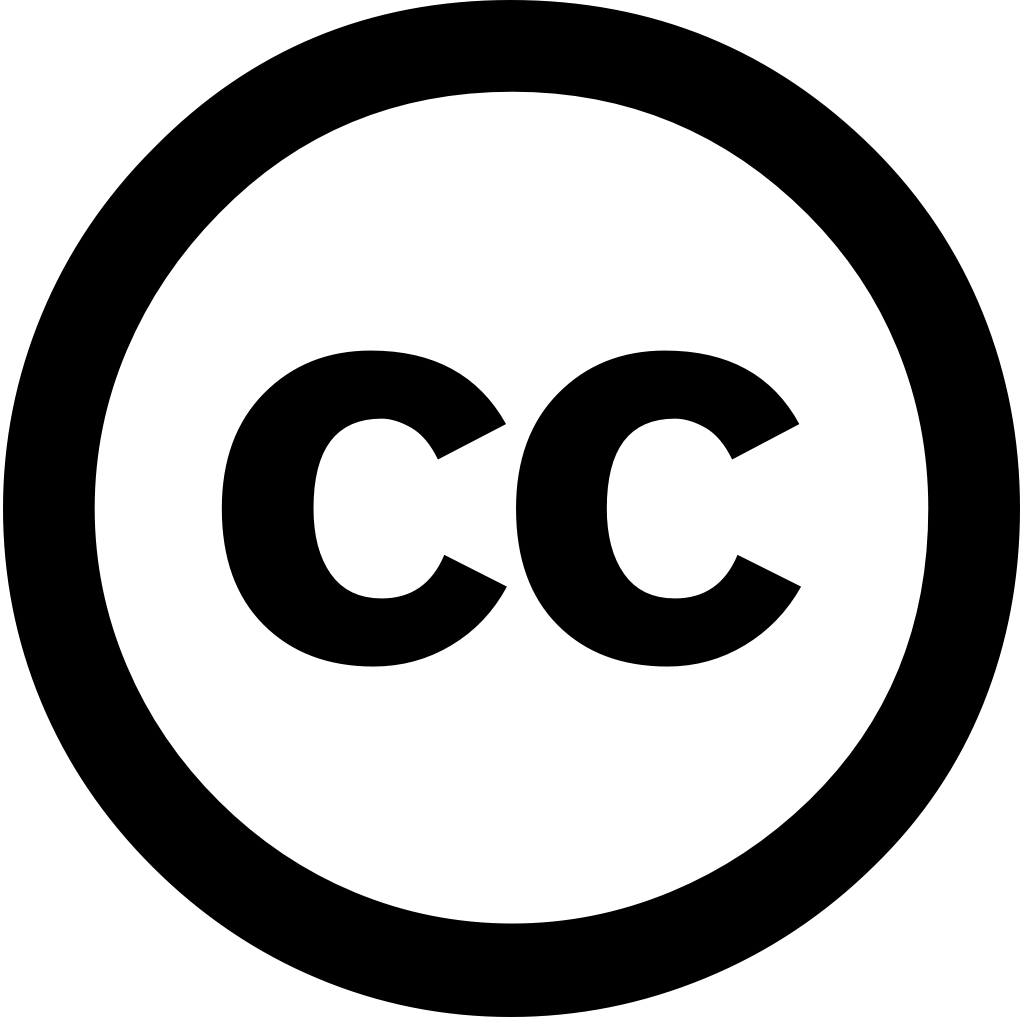
International Journal of Mechanical Sciences, Journal Year: 2024, Volume and Issue: 286, P. 109824 - 109824
Published: Nov. 12, 2024
Language: Английский
International Journal of Mechanical Sciences, Journal Year: 2024, Volume and Issue: 286, P. 109824 - 109824
Published: Nov. 12, 2024
Language: Английский
Computers and Geotechnics, Journal Year: 2024, Volume and Issue: 167, P. 106085 - 106085
Published: Jan. 16, 2024
Language: Английский
Citations
7Mechanics of Advanced Materials and Structures, Journal Year: 2024, Volume and Issue: unknown, P. 1 - 19
Published: Dec. 17, 2024
Machine learning (ML) models are widely used across numerous scientific and engineering disciplines due to their exceptional performance, flexibility, prediction quality, ability handle highly complex problems if appropriate data available. One example of such areas which has attracted a lot attentions in the last couple years is integration data-driven approaches material modeling. There been several successful researches implementing ML-based constitutive instead classical phenomenological for various materials, particularly those with non-linear mechanical behaviors. This review paper aims systematically investigate literature on materials classify these based suitability non-linearity including Non-linear elasticity (hyperelasticity), plasticity, visco-elasticity, visco-plasticity. Furthermore, we also reviewed compared that have applied architectured as groups designed represent specific behaviors might not exist conventional categories. The other goal this provide initial steps understanding modeling, artificial neural networks (ANN), Gaussian processes, random forests (RF), generated adversarial (GANs), support vector machines (SVM), different regression physics-informed (PINN). outlines collection methods, types data, processing approaches, theoretical background ML models, advantage limitations potential future research directions. comprehensive will researchers knowledge necessary develop high-fidelity, robust, adaptable, flexible, accurate advanced materials.
Language: Английский
Citations
5Computer Methods in Applied Mechanics and Engineering, Journal Year: 2024, Volume and Issue: 422, P. 116789 - 116789
Published: Feb. 14, 2024
Language: Английский
Citations
4Computers and Geotechnics, Journal Year: 2025, Volume and Issue: 179, P. 107060 - 107060
Published: Jan. 9, 2025
Language: Английский
Citations
0Advanced Engineering Informatics, Journal Year: 2025, Volume and Issue: 65, P. 103306 - 103306
Published: April 7, 2025
Language: Английский
Citations
0Engineering With Computers, Journal Year: 2025, Volume and Issue: unknown
Published: April 7, 2025
Language: Английский
Citations
0Computers and Geotechnics, Journal Year: 2024, Volume and Issue: 177, P. 106907 - 106907
Published: Nov. 20, 2024
Language: Английский
Citations
2Scripta Materialia, Journal Year: 2024, Volume and Issue: 254, P. 116315 - 116315
Published: Aug. 26, 2024
Language: Английский
Citations
2Computer Methods in Applied Mechanics and Engineering, Journal Year: 2024, Volume and Issue: 428, P. 117070 - 117070
Published: June 14, 2024
Language: Английский
Citations
1Computers and Geotechnics, Journal Year: 2024, Volume and Issue: 168, P. 106133 - 106133
Published: Feb. 8, 2024
In deep geological repositories for high level nuclear waste with close canister spacings, bentonite buffers can experience temperatures higher than 100 °C. this range of extreme temperatures, phenomenological constitutive laws face limitations in capturing the thermo-hydraulic behavior bentonite, since pre-defined functional often lack generality and flexibility to capture a wide complex coupling phenomena as well effects stress state path dependency. work, neural network (DNN)-based soil–water retention curve (SWRC) is introduced integrated into Reproducing Kernel Particle Method (RKPM) conducting simulations buffer. The DNN-SWRC model incorporates temperature an additional input variable, allowing it learn relationship between suction degree saturation under general non-isothermal condition, which difficult represent using SWRC. For effective modeling tank-scale test, new axisymmetric basis functions enriched singular Dirichlet enforcement representing heater placement convective heat transfer coefficient thin-layer composite tank construction are developed. proposed method demonstrated through experiment involving cylindrical layer MX-80 exposed central heating.
Language: Английский
Citations
0