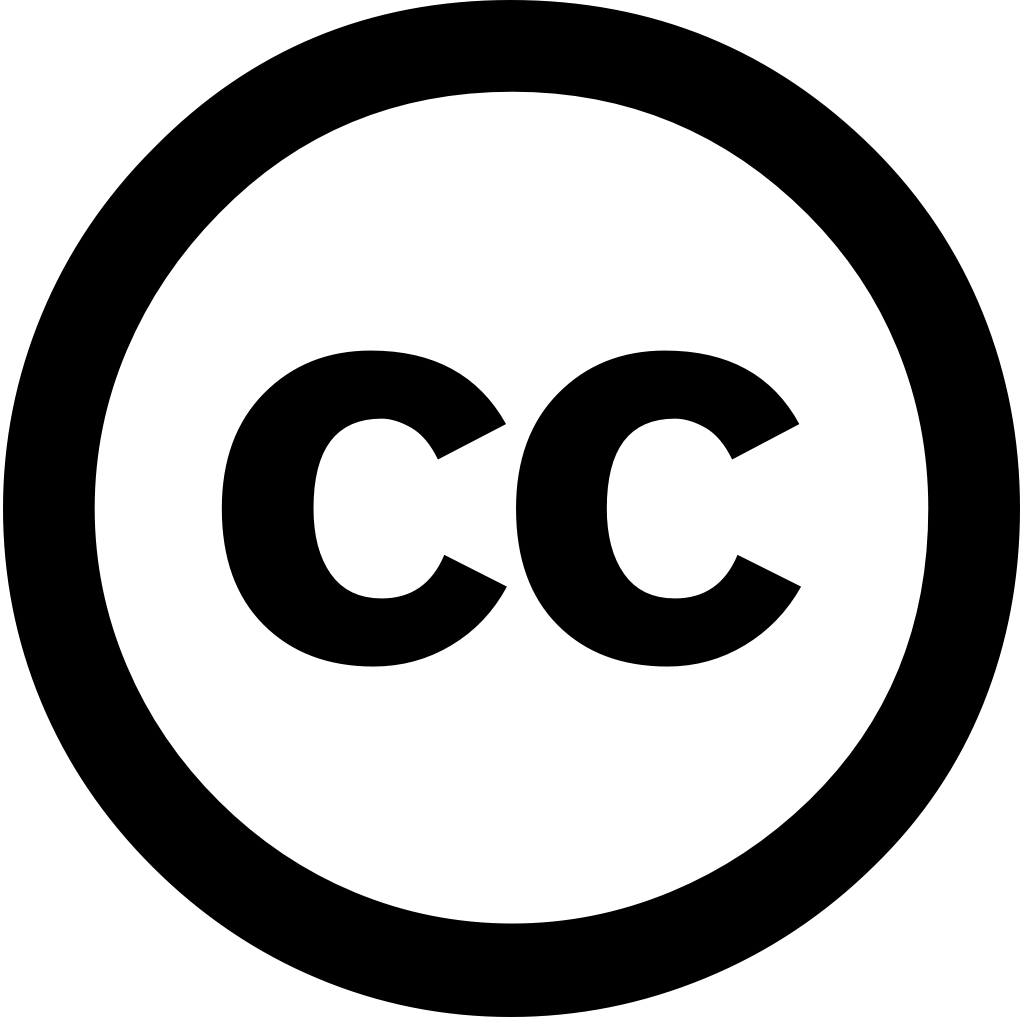
Deleted Journal, Journal Year: 2025, Volume and Issue: 7(5)
Published: May 8, 2025
Language: Английский
Deleted Journal, Journal Year: 2025, Volume and Issue: 7(5)
Published: May 8, 2025
Language: Английский
Scientific Reports, Journal Year: 2024, Volume and Issue: 14(1)
Published: June 20, 2024
Abstract Graphene nanoplatelets (GrNs) emerge as promising conductive fillers to significantly enhance the electrical conductivity and strength of cementitious composites, contributing development highly efficient composites advancement non-destructive structural health monitoring techniques. However, complexities involved in these nanoscale are markedly intricate. Conventional regression models encounter limitations fully understanding intricate compositions. Thus, current study employed four machine learning (ML) methods such decision tree (DT), categorical boosting (CatBoost), adaptive neuro-fuzzy inference system (ANFIS), light gradient (LightGBM) establish strong prediction for compressive (CS) graphene nanoplatelets-based materials. An extensive dataset containing 172 data points was gathered from published literature model development. The majority portion (70%) database utilized training while 30% used validating efficacy on unseen data. Different metrics were assess performance established ML models. In addition, SHapley Additve explanation (SHAP) interpretability. DT, CatBoost, LightGBM, ANFIS exhibited excellent with R-values 0.8708, 0.9999, 0.9043, 0.8662, respectively. While all suggested demonstrated acceptable accuracy predicting strength, CatBoost exceptional efficiency. Furthermore, SHAP analysis provided that thickness GrN plays a pivotal role GrNCC, influencing CS consequently exhibiting highest value + 9.39. diameter GrN, curing age, w/c ratio also prominent features estimating This research underscores accurately forecasting characteristics concrete reinforced nanoplatelets, providing swift economical substitute laborious experimental procedures. It is improve generalization study, more inputs increased datasets should be considered future studies.
Language: Английский
Citations
7Scientific Reports, Journal Year: 2024, Volume and Issue: 14(1)
Published: July 27, 2024
Abstract The utilization of Self-compacting Concrete (SCC) has escalated worldwide due to its superior properties in comparison normal concrete such as compaction without vibration, increased flowability and segregation resistance. Various other desirable like ductile behaviour, strain capacity tensile strength etc. can be imparted SCC by incorporation fibres. Thus, this study presents a novel approach predict 28-day compressive (C–S) FR-SCC using Gene Expression Programming (GEP) Multi (MEP) for fostering widespread use the industry. For purpose, dataset had been compiled from internationally published literature having six input parameters including water-to-cement ratio, silica fume, fine aggregate, coarse fibre, superplasticizer. predictive abilities developed algorithms were assessed error metrices mean absolute (MAE), a20-index, objective function (OF) MEP GEP models indicated that gave simple equation lesser errors than MEP. OF value was 0.029 compared 0.031 sensitivity analysis performed on model. also checked some external validation checks which verified equations used forecast practical uses.
Language: Английский
Citations
5Journal of Water Process Engineering, Journal Year: 2024, Volume and Issue: 66, P. 105937 - 105937
Published: Aug. 19, 2024
Language: Английский
Citations
5Sustainable Chemistry and Pharmacy, Journal Year: 2024, Volume and Issue: 42, P. 101763 - 101763
Published: Sept. 3, 2024
Language: Английский
Citations
5Case Studies in Construction Materials, Journal Year: 2024, Volume and Issue: 22, P. e04112 - e04112
Published: Dec. 11, 2024
Language: Английский
Citations
5Scientific Reports, Journal Year: 2025, Volume and Issue: 15(1)
Published: Feb. 10, 2025
The increasing demand for sustainable construction materials has led to the incorporation of Palm Oil Fuel Ash (POFA) into concrete reduce cement consumption and lower CO₂ emissions. However, predicting compressive strength (CS) POFA-based remains challenging due variability input factors. This study addresses this issue by applying advanced machine learning models forecast CS POFA-incorporated concrete. A dataset 407 samples was collected, including six parameters: content, POFA dosage, water-to-binder ratio, aggregate superplasticizer curing age. divided 70% training 30% testing. evaluated include Hybrid XGB-LGBM, ANN, Bagging, LSSVM, GEP, XGB LGBM. performance these assessed using key metrics, coefficient determination (R2), root mean square error (RMSE), normalized means (NRMSE), absolute (MAE) Willmott index (d). XGB-LGBM model achieved maximum R2 0.976 lowest RMSE, demonstrating superior accuracy, followed ANN with an 0.968. SHAP analysis further validated identifying most impactful factors, ratio emerging as influential. These predictive offer industry a reliable framework evaluating concrete, reducing need extensive experimental testing, promoting development more eco-friendly, cost-effective building materials.
Language: Английский
Citations
0Archives of Computational Methods in Engineering, Journal Year: 2025, Volume and Issue: unknown
Published: March 12, 2025
Language: Английский
Citations
0Ain Shams Engineering Journal, Journal Year: 2024, Volume and Issue: 15(10), P. 102982 - 102982
Published: Aug. 2, 2024
The integration of metaheuristics with machine learning methodologies presents significant advantages, particularly in optimization and computational intelligence. This amalgamation leverages the global search capabilities alongside pattern recognition predictive prowess learning, facilitating enhanced convergence rates solution quality complex problem spaces. Quantum Long Short-Term Memory (QLSTM) emerges as a highly efficient deep model tailored to tackle such intricate engineering problems. QLSTM's architecture, comprising data encoding, variational, quantum measurement layers, facilitates effective encoding processing civil data, leading heightened prediction accuracy. However, task determining optimal values for QLSTM parameters challenges due its NP-problem nature time-consuming characteristics. To address this, we propose an alternative technique optimize based on modified Electric Eel Foraging Optimization (MEEFO). MEEFO is version original EEFO that applies triangular mutation operators boost capability traditional EEFO. Thus, optimizes boosts performance. validate efficacy our proposed method, conduct comprehensive experiments utilizing five real-world datasets related construction structure engineering. evaluation outcomes unequivocally demonstrate MMEFO significantly enhances performance QLSTM.
Language: Английский
Citations
3Case Studies in Construction Materials, Journal Year: 2024, Volume and Issue: 21, P. e03820 - e03820
Published: Oct. 6, 2024
Language: Английский
Citations
3Case Studies in Construction Materials, Journal Year: 2025, Volume and Issue: unknown, P. e04357 - e04357
Published: Feb. 1, 2025
Language: Английский
Citations
0