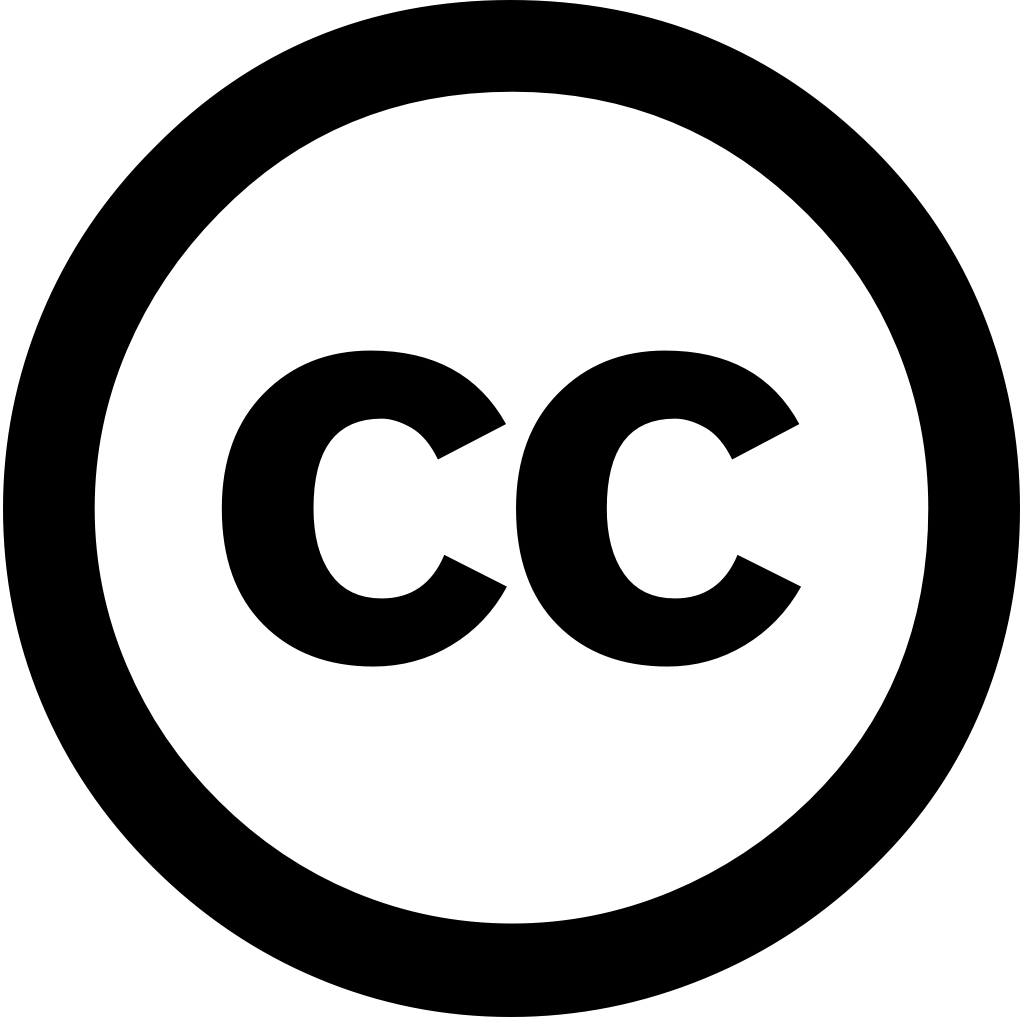
Future Internet, Journal Year: 2025, Volume and Issue: 17(4), P. 169 - 169
Published: April 11, 2025
The Internet of Things (IoT) has revolutionized modern communication systems by enabling seamless connectivity among low-power devices. However, the increasing demand for high-performance wireless networks necessitates advanced frameworks that optimize both energy efficiency (EE) and security. Cell-free massive multiple-input multiple-output (CF m-MIMO) emerged as a promising solution IoT networks, offering enhanced spectral efficiency, low-latency communication, robust connectivity. Nevertheless, balancing EE security in such remains significant challenge due to stringent power computational constraints This study employs secrecy (SEE) key performance metric evaluate trade-off between consumption secure efficiency. By jointly considering rate, our analysis provides comprehensive assessment security-aware CF m-MIMO-based networks. To enhance SEE, we introduce hybrid deep-learning (DL) framework integrates convolutional neural (CNN) long short-term memory (LSTM) joint optimization. CNN extracts spatial features, while LSTM captures temporal dependencies, more adaptive modeling dynamic patterns. Additionally, multi-objective improved biogeography-based optimization (MOIBBO) algorithm is utilized hyperparameters, ensuring an balance convergence speed model performance. Extensive simulation results demonstrate proposed MOIBBO-CNN–LSTM achieves superior SEE compared benchmark schemes. Specifically, attains gain up 38% 22% over converging significantly faster at early training epochs. Furthermore, reveal improves with AP transmit saturation point (approximately 9.5 Mb/J PAPmax=500 mW), beyond which excessive limits gains. decreases number APs increases, underscoring need selection strategies mitigate static backhaul links. These findings confirm offers effective optimizing paving way energy-efficient communications.
Language: Английский