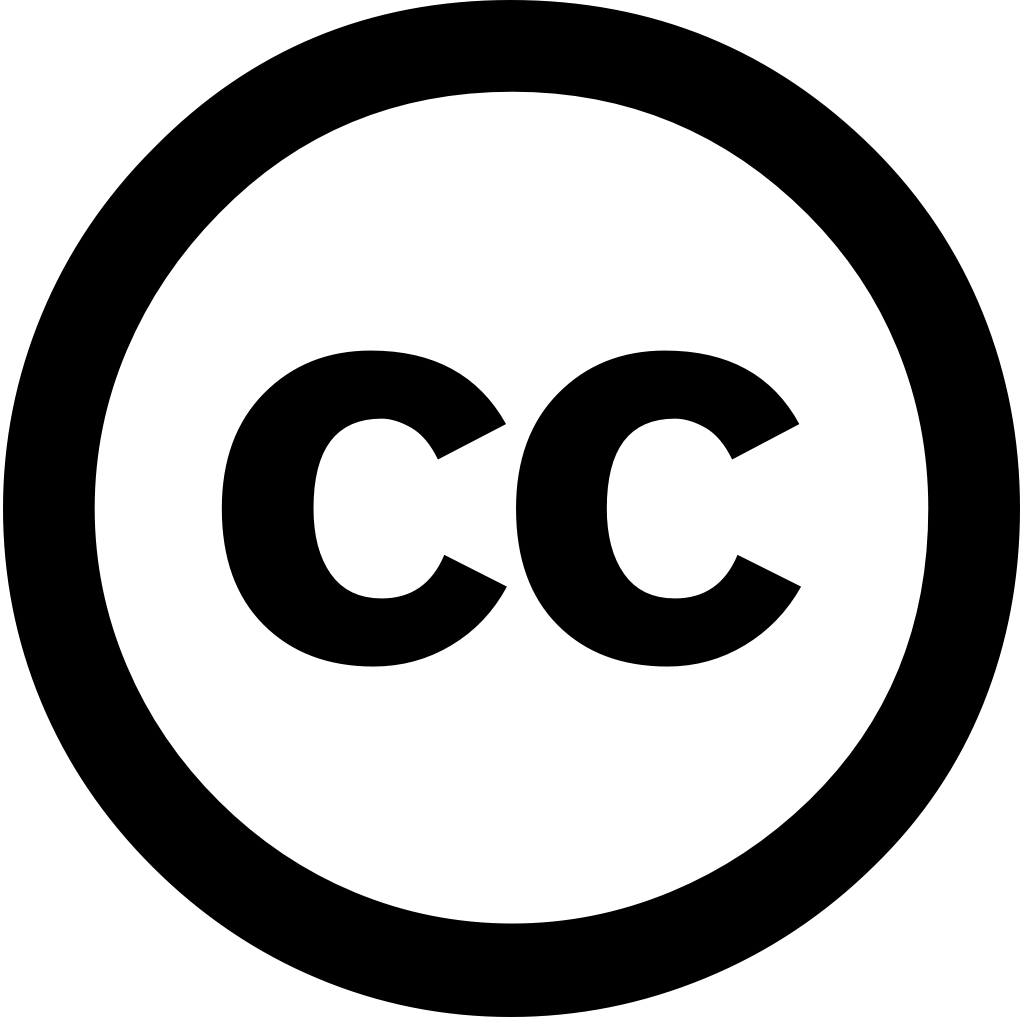
Bioengineering, Journal Year: 2024, Volume and Issue: 11(12), P. 1274 - 1274
Published: Dec. 15, 2024
Intracerebral hemorrhage (ICH) and perihematomal edema (PHE) are key imaging markers of primary secondary brain injury in hemorrhagic stroke. Accurate segmentation quantification ICH PHE can help with prognostication guide treatment planning. In this study, we combined Swin-Unet Transformers nnU-NETv2 convolutional network for on non-contrast head CTs. We also applied test-time data augmentations to assess individual-level prediction uncertainty, ensuring high confidence prediction. The model was trained 1782 CT scans from a multicentric trial tested two independent datasets Yale (n = 396) University Berlin Charité Hospital Medical Center Hamburg-Eppendorf 943). Model performance evaluated the Dice coefficient Volume Similarity (VS). Our dual Swin-nnUNET achieved median (95% interval) 0.93 (0.90–0.95) VS 0.97 (0.95–0.98) ICH, 0.70 (0.64–0.75) 0.87 (0.80–0.93) cohort. 0.86 (0.80–0.90) 0.91 (0.85–0.95) 0.65 (0.56–0.70) (0.77–0.93) Berlin/Hamburg-Eppendorf Prediction uncertainty associated lower accuracy, smaller ICH/PHE volumes, infratentorial location. results highlight benefits transformer-convolutional neural architecture augmentation quantification.
Language: Английский