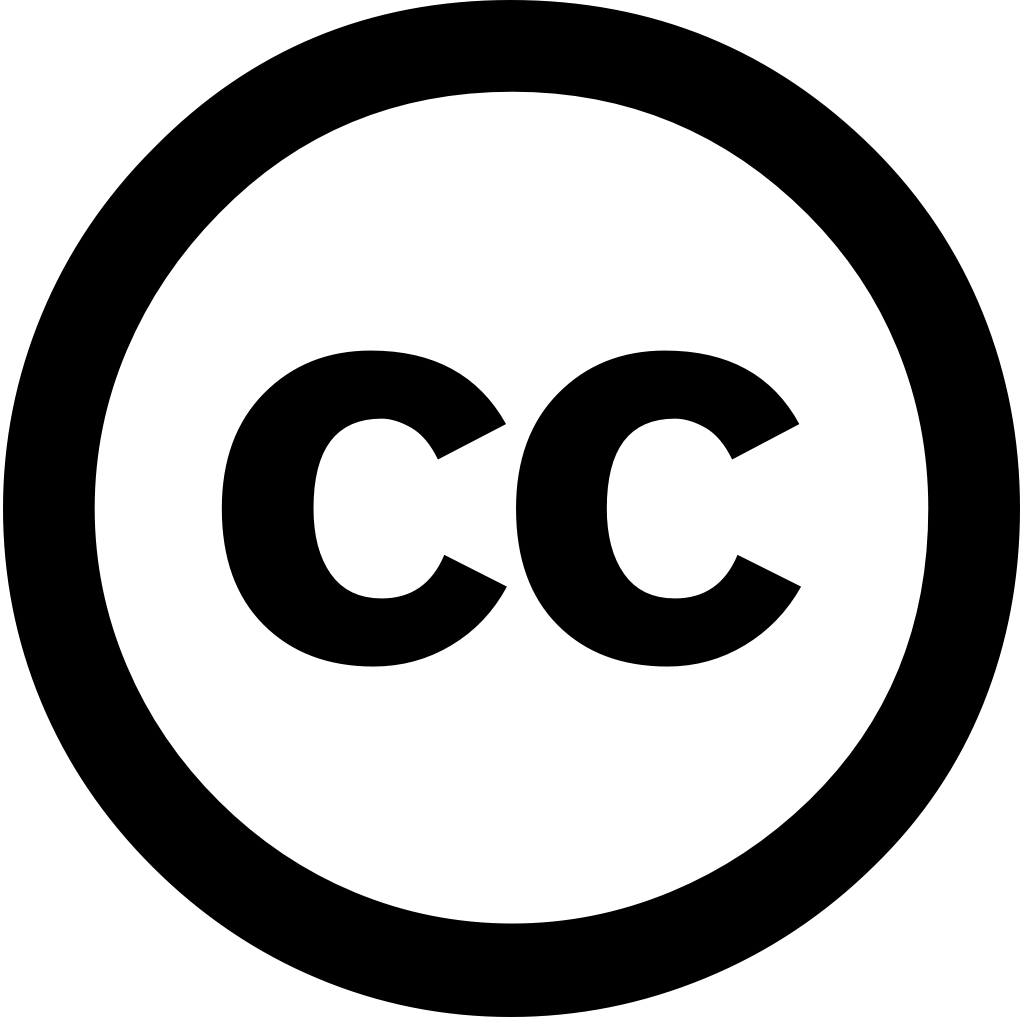
Fuel Processing Technology, Journal Year: 2024, Volume and Issue: 266, P. 108162 - 108162
Published: Nov. 20, 2024
Language: Английский
Fuel Processing Technology, Journal Year: 2024, Volume and Issue: 266, P. 108162 - 108162
Published: Nov. 20, 2024
Language: Английский
Process Safety and Environmental Protection, Journal Year: 2025, Volume and Issue: unknown, P. 106789 - 106789
Published: Jan. 1, 2025
Language: Английский
Citations
0Journal of Hazardous Toxic and Radioactive Waste, Journal Year: 2025, Volume and Issue: 29(3)
Published: April 21, 2025
Language: Английский
Citations
0International Journal of Energy Research, Journal Year: 2025, Volume and Issue: 2025(1)
Published: Jan. 1, 2025
This study proposes a deep neural network (DNN)–based regression model for predicting the excess air ratio, which is critical indicator optimizing combustion efficiency and minimizing harmful emissions in industrial systems. The chemiluminescence signals of OH ∗ radicals fuel pressure were used as input features prediction model. To evaluate effect multidimensional input, Case 1, with only radical signal single was compared 2, inputs. results showed that 2 reduced mean absolute error (MAE), relative (MRE), root squared (RMSE) by approximately 40.71%, 41.85%, 19.69%, respectively, to average rate also 2.25% lower. These demonstrate potential improving accuracy generalization ability incorporating features. Therefore, DNN models using inputs can contribute design implementation control systems optimize reduce ratio.
Language: Английский
Citations
0Energies, Journal Year: 2024, Volume and Issue: 17(18), P. 4634 - 4634
Published: Sept. 17, 2024
Expanding waste-to-energy (WtE) facilities is difficult, and with tightening incineration regulations, improvements in WtE facility operations are required to dispose of waste that increasing by an average 4.8% annually. To achieve this, intelligent combustion control (ICC) system was studied using digital technologies such as the Internet Things artificial intelligence improve operation facilities. The ICC this study composed three modules: perception, decision, control. Perception: collecting visualizing data on operating status facilities; Decision: AI propose optimal methods; Control: automatically controlling according AI-suggested optimization methods. applied “G” facility, a solid Gyeonggi province, Republic Korea, collected over six months showed high quality, low delay loss rate only 0.12%. Additionally, January 2024, used second forced draft fan induced four-day period. As result, incinerator flue gas temperature decreased 0.66%, steam flow improved 2.41%, power generation increased 3.09%, CO emissions were reduced 60.72%, NOx 7.33%. Future research will expand include automatic first time stoker.
Language: Английский
Citations
2Fuel Processing Technology, Journal Year: 2024, Volume and Issue: 266, P. 108162 - 108162
Published: Nov. 20, 2024
Language: Английский
Citations
0