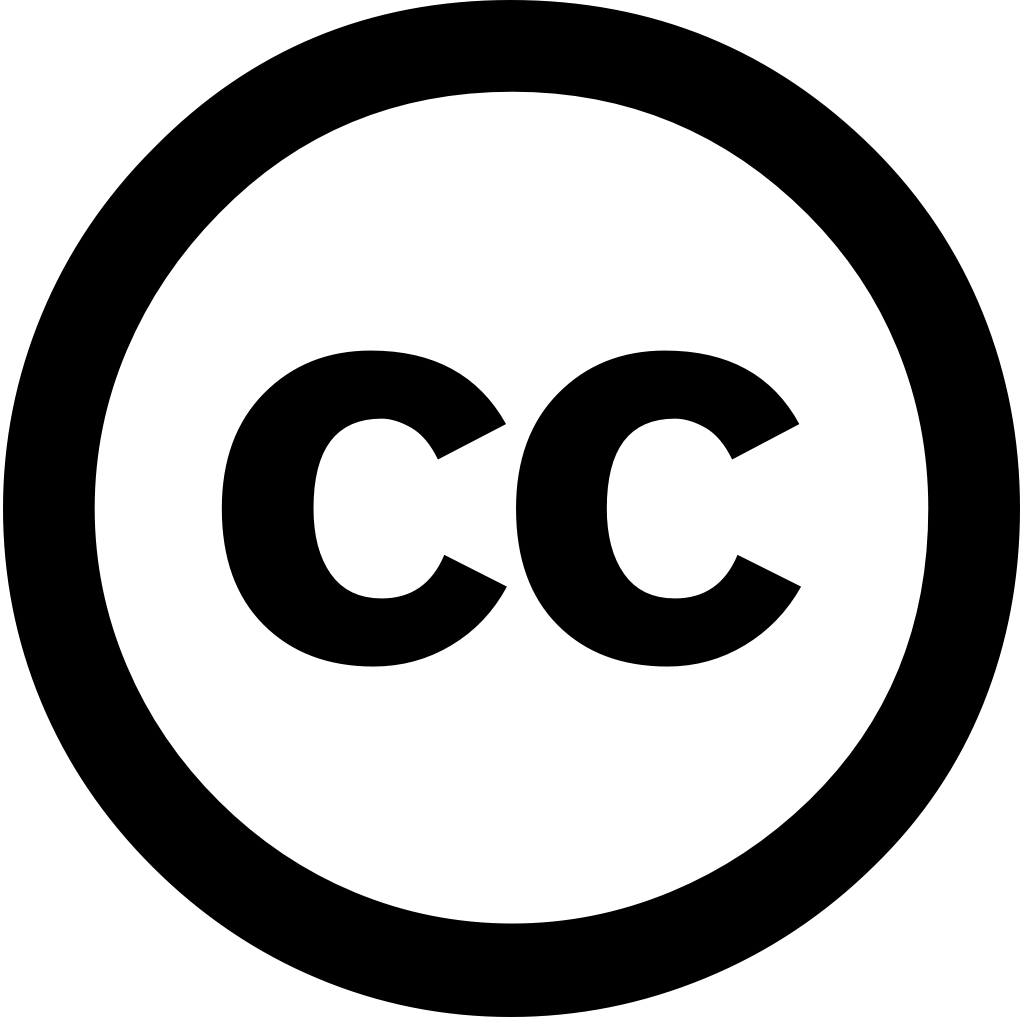
Sensors, Journal Year: 2025, Volume and Issue: 25(7), P. 2254 - 2254
Published: April 3, 2025
Intelligent fault diagnosis of bearings is crucial to the safe operation and productivity mechanical equipment, but it still faces challenge difficulty in acquiring real data practical applications. Therefore, this paper proposes a domain adaptive meta-relation network (DAMRN) achieve diagnostic knowledge transfer from laboratory-simulated faults (human-induced faults) scenario (natural by fusing meta-learning adaptation techniques. Specifically, firstly, through meta-task training, DAMRN captures task-independent generic features human-induced samples, which gives model ability adapt quickly target tasks. Secondly, strategy that complements each other with explicit alignment implicit confrontation set up effectively reduce discrepancy between natural faults. Finally, experimentally validates two cases (same-machine cross-machine) fault, outperforms methods average accuracies as high 99.62% 96.38%, respectively. The success provides viable solution for industrial applications bearing diagnosis.
Language: Английский