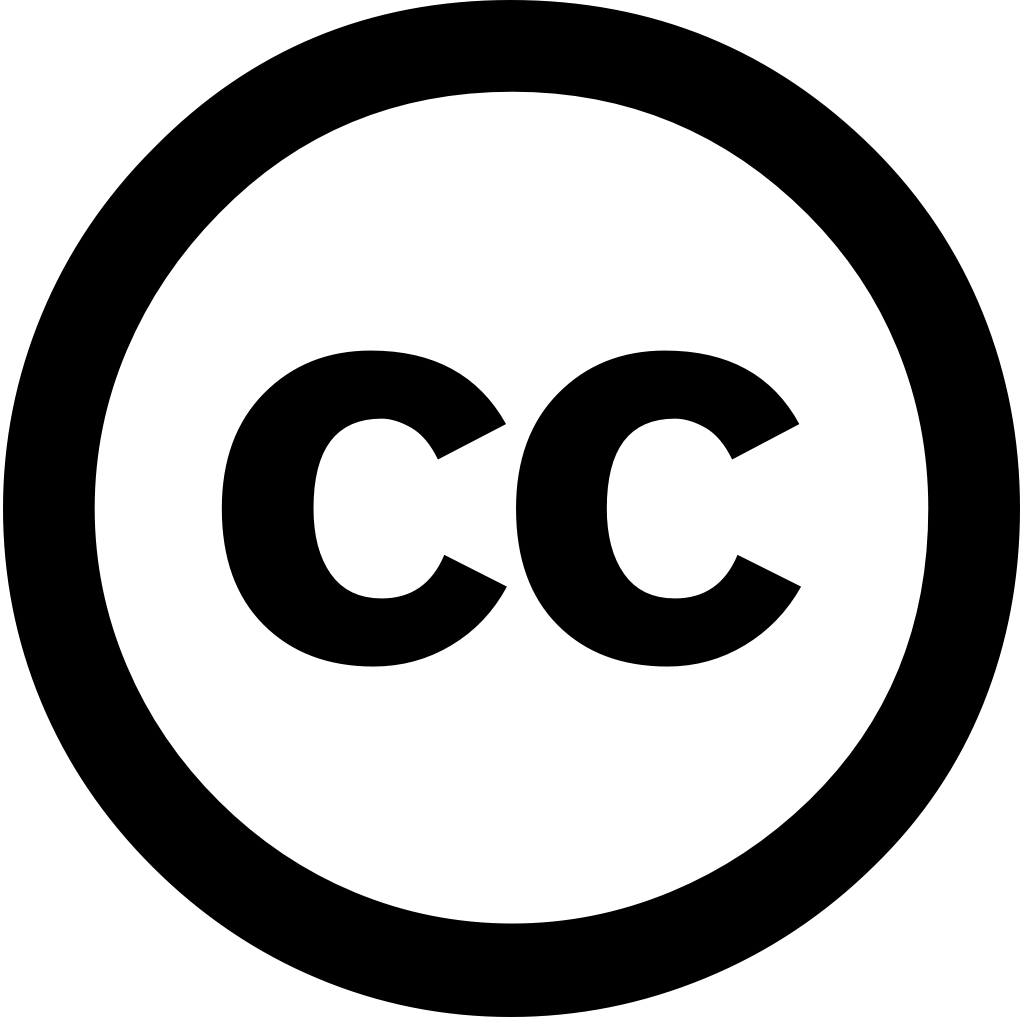
Journal of Engineering Research, Journal Year: 2025, Volume and Issue: unknown
Published: March 1, 2025
Language: Английский
Journal of Engineering Research, Journal Year: 2025, Volume and Issue: unknown
Published: March 1, 2025
Language: Английский
IEEE Access, Journal Year: 2024, Volume and Issue: 12, P. 23733 - 23750
Published: Jan. 1, 2024
The field of Natural Language Processing (NLP) is currently undergoing a revolutionary transformation driven by the power pre-trained Large Models (LLMs) based on groundbreaking Transformer architectures. As frequency and diversity cybersecurity attacks continue to rise, importance incident detection has significantly increased. IoT devices are expanding rapidly, resulting in growing need for efficient techniques autonomously identify network-based networks with both high precision minimal computational requirements. This paper presents SecurityBERT, novel architecture that leverages Bidirectional Encoder Representations from Transformers (BERT) model cyber threat networks. During training we incorporated privacy-preserving encoding technique called Privacy-Preserving Fixed-Length Encoding (PPFLE). We effectively represented network traffic data structured format combining PPFLE Byte-level Byte-Pair (BBPE) Tokenizer. Our research demonstrates SecurityBERT outperforms traditional Machine Learning (ML) Deep (DL) methods, such as Convolutional Neural Networks (CNNs) or Recurrent (RNNs), detection. Employing Edge-IIoTset dataset, our experimental analysis shows achieved an impressive 98.2% overall accuracy identifying fourteen distinct attack types, surpassing previous records set hybrid solutions GAN-Transformer-based architectures CNN-LSTM models. With inference time less than 0.15 seconds average CPU compact size just 16.7MB, ideally suited real-life suitable choice deployment resource-constrained devices.
Language: Английский
Citations
60Internet of Things and Cyber-Physical Systems, Journal Year: 2023, Volume and Issue: 4, P. 110 - 128
Published: Oct. 10, 2023
The Internet of Things (IoT) has revolutionized modern tech with interconnected smart devices. While these innovations offer unprecedented opportunities, they also introduce complex security challenges. Cybersecurity is a pivotal concern for intrusion detection systems (IDS). Deep Learning shown promise in effectively detecting and preventing cyberattacks on IoT Although IDS vital safeguarding sensitive information by identifying mitigating suspicious activities, conventional solutions grapple challenges the context. This paper delves into cutting-edge methods security, anchored Learning. We review recent advancements IoT, highlighting underlying deep learning algorithms, associated datasets, types attacks, evaluation metrics. Further, we discuss faced deploying suggest potential areas future research. survey will guide researchers industry experts adopting techniques detection.
Language: Английский
Citations
46IEEE Internet of Things Journal, Journal Year: 2024, Volume and Issue: 11(21), P. 34617 - 34638
Published: May 30, 2024
Federated learning (FL) has been gaining attention for its ability to share knowledge while maintaining user data, protecting privacy, increasing efficiency, and reducing communication overhead. Decentralized FL (DFL) is a decentralized network architecture that eliminates the need central server in contrast centralized (CFL). DFL enables direct between clients, resulting significant savings resources. In this paper, comprehensive survey profound perspective are provided DFL. First, review of methodology, challenges, variants CFL conducted, laying background Then, systematic detailed on introduced, including iteration order, protocols, topologies, paradigm proposals, temporal variability. Next, based definition DFL, several extended categorizations proposed with state-of-the-art (SOTA) technologies. Lastly, addition summarizing current challenges some possible solutions future research directions also discussed.
Language: Английский
Citations
39Computers & Security, Journal Year: 2023, Volume and Issue: 137, P. 103597 - 103597
Published: Nov. 17, 2023
Language: Английский
Citations
41Expert Systems with Applications, Journal Year: 2023, Volume and Issue: 242, P. 122861 - 122861
Published: Dec. 6, 2023
In 2016, Google proposed Federated Learning (FL) as a novel paradigm to train Machine (ML) models across the participants of federation while preserving data privacy. Since its birth, Centralized FL (CFL) has been most used approach, where central entity aggregates participants' create global one. However, CFL presents limitations such communication bottlenecks, single point failure, and reliance on server. Decentralized (DFL) addresses these issues by enabling decentralized model aggregation minimizing dependency entity. Despite advances, current platforms training DFL struggle with key managing heterogeneous network topologies, adapting process virtualized or physical deployments, using limited number metrics evaluate different scenarios for efficient implementation. To overcome challenges, this paper Fedstellar, platform designed in decentralized, semi-decentralized, centralized fashion diverse federations devices. Fedstellar allows users customizing parameters like type devices models, topology connecting them, machine deep learning algorithms, datasets each participant, among others. Additionally, it offers real-time monitoring performance. The implementation encompasses web application an interactive graphical interface, controller deploying nodes virtual devices, core deployed device, which provides logic needed train, aggregate, communicate network. effectiveness demonstrated two scenarios: deployment involving single-board Raspberry Pis detecting cyberattacks comparing various approaches controlled environment MNIST CIFAR-10 datasets. both scenarios, consistent performance adaptability, achieving F1scores 91%, 98%, 91.2% classifying CIFAR-10, respectively, reducing time 32% compared approaches.
Language: Английский
Citations
25IEEE Communications Surveys & Tutorials, Journal Year: 2023, Volume and Issue: 25(4), P. 2654 - 2713
Published: Jan. 1, 2023
The deployment of the fifth-generation (5G) wireless networks in Internet Everything (IoE) applications and future (e.g., sixth-generation (6G) networks) has raised a number operational challenges limitations, for example terms security privacy. Edge learning is an emerging approach to training models across distributed clients while ensuring data Such when integrated network infrastructures 6G) can potentially solve challenging problems such as resource management behavior prediction. However, edge (including deep learning) are known be susceptible tampering manipulation. This survey article provides holistic review extant literature focusing on learning-related vulnerabilities defenses 6G-enabled Things (IoT) systems. Existing machine approaches 6G–IoT learning-associated threats broadly categorized based modes, namely: centralized, federated, distributed. Then, we provide overview enabling technologies intelligence. We also existing research attacks against classify threat into eight categories, backdoor attacks, adversarial examples, combined poisoning Sybil byzantine inference dropping attacks. In addition, comprehensive detailed taxonomy comparative summary state-of-the-art defense methods vulnerabilities. Finally, new realized, overall prospects IoT discussed.
Language: Английский
Citations
23IEEE Access, Journal Year: 2024, Volume and Issue: 12, P. 45762 - 45772
Published: Jan. 1, 2024
The Industrial Internet of Things (IIoT) comprises a variety systems, smart devices, and an extensive range communication protocols.Hence, these systems face susceptibility to privacy security challenges, making them prime targets for malicious attacks that can result in harm the overall system.Privacy breach issues are notable concern within realm IIoT.Various intrusion detection based on machine learning (ML) deep (DL) have been introduced detect activities networks identify attacks.The existing ML DL-based models challenges when confronted with highly imbalanced training.Repetitive data network datasets inflates model performance, as has encountered much test set during training.Moreover, decrease performance include repetitions similar across various classes, where only class labels different.To overcome inherent this paper presents self-attention-based convolutional neural (SA-DCNN) designed monitoring IIoT detecting activities.Additionally, two-step cleaning method implemented eliminate redundancy training data, considering both intra-class cross-class samples.The SA-DCNN is assessed using IoTID20 Edge-IIoTset datasets.Furthermore, proposed study demonstrated through comprehensive comparison other DL models, well against relevant studies, showcasing superior efficacy model.
Language: Английский
Citations
14IEEE Communications Surveys & Tutorials, Journal Year: 2024, Volume and Issue: unknown, P. 1 - 1
Published: Jan. 1, 2024
The rapid advances in the Internet of Things (IoT) have promoted a revolution communication technology and offered various customer services. Artificial intelligence (AI) techniques been exploited to facilitate IoT operations maximize their potential modern application scenarios. In particular, convergence AI has led new networking paradigm called Intelligent (IIoT), which significantly transform businesses industrial domains. This paper presents comprehensive survey IIoT by investigating its significant applications mobile networks, as well associated security privacy issues. Specifically, we explore discuss roles wide range key domains, from smart healthcare cities transportation industries. Through such extensive discussions, investigate important issues where network attacks, confidentiality, integrity, intrusion are analyzed, along with discussion countermeasures. Privacy networks were also surveyed discussed, including data, location, model leakage. Finally, outline several challenges highlight research directions this area.
Language: Английский
Citations
14IEEE Open Journal of the Communications Society, Journal Year: 2024, Volume and Issue: 5, P. 5799 - 5856
Published: Jan. 1, 2024
Language: Английский
Citations
9Computer Networks, Journal Year: 2023, Volume and Issue: 236, P. 110015 - 110015
Published: Sept. 15, 2023
Language: Английский
Citations
22