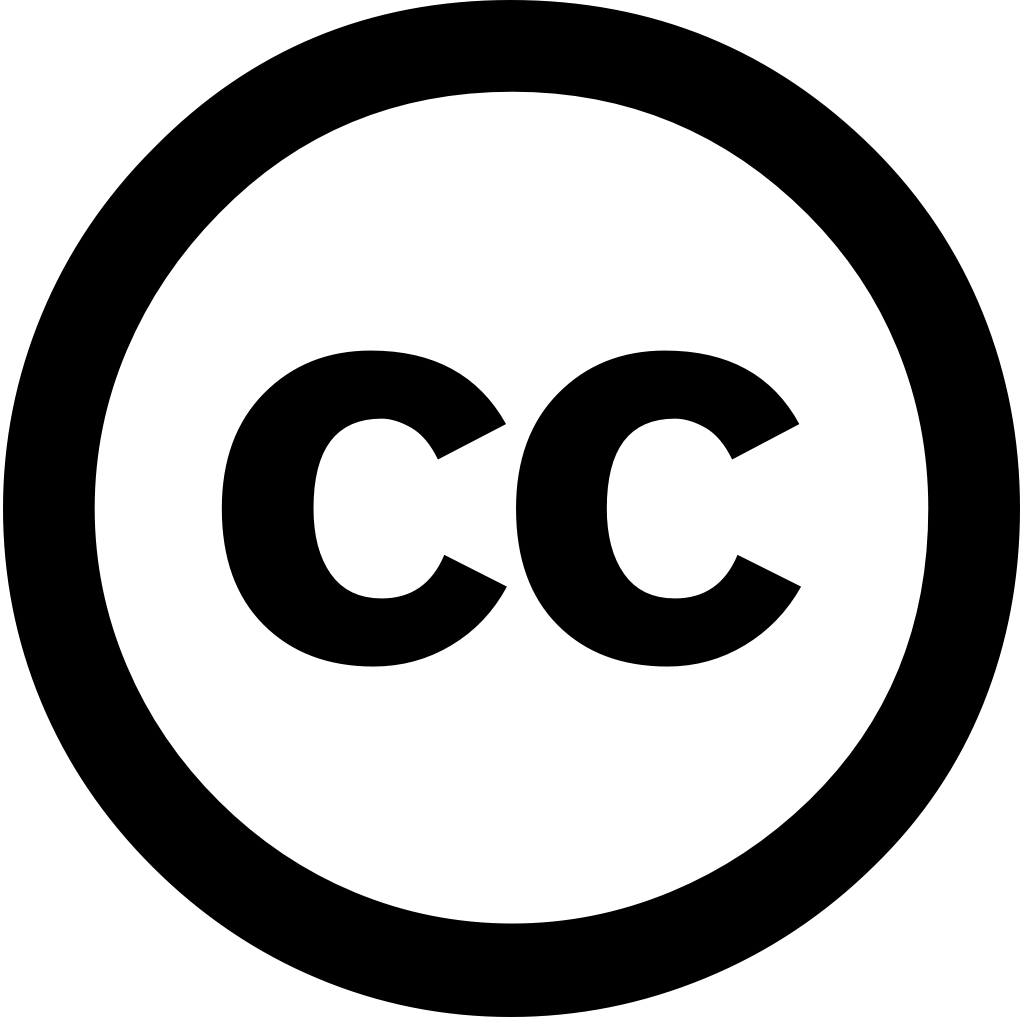
Journal of Computational Mathematics and Data Science, Journal Year: 2024, Volume and Issue: unknown, P. 100105 - 100105
Published: Oct. 1, 2024
Language: Английский
Journal of Computational Mathematics and Data Science, Journal Year: 2024, Volume and Issue: unknown, P. 100105 - 100105
Published: Oct. 1, 2024
Language: Английский
BMC Public Health, Journal Year: 2024, Volume and Issue: 24(1)
Published: Jan. 23, 2024
Abstract Background Elevated levels of executive function and physical fitness play a pivotal role in shaping future quality life. However, few studies have examined the collaborative influences mental health on academic achievement. This study aims to investigate key factors that collaboratively influence primary school students' achievement from function, fitness, demographic factors. Additionally, ensemble learning methods are employed predict achievement, their predictive performance is compared with individual learners. Methods A cluster sampling method was utilized select 353 students Huai'an, China, who underwent assessments for The recursive feature elimination cross-validation identify Ensemble models, utilizing eXtreme Gradient Boosting Random Forest algorithms, were constructed based Bagging methods. Individual learners developed using Support Vector Machine, Decision Tree, Logistic Regression, Linear Discriminant Analysis followed by establishment Stacking model. Results Our findings revealed sex, body mass index, muscle strength, cardiorespiratory inhibition, working memory, shifting influencing students. Moreover, models demonstrated superior predicting among Conclusions results suggest recognizing sex differences emphasizing simultaneous development cognition well-being can positively impact warrant further attention, as they enable an accurate early warning system
Language: Английский
Citations
8Decision Analytics Journal, Journal Year: 2024, Volume and Issue: 11, P. 100468 - 100468
Published: April 20, 2024
Digital devices are an integral component of the healthcare sector. With advancement modern technology with Artificial Intelligence (AI) and Machine Learning (ML), automated diagnosis system promising results is not a difficult task. This study aims to develop recommender (RS) for better improvement patient care by hybridizing machine learning association rules (AR) rough set theory (RST) classify acute life-threatening diseases. Initially data preprocessed using binary, on-hot vector, min–max scale remove noise. RST used feature selection deal incompleteness, inconsistency, vagueness. We have designed Associated Symptom Selection (ASS) algorithm extract mutually associated symptoms which need be further matched in existing database prediction. ASS especially helpful detecting neurodevelopmental type diseases because usually detectable standard tests, observations behavioral expressions do general testing. The experiment carried out six popular ML classifiers such as AR, Decision Tree (DT), Random Forest (RF), K-Nearest Neighbors (KNN), Linear Support Vector (LSVM), Naive Bayes (NB) on publicly available datasets. Performance was compared among different regarding accuracy, precision, recall, F1-score, J-Score value. experimental result shows that AR performs clinical accuracy 94.40%, precision 90.73%, recall 94.45%, F1-score 92.55%, J-score 95.14% autism 98.7% 98% 97.8% 97.9% 97.12% respectively.
Language: Английский
Citations
4Mathematics, Journal Year: 2024, Volume and Issue: 12(11), P. 1648 - 1648
Published: May 24, 2024
Autism Spectrum Disorder (ASD) presents significant diagnostic challenges due to its complex, heterogeneous nature. This study explores a novel approach enhance the accuracy and reliability of ASD diagnosis by integrating resting-state functional magnetic resonance imaging with demographic data (age, gender, IQ). is based on improving spectral graph convolutional neural network (GCN). It introduces multi-view attention fusion module extract useful information from different views. The graph’s edges are informed data, wherein an edge-building computes weights grounded in information, thereby bolstering inter-subject correlation. To tackle oversmoothing neighborhood explosion inherent deep GCNs, this DropEdge regularization residual connections, thus augmenting feature diversity model generalization. proposed method trained evaluated ABIDE-I ABIDE-II datasets. experimental results underscore potential multimodal advance capabilities GCNs for ASD.
Language: Английский
Citations
1Psychiatry Research Neuroimaging, Journal Year: 2024, Volume and Issue: 345, P. 111901 - 111901
Published: Sept. 17, 2024
Language: Английский
Citations
1Journal of Computational Mathematics and Data Science, Journal Year: 2024, Volume and Issue: unknown, P. 100105 - 100105
Published: Oct. 1, 2024
Language: Английский
Citations
0