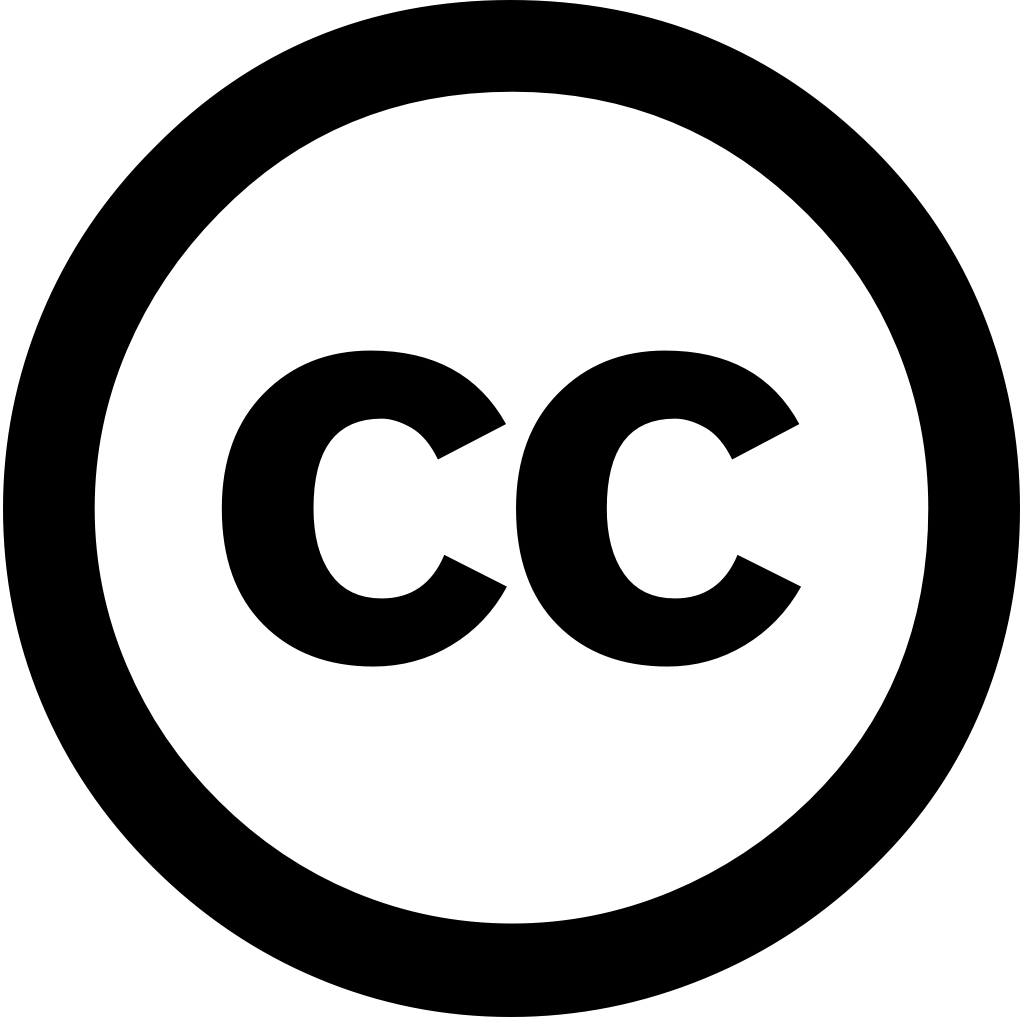
International Journal of Disaster Risk Reduction, Journal Year: 2024, Volume and Issue: 114, P. 104943 - 104943
Published: Oct. 31, 2024
Language: Английский
International Journal of Disaster Risk Reduction, Journal Year: 2024, Volume and Issue: 114, P. 104943 - 104943
Published: Oct. 31, 2024
Language: Английский
Agricultural Water Management, Journal Year: 2025, Volume and Issue: 309, P. 109349 - 109349
Published: Feb. 5, 2025
Language: Английский
Citations
1Machine Learning and Knowledge Extraction, Journal Year: 2025, Volume and Issue: 7(1), P. 13 - 13
Published: Feb. 7, 2025
The integration of machine learning (ML) with big data has revolutionized industries by enabling the extraction valuable insights from vast and complex datasets. This convergence fueled advancements in various fields, leading to development sophisticated models capable addressing complicated problems. However, application ML environments presents significant challenges, including issues related scalability, quality, model interpretability, privacy, handling diverse high-velocity data. survey provides a comprehensive overview current state applications data, systematically identifying key challenges recent field. By critically analyzing existing methodologies, this paper highlights gaps research proposes future directions for scalable, interpretable, privacy-preserving techniques. Additionally, addresses ethical societal implications emphasizing need responsible equitable approaches harnessing these technologies. presented aim guide contribute ongoing discourse on
Language: Английский
Citations
1Results in Engineering, Journal Year: 2025, Volume and Issue: unknown, P. 104349 - 104349
Published: Feb. 1, 2025
Language: Английский
Citations
1Remote Sensing, Journal Year: 2024, Volume and Issue: 16(15), P. 2842 - 2842
Published: Aug. 2, 2024
Wildfire susceptibility maps play a crucial role in preemptively identifying regions at risk of future fires and informing decisions related to wildfire management, thereby aiding mitigating the risks potential damage posed by wildfires. This study employs eXplainable Artificial Intelligence (XAI) techniques, particularly SHapley Additive exPlanations (SHAP), map Izmir Province, Türkiye. Incorporating fifteen conditioning factors spanning topography, climate, anthropogenic influences, vegetation characteristics, machine learning (ML) models (Random Forest, XGBoost, LightGBM) were used predict wildfire-prone areas using freely available active fire pixel data (MODIS Active Fire Collection 6 MCD14ML product). The evaluation trained ML showed that Random Forest (RF) model outperformed XGBoost LightGBM, achieving highest test accuracy (95.6%). All classifiers demonstrated strong predictive performance, but RF excelled sensitivity, specificity, precision, F-1 score, making it preferred for generating conducting SHAP analysis. Unlike prevailing approaches focusing solely on global feature importance, this fills critical gap employing summary dependence plots comprehensively assess each factor’s contribution, enhancing explainability reliability results. analysis reveals clear associations between such as wind speed, temperature, NDVI, slope, distance villages with increased susceptibility, while rainfall streams exhibit nuanced effects. spatial distribution classes highlights areas, flat coastal near settlements agricultural lands, emphasizing need enhanced awareness preventive measures. These insights inform targeted management strategies, highlighting importance tailored interventions like firebreaks management. However, challenges remain, including ensuring selected factors’ adequacy across diverse regions, addressing biases from resampling spatially varied data, refining broader applicability.
Language: Английский
Citations
6Applied Data Science and Analysis, Journal Year: 2024, Volume and Issue: 2024, P. 121 - 147
Published: Aug. 7, 2024
There is a considerable threat present in genres such as machine learning due to adversarial attacks which include purposely feeding the system with data that will alter decision region. These are committed presenting different models way model would be wrong its classification or prediction. The field of study still relatively young and has develop strong bodies scientific research eliminate gaps current knowledge. This paper provides literature review defenses based on highly cited articles conference published Scopus database. Through assessment 128 systematic articles: 80 original papers 48 till May 15, 2024, this categorizes reviews from domains, Graph Neural Networks, Deep Learning Models for IoT Systems, others. posits findings identified metrics, citation analysis, contributions these studies while suggesting area’s further development robustness’ protection mechanisms. objective work basic background defenses, need maintaining adaptability platforms. In context, contribute building efficient sustainable mechanisms AI applications various industries
Language: Английский
Citations
5Remote Sensing in Earth Systems Sciences, Journal Year: 2025, Volume and Issue: unknown
Published: Jan. 8, 2025
Language: Английский
Citations
0Results in Engineering, Journal Year: 2025, Volume and Issue: unknown, P. 104137 - 104137
Published: Jan. 1, 2025
Language: Английский
Citations
0Journal of Environmental Management, Journal Year: 2025, Volume and Issue: 379, P. 124835 - 124835
Published: March 7, 2025
Language: Английский
Citations
0SN Computer Science, Journal Year: 2025, Volume and Issue: 6(3)
Published: March 14, 2025
Language: Английский
Citations
0Green Technologies and Sustainability, Journal Year: 2025, Volume and Issue: unknown, P. 100202 - 100202
Published: March 1, 2025
Language: Английский
Citations
0