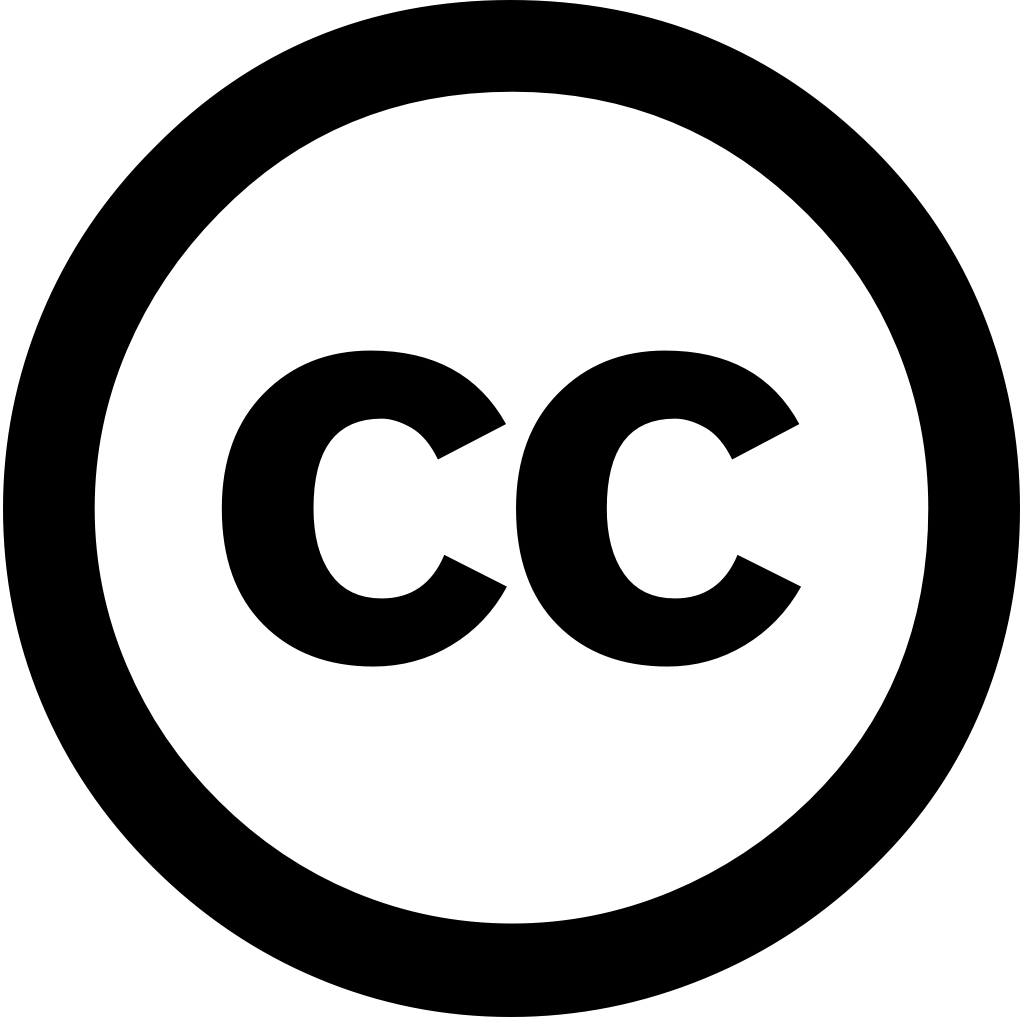
Smart Agricultural Technology, Journal Year: 2024, Volume and Issue: unknown, P. 100746 - 100746
Published: Dec. 1, 2024
Language: Английский
Smart Agricultural Technology, Journal Year: 2024, Volume and Issue: unknown, P. 100746 - 100746
Published: Dec. 1, 2024
Language: Английский
Humanities and Social Sciences Communications, Journal Year: 2025, Volume and Issue: 12(1)
Published: Jan. 3, 2025
Abstract Marine fisheries constitute a crucial component of global green development, where artificial intelligence (AI) plays an essential role in enhancing economic efficiency associated with marine fisheries. This study utilizes panel data from 11 coastal provinces and municipalities China 2009 to 2020, employing the entropy method super-efficiency EBM model calculate AI index Based on these calculations, we utilize fixed effects models, moderation effect threshold models examine impact The reveals that: (i) From has significantly improved overall, while shown fluctuating trend, substantial regional disparities. (ii) enhances (iii) Green finance, trade openness, R&D investment act as moderating variables, accelerating development further improving (iv) varies across different intervals investment. These findings are for understanding advancing informatization strategy hold significant implications sustainable
Language: Английский
Citations
15Ecological Informatics, Journal Year: 2024, Volume and Issue: 80, P. 102501 - 102501
Published: Jan. 29, 2024
Dissolved oxygen (DO) level is an important indicator aquaculture quality. This study proposes ensembled method, WTD-GWO-SVR, combining wavelet threshold denoising (WTD), grey wolf optimization (GWO), and support vector regression (SVR) for accurately predicting DO levels. Addressing challenges such as high noise, poor data quality, non-linearity non-stationary properties of time series data, our method integrates SVR regression-based estimation, WTD denoising, GWO optimizing the parameters Gaussian kernel's radial basis function. We collected a dataset using variety low-cost sensors in real setting. Our comprehensive evaluation on demonstrates that WTD-GWO-SVR achieved mean squared error, absolute R2 values 0.38%, 3.81%, 99.73%, respectively. It also consistently outperformed back-propagation neural network long short-term memory model. superior computational performance compared to these methods. The throughput accuracy make it potential choice prediction water quality monitoring systems.
Language: Английский
Citations
13Ecological Informatics, Journal Year: 2024, Volume and Issue: 80, P. 102482 - 102482
Published: Jan. 21, 2024
Caenorhabditis elegans is a representative organism whose DNA structure has been fully elucidated. It used as model for various analyses, including genetic functional analysis, individual behavioral and group analysis. Recently, it also studied an important bioindicator of water pollution. In previous studies, traditional machine learning methods, such the Hidden Markov Model (HMM), were to determine pollution identify pollutants based on differences in swimming behavior C. before after exposure chemicals. However, these models have low accuracy relatively high false-negative rate. This study proposes method detecting identifying types using Long Short-Term Memory (LSTM) model, deep suitable time-series data The activities each image frames are characterized by Branch Length Similarity (BLS) entropy profile. These BLS profiles converted into input vectors through additional preprocessing two clustering methods. We conduct experiments formaldehyde benzene at 0.1 mg/L each, with observation time intervals varying from 30 180 s. performance proposed compared that previously HMM approach variants LSTM models, Gated Recurrent Unit (GRU) Bidirectional (BiLSTM).
Language: Английский
Citations
7Ecological Informatics, Journal Year: 2023, Volume and Issue: 79, P. 102405 - 102405
Published: Dec. 12, 2023
Water contamination presents a significant challenge in aquaculture, impacting the sustainability of ecosystems and health aquatic organisms. Precisely assessing water levels is crucial for effective monitoring safeguarding life within aquaculture industry. Traditional methods evaluating are characterized by their costliness, time-consuming nature, susceptibility to errors. Integrating computer technologies such as Artificial Intelligence (AI), Internet Things (IoT), Data Analytics offers promising potential addressing this issue. Nevertheless, current deep learning solutions have limitations related data variability, interpretability, performance. To address these limitations, study proposes comprehensive framework that incorporates IoT-based collection segregation techniques enhance accuracy classification aquaculture. Real-time collected through IoT devices, encompassing parameters like temperature, pH levels, dissolved oxygen, nitrate concentration, other quality indicators, enables holistic evaluation quality. By considering predefined acceptable ranges life, calculates index, facilitating into categories contaminated non-contaminated. ensure robust classification, introduces an innovative attention-based model known Ordinary Differential Equation Gated Recurrent Unit (AODEGRU). This attention mechanism directs model's focus towards salient features associated with contamination, while AODEGRU architecture captures temporal patterns data. Experimental results underscore effectiveness proposed model. It demonstrates its superiority high performance, achieving rate approximately 98.69% on publicly available dataset impressive 99.89% real-time dataset, clearly outperforming existing methodologies.
Language: Английский
Citations
16Artificial Intelligence in Agriculture, Journal Year: 2025, Volume and Issue: unknown
Published: Jan. 1, 2025
Language: Английский
Citations
0Computers and Electronics in Agriculture, Journal Year: 2025, Volume and Issue: 232, P. 110127 - 110127
Published: Feb. 16, 2025
Language: Английский
Citations
0Expert Systems with Applications, Journal Year: 2025, Volume and Issue: unknown, P. 126820 - 126820
Published: Feb. 1, 2025
Citations
0Energy Sustainable Development/Energy for sustainable development, Journal Year: 2025, Volume and Issue: 85, P. 101683 - 101683
Published: Feb. 20, 2025
Language: Английский
Citations
0Journal of ISMAC, Journal Year: 2025, Volume and Issue: 7(1), P. 18 - 41
Published: Feb. 27, 2025
The increasing demand for sustainable aquaculture necessitates efficient water quality management to enhance fish health, reduce mortality rates, and improve overall productivity. However, conventional monitoring relies on manual testing, which is labour-intensive, time-consuming, ineffective in detecting rapid environmental fluctuations. To address these limitations, this study presents EcoGuard, an IoT-enabled smart system that integrates edge computing federated learning-based predictive analytics real-time assessment management. EcoGuard continuously monitors the important parameters, including pH, dissolved oxygen (DO), temperature, turbidity, ammonia levels, through a wireless sensor network. module, employing Random Forest Long Short-Term Memory (LSTM) models, forecasts trends, enabling early intervention risk mitigation. A key feature of its learning framework, facilitates collaborative model training across multiple farms while ensuring data privacy security. utilizes MQTT protocol low-latency transmission, integrated mobile application provides alerts decision support optimized resource Experimental validation demonstrates effectively reduces mortality, enhances operational efficiency, supports practices. By utilizing IoT, AI, learning, proposed offers scalable, cost-effective, intelligent solution modernizing aquaculture, contributing food security, conservation, resilient fisheries
Language: Английский
Citations
0Sensors, Journal Year: 2025, Volume and Issue: 25(6), P. 1846 - 1846
Published: March 16, 2025
In video-based fish surveys, species recognition plays a vital role in stock assessments, ecosystem analysis, production management, and protection of endangered species. However, implementing detection algorithms underwater environments presents significant challenges due to factors such as varying lighting conditions, water turbidity, the diverse appearances this work, transformer-enhanced YOLOv8 (YOLOv8-TF) is proposed for recognition. The YOLOv8-TF enhances performance by adjusting depth scales, incorporating transformer block into backbone neck, introducing class-aware loss function address class imbalance dataset. considers count instances within each assigns higher weight with fewer instances. This approach enables through object detection, encompassing classification localization estimate their position size an image. Experiments were conducted using 2021 Southeast Area Monitoring Assessment Program (SEAMAPD21) dataset, detailed extensive reef dataset from Gulf Mexico. experimental results on SEAMAPD21 demonstrate that model, mean Average Precision (mAP)0.5 87.9% mAP0.5–0.95 61.2%, achieves better compared state-of-the-art YOLO models. Additionally, publicly available datasets, Pascal VOC MS COCO datasets model outperforms existing approaches.
Language: Английский
Citations
0