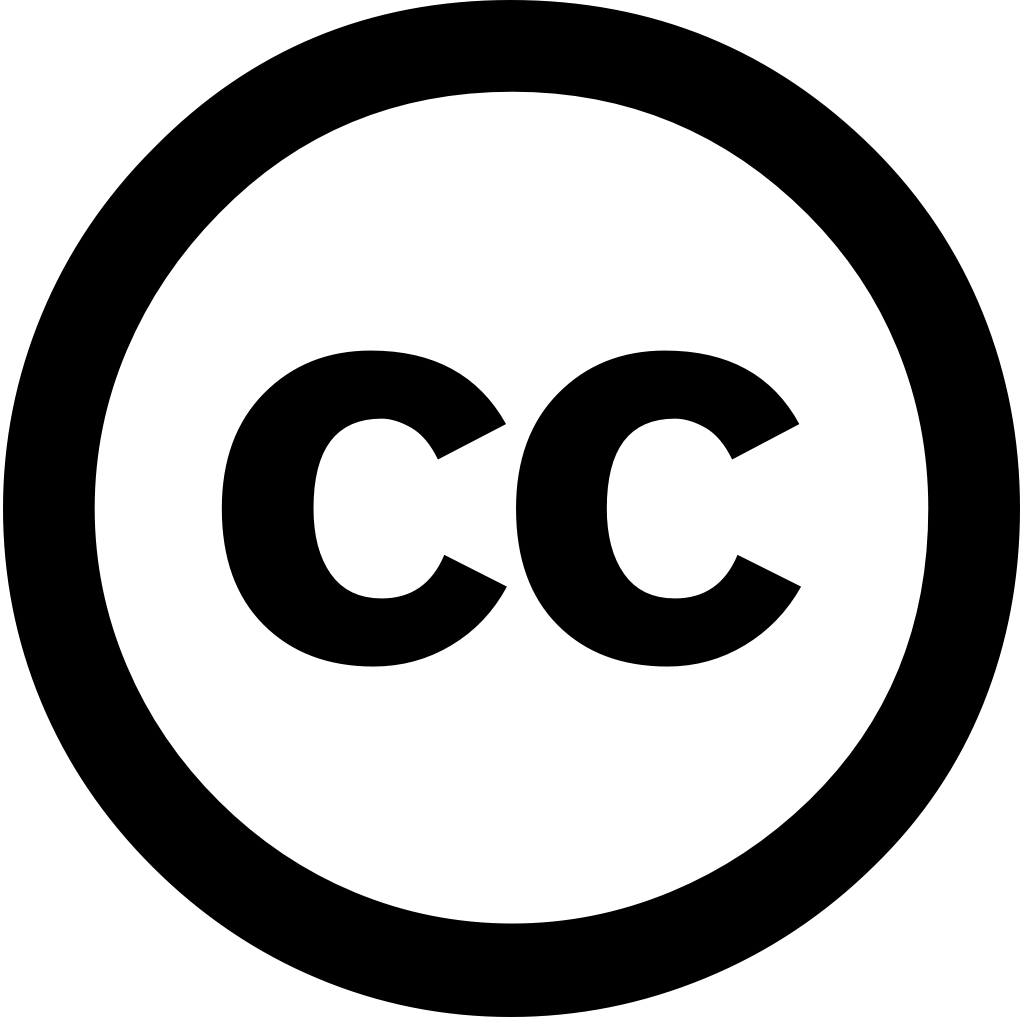
Journal of bone oncology, Journal Year: 2024, Volume and Issue: 51, P. 100659 - 100659
Published: Dec. 31, 2024
Colorectal cancer is a prevalent malignancy with significant risk of metastasis, including to bones, which severely impacts patient outcomes. Accurate prediction bone metastasis crucial for optimizing treatment strategies and improving prognosis. This study aims develop predictive model combining radiomics Vision Transformer (ViT) deep learning techniques assess the in colorectal patients using both plain contrast-enhanced CT images. We conducted retrospective analysis 155 patients, 81 74 without. Radiomic features were extracted from segmented tumors on LASSO regression was applied select key features, then used build traditional machine models, Support Vector Machine (SVM), K-Nearest Neighbors (KNN), Random Forest, LightGBM, XGBoost. Additionally, dual-modality ViT trained same images, late fusion strategy employed combine outputs different modalities. Model performance evaluated AUC-ROC, accuracy, sensitivity, specificity, differences statistically assessed DeLong's test. The demonstrated superior performance, achieving an AUC 0.918 test set, significantly outperforming all radiomics-based models. SVM model, while best among still underperformed compared model. model's strength lies its ability capture complex spatial relationships long-range dependencies within imaging data, are often missed by confirmed statistical significance enhanced highlighting potential as powerful tool predicting patients. integration ViT-based offers robust accurate method analyze data provides greater precision assessment, can improve clinical decision-making personalized strategies. These findings underscore promise advanced models enhancing accuracy prediction. Further validation larger, multicenter studies recommended confirm generalizability these results.
Language: Английский