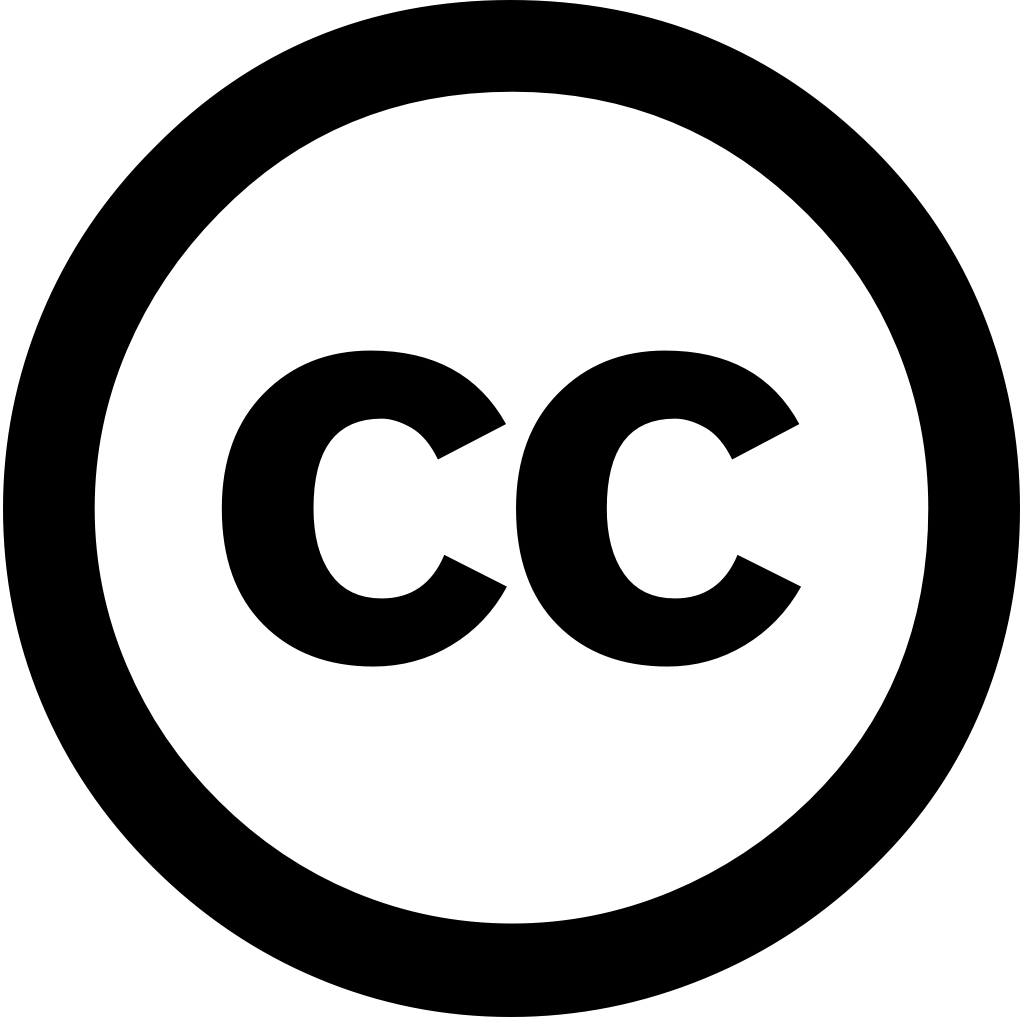
Sustainable Chemistry for the Environment, Journal Year: 2024, Volume and Issue: unknown, P. 100186 - 100186
Published: Nov. 1, 2024
Language: Английский
Sustainable Chemistry for the Environment, Journal Year: 2024, Volume and Issue: unknown, P. 100186 - 100186
Published: Nov. 1, 2024
Language: Английский
Journal of Materials Science, Journal Year: 2024, Volume and Issue: 59(31), P. 14095 - 14140
Published: July 30, 2024
Abstract Electrospun nanofibers have gained prominence as a versatile material, with applications spanning tissue engineering, drug delivery, energy storage, filtration, sensors, and textiles. Their unique properties, including high surface area, permeability, tunable porosity, low basic weight, mechanical flexibility, alongside adjustable fiber diameter distribution modifiable wettability, make them highly desirable across diverse fields. However, optimizing the properties of electrospun to meet specific requirements has proven be challenging endeavor. The electrospinning process is inherently complex influenced by numerous variables, applied voltage, polymer concentration, solution flow rate, molecular weight polymer, needle-to-collector distance. This complexity often results in variations nanofibers, making it difficult achieve desired characteristics consistently. Traditional trial-and-error approaches parameter optimization been time-consuming costly, they lack precision necessary address these challenges effectively. In recent years, convergence materials science machine learning (ML) offered transformative approach electrospinning. By harnessing power ML algorithms, scientists researchers can navigate intricate space more efficiently, bypassing need for extensive experimentation. holds potential significantly reduce time resources invested producing wide range applications. Herein, we provide an in-depth analysis current work that leverages obtain target nanofibers. examining work, explore intersection ML, shedding light on advancements, challenges, future directions. comprehensive not only highlights processes but also provides valuable insights into evolving landscape, paving way innovative precisely engineered various Graphical abstract
Language: Английский
Citations
18Construction and Building Materials, Journal Year: 2024, Volume and Issue: 438, P. 137244 - 137244
Published: July 2, 2024
Language: Английский
Citations
14Nano Research, Journal Year: 2024, Volume and Issue: unknown
Published: Sept. 7, 2024
Language: Английский
Citations
3Construction and Building Materials, Journal Year: 2025, Volume and Issue: 470, P. 140698 - 140698
Published: March 7, 2025
Language: Английский
Citations
0Sustainable Chemistry for the Environment, Journal Year: 2024, Volume and Issue: unknown, P. 100186 - 100186
Published: Nov. 1, 2024
Language: Английский
Citations
0