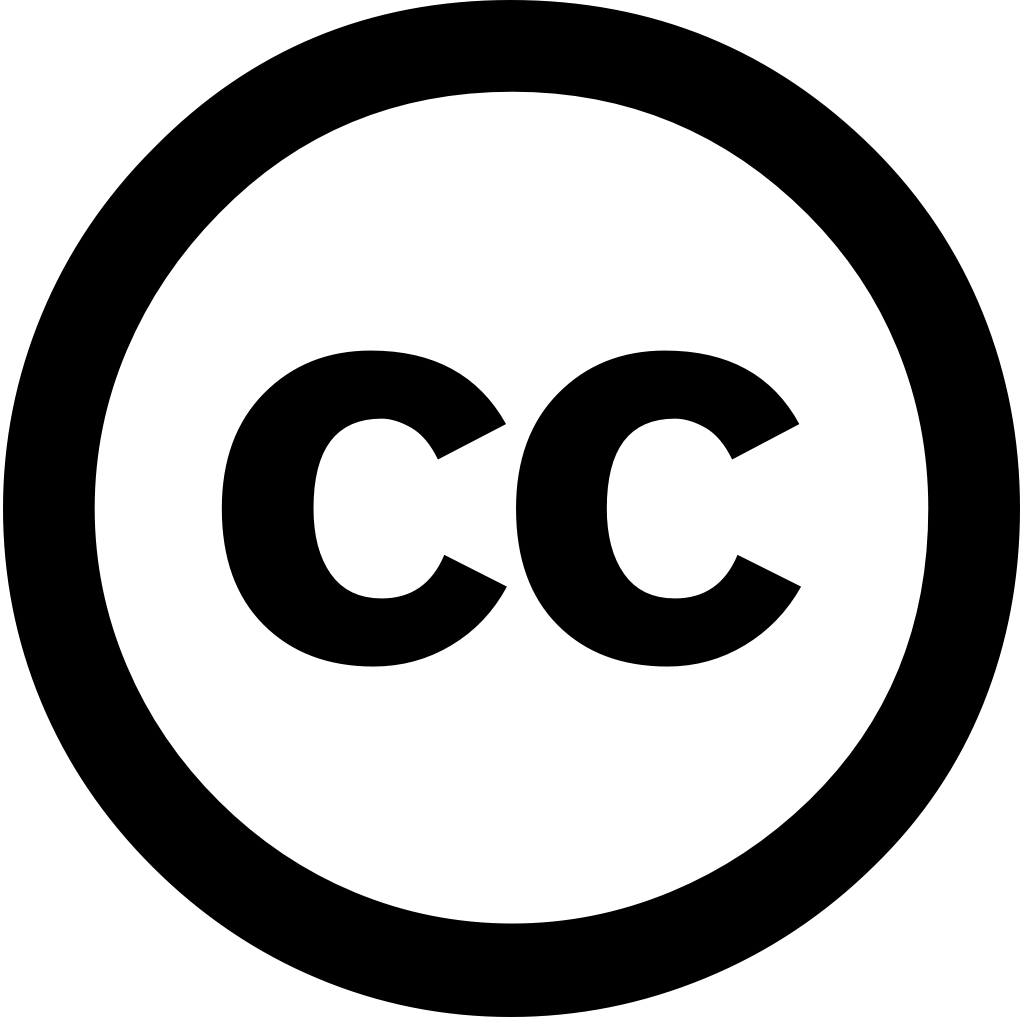
Deleted Journal, Journal Year: 2025, Volume and Issue: 7(5)
Published: May 8, 2025
Language: Английский
Deleted Journal, Journal Year: 2025, Volume and Issue: 7(5)
Published: May 8, 2025
Language: Английский
Journal of Water Process Engineering, Journal Year: 2024, Volume and Issue: 58, P. 104789 - 104789
Published: Jan. 17, 2024
Language: Английский
Citations
69Results in Engineering, Journal Year: 2024, Volume and Issue: 21, P. 101837 - 101837
Published: Feb. 6, 2024
Contemporary infrastructure requires structural elements with enhanced mechanical strength and durability. Integrating nanomaterials into concrete is a promising solution to improve However, the intricacies of such nanoscale cementitious composites are highly complex. Traditional regression models encounter limitations in capturing these intricate compositions provide accurate reliable estimations. This study focuses on developing robust prediction for compressive (CS) graphene nanoparticle-reinforced (GrNCC) through machine learning (ML) algorithms. Three ML models, bagging regressor (BR), decision tree (DT), AdaBoost (AR), were employed predict CS based comprehensive dataset 172 experimental values. Seven input parameters, including graphite nanoparticle (GrN) diameter, water-to-cement ratio (wc), GrN content (GC), ultrasonication (US), sand (SC), curing age (CA), thickness (GT), considered. The trained 70 % data, remaining 30 data was used testing models. Statistical metrics as mean absolute error (MAE), root square (RMSE) correlation coefficient (R) assess predictive accuracy DT AR demonstrated exceptional accuracy, yielding high coefficients 0.983 0.979 training, 0.873 0.822 testing, respectively. Shapley Additive exPlanation (SHAP) analysis highlighted influential role positively impacting CS, while an increased (w/c) negatively affected CS. showcases efficacy techniques accurately predicting nanoparticle-modified concrete, offering swift cost-effective approach assessing nanomaterial impact reducing reliance time-consuming expensive experiments.
Language: Английский
Citations
28Results in Engineering, Journal Year: 2024, Volume and Issue: 23, P. 102637 - 102637
Published: July 29, 2024
Airborne contaminants pose significant environmental and health challenges. Titanium dioxide (TiO2) has emerged as a leading photocatalyst in the degradation of air compared to other photocatalysts due its inherent inertness, cost-effectiveness, photostability. To assess effectiveness, laboratory examinations are frequently employed measure photocatalytic rate TiO2. However, this approach involves time-consuming requirements, labor-intensive tasks, high costs. In literature, ensemble or standalone models commonly used for assessing performance TiO2 water contaminants. Nonetheless, application metaheuristic hybrid potential be more effective predictive accuracy efficiency. Accordingly, research utilized machine learning (ML) algorithms estimate photo-degradation constants organic pollutants using nanoparticles exposure ultraviolet light. Six metaheuristics optimization algorithms, namely, nuclear reaction (NRO), differential evolution algorithm (DEA), human felicity (HFA), lightning search (LSA), Harris hawks (HHA), tunicate swarm (TSA) were combined with random forest (RF) technique establish models. A database 200 data points was acquired from experimental studies model training testing. Furthermore, multiple statistical indicators 10-fold cross-validation examine established model's robustness. The TSA-RF demonstrated superior prediction among six suggested models, achieving an impressive correlation (R) 0.90 lower root mean square error (RMSE) 0.25. contrast, HFA-RF, HHA-RF, NRO-RF exhibited slightly R-value 0.88, RMSE scores 0.32. DEA-RF LSA-RF while effective, showed marginally 0.85, values 0.45 0.44, respectively. Moreover, SHapley Additive exPlanation (SHAP) results indicated that rates through photocatalysis most notably influenced by factors such reactor sizes, dosage, humidity, intensity.
Language: Английский
Citations
21Results in Engineering, Journal Year: 2025, Volume and Issue: unknown, P. 103909 - 103909
Published: Jan. 1, 2025
Language: Английский
Citations
2Developments in the Built Environment, Journal Year: 2024, Volume and Issue: 17, P. 100378 - 100378
Published: Feb. 23, 2024
This paper reviews recent developments and proposes perspectives for future research on three-dimensional printing concrete (3DPC). review originally analyses the 3DP applications combined with types that are classified into three groups: functional concrete, sustainable special concrete. The technique shows different effects due to various modification methods (e.g., nano-additive, fibre addition, chemical reagent) challenging requirements anisotropy exploit defect). Summarily, oriented of 3DPC is a double-edged sword, asking optimal structural design engineered cementitious composite (ECC), ultra-high-performance (UHPC), most fibre-improved not propitious all types, such as foam because additional pressure in process poses huge disadvantage stability. also protentional from view features, which represents contribution advanced technology development direction.
Language: Английский
Citations
16Scientific Reports, Journal Year: 2024, Volume and Issue: 14(1)
Published: June 13, 2024
Abstract The escalation of global urbanization and industrial expansion has resulted in an increase the emission harmful substances into atmosphere. Evaluating effectiveness titanium dioxide (TiO 2 ) photocatalytic degradation through traditional methods is resource-intensive complex due to detailed photocatalyst structures wide range contaminants. Therefore this study, recent advancements machine learning (ML) are used offer data-driven approach using thirteen techniques namely XG Boost (XGB), decision tree (DT), lasso Regression (LR2), support vector regression (SVR), adaBoost (AB), voting Regressor (VR), CatBoost (CB), K-Nearest Neighbors (KNN), gradient boost (GB), random Forest (RF), artificial neural network (ANN), ridge (RR), linear (LR1) address problem estimation TiO rate air models developed literature data different methodical tools evaluate ML models. XGB, DT LR2 have high R values 0.93, 0.926 training 0.936, 0.924 test phase. While ANN, RR LR lowest 0.70, 0.56 0.40 0.62, 0.63 0.31 phase respectively. low MAE RMSE 0.450 min -1 /cm , 0.494 0.49 for 0.263 0.285 0.29 stage. DT, 93% percent errors within 20% error XGB 92% 94% with remained highest performing most robust effective predictions. Feature importances reveal role input parameters prediction made by Dosage, humidity, UV light intensity remain important experimental factors. This study will impact positively providing efficient estimate contaminants .
Language: Английский
Citations
13Case Studies in Construction Materials, Journal Year: 2024, Volume and Issue: 20, P. e03018 - e03018
Published: March 2, 2024
Modern infrastructure requirements necessitate structural components with improved durability and strength properties. The incorporation of nanomaterials (NMs) into concrete emerges as a viable strategy to enhance both the concrete. Nevertheless, complexities inherent in these nanoscale cementitious composites are notably intricate. Traditional regression models face constraints comprehensively capturing intricate compositions. Thus, posing challenges delivering precise dependable estimations. Therefore, current study utilized three machine learning (ML) methods, including artificial neural network (ANN), gene expression programming (GEP), adaptive neuro-fuzzy inference system (ANFIS), conjunction experimental investigation effect integration graphene nanoplatelets (GNPs) on electrical resistivity (ER) compressive (CS) containing GNPs. Concrete GNPs demonstrated an fractional change (FCR) strength. measures depict that enhancement was notable at GNP concentrations 0.05% 0.1%, showcasing increases 13.23% 16.58%, respectively. Simultaneously, highest observed FCR reached -12.19% -13%, prediction efficacy proved be outstanding forecasting characteristics For CS, GEP, ANN, ANFIS impressive correlation coefficient (R) values 0.974, 0.963, 0.954, resistivity, exhibited high R-values 0.999, 0.995, 0.987, comparative analysis revealed GEP model delivered predictions for ER CS. mean absolute error (MAE) GEP-CS 14.51% reduction compared ANN-CS substantial 48.15% improvement over ANFIS-CS model. Similarly, displayed MAE 38.14% lower Moreover, GEP-ER 56.80% 82.47% Shapley Additive explanation (SHAP) provided curing age SHAP score. indicating its predominant contribution CS prediction. In predicting ER, content score, signifying estimation. This highlights ML's accuracy properties nanoplatelets, offering fast cost-effective alternative time-consuming experiments.
Language: Английский
Citations
12Coatings, Journal Year: 2024, Volume and Issue: 14(4), P. 386 - 386
Published: March 26, 2024
Carbonation is one of the critical issues affecting durability reinforced concrete. Evaluating depth concrete carbonation great significance for ensuring quality and safety construction projects. In recent years, various prediction algorithms have been developed evaluating depth. This article provides a detailed overview existing models According to data processing methods used in model, can be divided into mathematical curve machine learning models. The further following categories: artificial neural network decision tree support vector combined basic idea model directly establish relationship between age by using certain function curves. advantage that only small amount experimental needed fitting, which very convenient engineering applications. limitation it consider influence some factors on concrete, accuracy cannot guaranteed. predict many considered at same time. When there are sufficient data, trained give more accurate results than model. main defect needs lot as training samples, so not A future research direction may combine with evaluate accurately.
Language: Английский
Citations
10Earth Science Informatics, Journal Year: 2025, Volume and Issue: 18(1)
Published: Jan. 1, 2025
Abstract
Soilcrete
is
an
innovative
construction
material
made
by
combining
naturally
occurring
earth
materials
with
cement.
It
can
be
effectively
used
in
areas
where
other
are
not
readily
available
due
to
financial
or
environmental
reasons
since
soilcrete
from
natural
clay.
also
help
cut
down
the
greenhouse
gas
emissions
industry
encouraging
use
of
resources
that
locally
available.
Thus,
it
imperative
reliably
predict
different
properties
accurate
determination
these
crucial
for
widespread
materials.
However,
laboratory
subjected
significant
time
and
resource
constraints.
As
a
result,
this
research
was
undertaken
provide
empirical
prediction
models
density,
shrinkage,
strain
mixes
using
two
machine
learning
algorithms:
Gene
Expression
Programming
(GEP)
Extreme
Gradient
Boosting
(XGB).
The
analysis
revealed
XGB-based
predictions
correlated
more
real-life
values
than
GEP
having
training
$${\text{R}}^{2}=0.999$$
Language: Английский
Citations
1Heliyon, Journal Year: 2024, Volume and Issue: 10(17), P. e36841 - e36841
Published: Aug. 27, 2024
Language: Английский
Citations
8