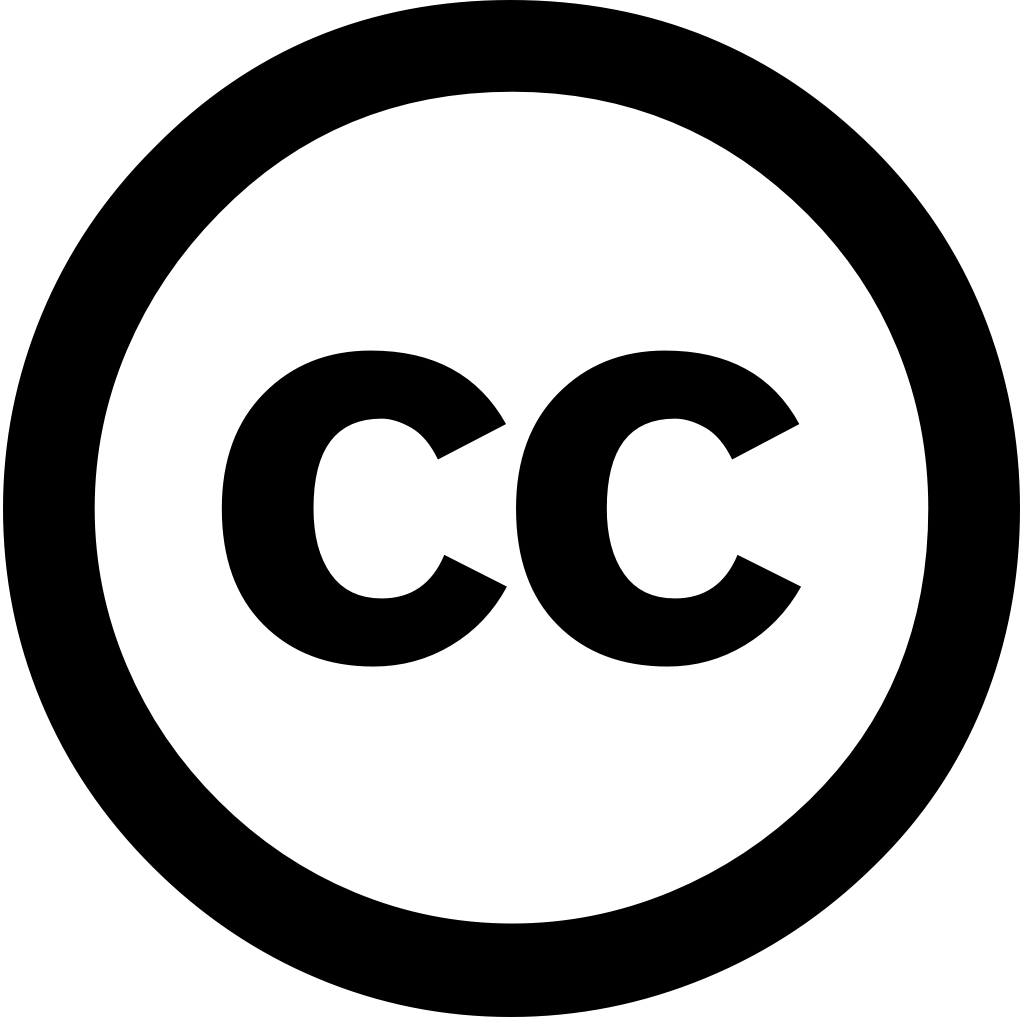
Sensors, Journal Year: 2024, Volume and Issue: 24(22), P. 7161 - 7161
Published: Nov. 7, 2024
To address the limitations of traditional optimization methods in achieving high accuracy high-dimensional problems, this paper introduces snow leopard (SLO) algorithm. SLO is a novel meta-heuristic approach inspired by territorial behaviors leopards. By emulating strategies such as territory delineation, neighborhood relocation, and dispute mechanisms, achieves balance between exploration exploitation, to navigate vast complex search spaces. The algorithm's performance was evaluated using CEC2017 benchmark genetic data feature selection tasks, demonstrating SLO's competitive advantage solving problems. In experiments, ranked first Friedman test, outperforming several well-known algorithms, including ETBBPSO, ARBBPSO, HCOA, AVOA, WOA, SSA, HHO. effective application further highlights its adaptability practical utility, marking significant progress field selection.
Language: Английский