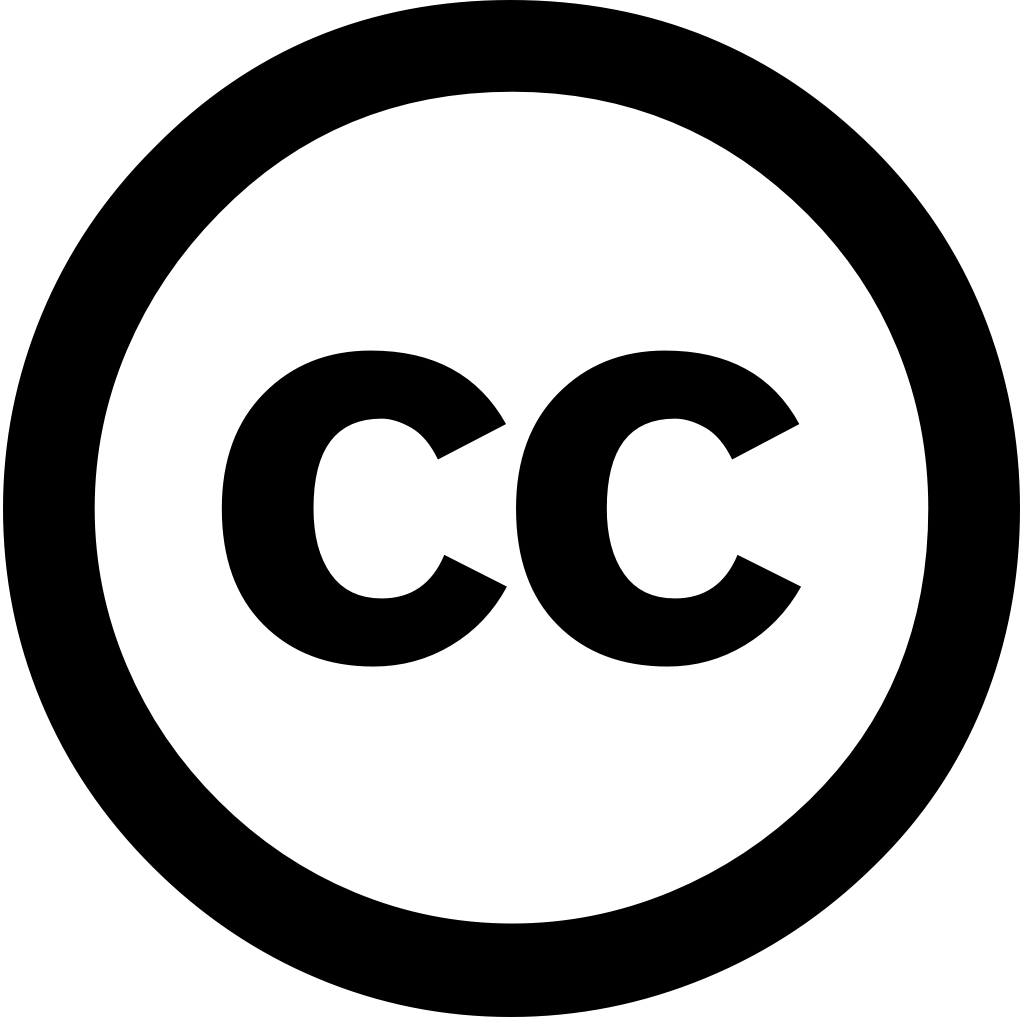
Diagnostics, Journal Year: 2025, Volume and Issue: 15(7), P. 848 - 848
Published: March 26, 2025
Background: The leading global cause of death is coronary artery disease (CAD), necessitating early and precise diagnosis. Intravascular ultrasound (IVUS) a sophisticated imaging technique that provides detailed visualization arteries. However, the methods for segmenting walls in IVUS scan into internal wall structures quantifying plaque are still evolving. This study explores use transformers attention-based models to improve diagnostic accuracy segmentation scans. Thus, objective explore application transformer scans assess their inherent biases artificial intelligence systems improving accuracy. Methods: By employing Preferred Reporting Items Systematic Reviews Meta-Analyses (PRISMA) framework, we pinpointed examined top strategies using transformer-based techniques, assessing traits, scientific soundness, clinical relevancy. Coronary thickness determined by boundaries (inner: lumen-intima outer: media-adventitia) through cross-sectional Additionally, it first investigate deep learning (DL) associated with segmentation. Finally, incorporates explainable AI (XAI) concepts DL structure Findings: Because its capacity automatically extract features at numerous scales encoders, rebuild segmented pictures via decoders, fuse variations skip connections, UNet model stands out as an efficient Conclusions: investigation underscores deficiency incentives embracing XAI pruned (PAI) models, no attaining bias-free configuration. Shifting from theoretical practical usage crucial bolstering evaluation deployment.
Language: Английский