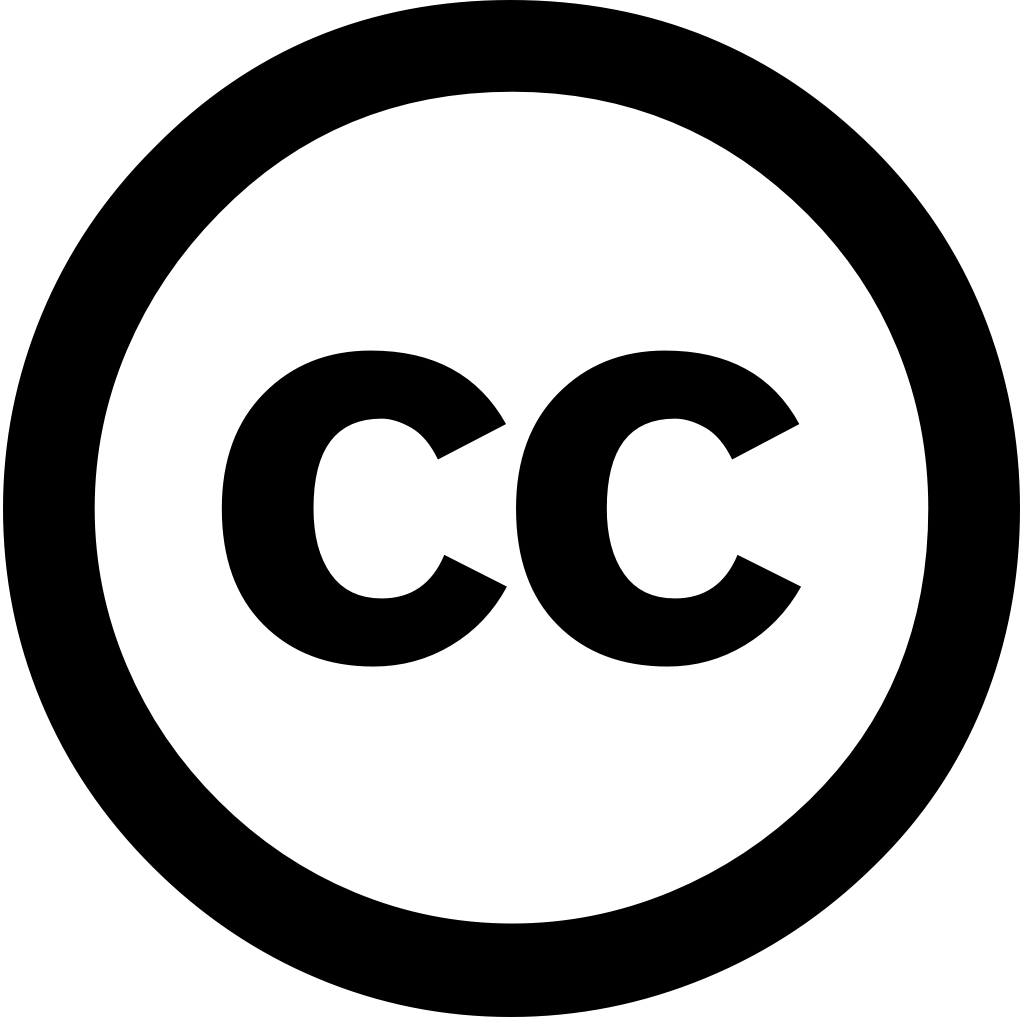
Scientific Reports, Journal Year: 2025, Volume and Issue: 15(1)
Published: April 12, 2025
Language: Английский
Scientific Reports, Journal Year: 2025, Volume and Issue: 15(1)
Published: April 12, 2025
Language: Английский
Decision Analytics Journal, Journal Year: 2024, Volume and Issue: 12, P. 100499 - 100499
Published: July 2, 2024
Traditional deep learning models are often considered "black boxes" due to their lack of interpretability, which limits therapeutic use despite success in classification tasks. This study aims improve the interpretability diagnoses for COVID-19, pneumonia, and tuberculosis from X-ray images using an enhanced DenseNet201 model within a transfer framework. We incorporated Explainable Artificial Intelligence (XAI) techniques, including SHAP, LIME, Grad-CAM, Grad-CAM++, make model's decisions more understandable. To enhance image clarity detail, we applied preprocessing methods such as Denoising Autoencoder, Contrast Limited Adaptive Histogram Equalization (CLAHE), Gamma Correction. An ablation was conducted identify optimal parameters proposed approach. Our performance compared with other learning-based like EfficientNetB0, InceptionV3, LeNet evaluation metrics. The that included data augmentation techniques achieved best results, accuracy 99.20%, precision recall 99%. demonstrates critical role improving performance. SHAP LIME provided significant insights into decision-making process, while Grad-CAM Grad-CAM++ highlighted specific features regions influencing classifications. These transparency trust AI-assisted diagnoses. Finally, developed Android-based system most effective support medical specialists process.
Language: Английский
Citations
9International Journal of Computational and Experimental Science and Engineering, Journal Year: 2024, Volume and Issue: 10(4)
Published: Oct. 16, 2024
A convolutional neural network (CNN) architecture, NutriFoodNet, enhanced through Particle Swarm Optimization (PSO) is suggested in this paper to optimize data augmentation parameters and key hyperparameters, specifically designed for food image recognition. Accurate classification plays a vital function various applications, including nutrition management, dietary assessment, healthcare, as it aids the automated recognition analysis of items from images. The implementation aimed improve accuracy on Food101 dataset. Initially, NutriFoodNet model achieved an 97.3%. By applying PSO, model's performance was further refined, resulting increased 98.5%. This optimized system benchmarked against state-of-the-art architectures, ResNet-18, ResNet-50, Inception V3, showcasing its exceptional performance. proposed highlights efficiency PSO fine-tuning CNN leading significant improvements tasks. advancement underscores potential systems contributing better monitoring healthcare outcomes.
Language: Английский
Citations
9Intelligent Systems with Applications, Journal Year: 2024, Volume and Issue: 23, P. 200422 - 200422
Published: Aug. 4, 2024
Language: Английский
Citations
8Engineering Applications of Computational Fluid Mechanics, Journal Year: 2025, Volume and Issue: 19(1)
Published: Jan. 8, 2025
Predicting water levels in glacier-fed lakes is vital for resource management, flood forecasting, and ecological balance. This study examines the predictive capacity of multiple climate factors affecting Blue Moon Lake Valley, fed by Baishui River glacier on Yulong Snow Mountain. The introduces a novel quad-meta (QM) ensemble model that integrates outputs from four machine learning models – extreme gradient boosting (XGB), random forest (RF), (GBM), decision tree (DT) through meta-learning to improve prediction accuracy under complex environmental conditions. High-frequency depth data, recorded every five minutes using an RBR logger, alongside variables such as temperature, wind speed, humidity, evaporation, solar radiation, rainfall, were analyzed. Temperature was identified most significant factor influencing levels, with importance score 15.69, followed atmospheric pressure (14.08) radiation (12.89), which impacted surface conditions evaporation. Relative humidity (10.24) speed (8.71) influenced lake stability mixing. QM outperformed individual models, achieved RMSE values 0.003 m (climate data) 0.001 (water data), R2 0.994 0.999, respectively. In comparison, XGB GBM exhibited higher lower scores. RF struggled 0.008 0.962, while DT performed better (RMSE: 0.006 but remained inferior proposed model. These findings demonstrate robustness approach handling particularly where fall short. highlights potential enhanced systems, recommending future research directions incorporate deep long-term forecasting expand capabilities global scale.
Language: Английский
Citations
1Metals, Journal Year: 2025, Volume and Issue: 15(1), P. 62 - 62
Published: Jan. 12, 2025
The scrap-based electric arc furnace process is expected to capture a significant share of the steel market in future due its potential for reducing environmental impacts through recycling. However, managing impurities, particularly phosphorus, remains challenge. This study aims develop machine learning model estimate phosphorus content at end based on input parameters. Data were collected over one year from plant, focusing parameters such as chemical composition and weight scrap, volume oxygen injected, injected lime, duration. After preprocessing data, several models evaluated, with artificial neural network (ANN) emerging most effective. Adam optimizer non-linear sigmoid activation function employed. best ANN included four hidden layers 448 neurons. was trained 500 epochs batch size 50. achieves mean square error (MSE) 0.000016, root (RMSE) 0.0049998, coefficient determination (R2) 99.96%, correlation (r) 99.98%. Notably, tested 200 unseen data points achieved 100% hit rate predicting within ±0.001 wt% (±10 ppm). These results demonstrate that optimized offers accurate predictions final content.
Language: Английский
Citations
1Scientific Reports, Journal Year: 2025, Volume and Issue: 15(1)
Published: Jan. 29, 2025
Attention mechanisms such as the Convolutional Block Module (CBAM) can help emphasize and refine most relevant feature maps color, texture, spots, wrinkle variations for avocado ripeness classification. However, CBAM lacks global context awareness, which may prevent it from capturing long-range dependencies or patterns relationships between distant regions in image. Further, more complex neural networks improve model performance but at cost of increasing number layers train parameters, not be suitable resource constrained devices. This paper presents Hybrid Neural Network (HACNN) classifying on It aims to perform local enhancement capture relationships, leading a comprehensive extraction by combining attention modules models. The proposed HACNN combines transfer learning with hybrid mechanisms, including Spatial, Channel, Self-Attention Modules, effectively intricate features fourteen thousand images. Extensive experiments demonstrate that EfficienctNet-B3 significantly outperforms conventional models regarding accuracy 96.18%, 92.64%, 91.25% train, validation, test models, respectively. In addition, this consumed 59.81 MB memory an average inference time 280.67 ms TensorFlow Lite smartphone. Although ShuffleNetV1 (1.0x) consumes least resources, its testing is only 82.89%, insufficient practical applications. Thus, MobileNetV3 Large exciting option has 91.04%, usage 26.52 MB, 86.94 These findings indicated method enhances classification ensures feasibility implementation low-resource environments.
Language: Английский
Citations
1Radiography, Journal Year: 2025, Volume and Issue: 31(2), P. 102878 - 102878
Published: Jan. 31, 2025
Language: Английский
Citations
1Applied Energy, Journal Year: 2024, Volume and Issue: 377, P. 124398 - 124398
Published: Sept. 10, 2024
Language: Английский
Citations
4Peer-to-Peer Networking and Applications, Journal Year: 2025, Volume and Issue: 18(2)
Published: Jan. 20, 2025
Language: Английский
Citations
0Engineering Applications of Artificial Intelligence, Journal Year: 2025, Volume and Issue: 143, P. 110046 - 110046
Published: Jan. 22, 2025
Language: Английский
Citations
0