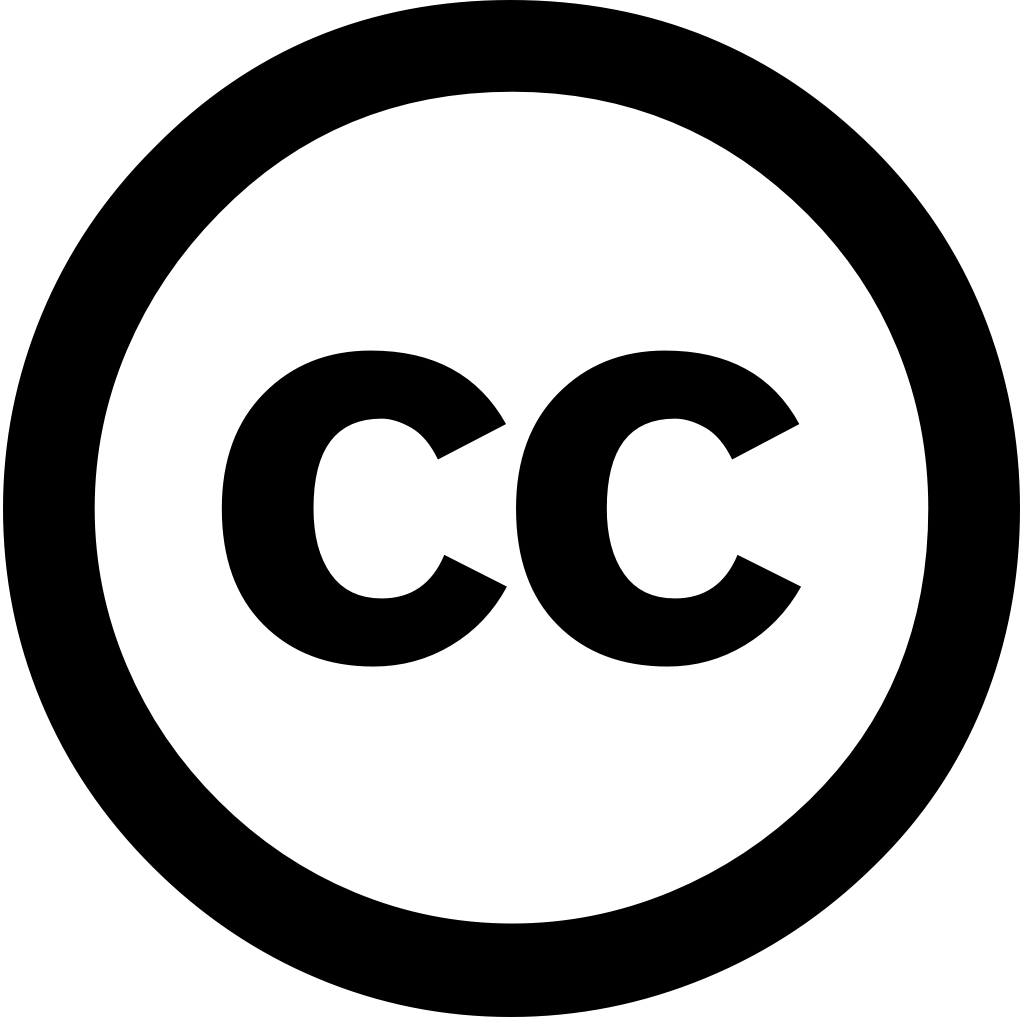
AIMS Mathematics, Journal Year: 2024, Volume and Issue: 9(12), P. 35678 - 35701
Published: Jan. 1, 2024
<p>Autonomous vehicles (AVs), particularly self-driving cars, have produced a large amount of interest in artificial intelligence (AI), intelligent transportation, and computer vision. Tracing detecting numerous targets real-time, mainly city arrangements adversarial environmental conditions, has become significant challenge for AVs. The effectiveness vehicle detection been measured as crucial stage visual surveillance or traffic monitoring. After developing driver assistance AV methods, weather conditions an essential problem. Nowadays, deep learning (DL) machine (ML) models are critical to enhancing object AVs, conditions. However, according statistical learning, conventional AI is fundamental, facing restrictions due manual feature engineering restricted flexibility adaptive environments. This study presents the explainable with fusion-based transfer on adverse autonomous (XAIFTL-AWCDAV) method. XAIFTL-AWCDAV model's main aim detect classify AVs challenging scenarios. In preprocessing stage, model utilizes non-local mean filtering (NLM) method noise reduction. Besides, performs extraction by fusing three models: EfficientNet, SqueezeNet, MobileNetv2. denoising autoencoder (DAE) technique employed Next, DAE method's hyperparameter selection uses Levy sooty tern optimization (LSTO) approach. Finally, ensure transparency predictions, integrates (XAI) techniques, utilizing SHAP visualize interpret each feature's impact decision-making process. efficiency validated comprehensive studies using benchmark dataset. Numerical results show that obtained superior value 98.90% over recent techniques.</p>
Language: Английский