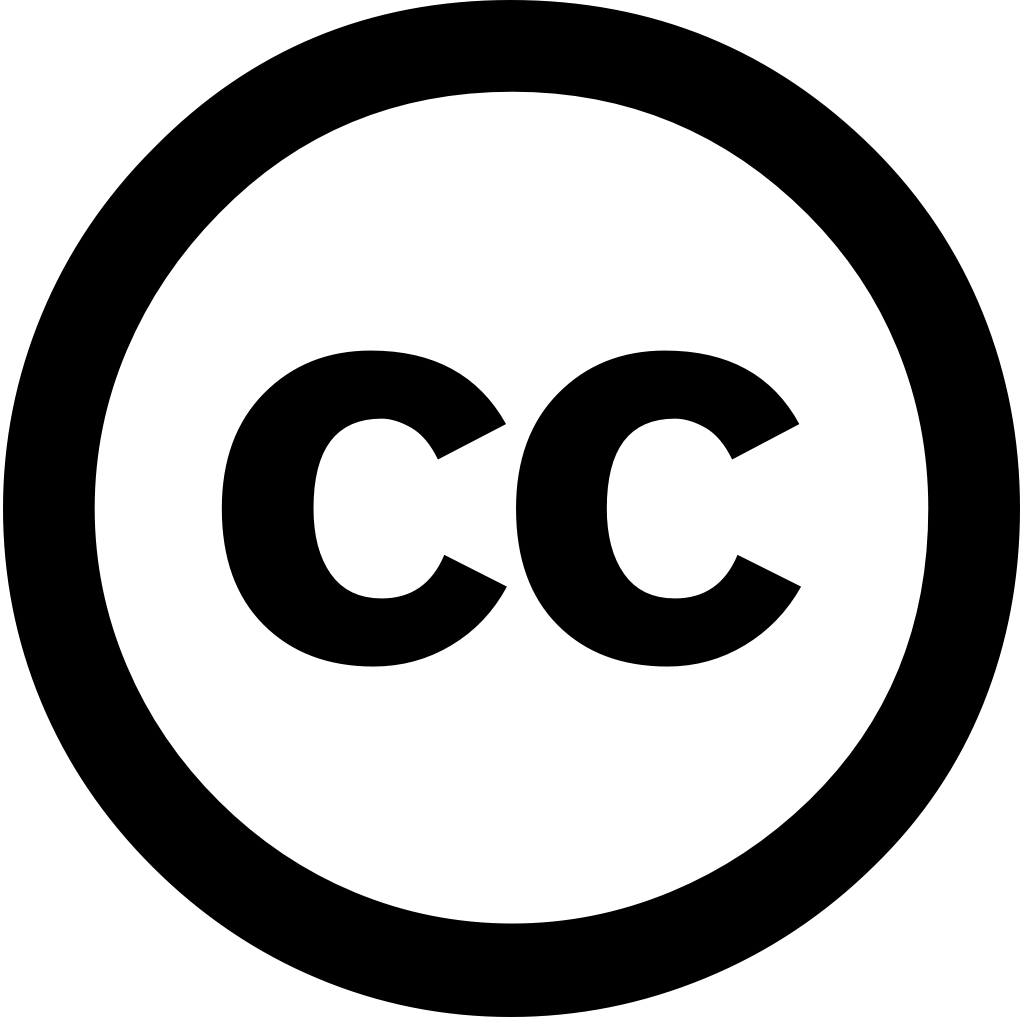
Ecological Informatics, Journal Year: 2024, Volume and Issue: 83, P. 102797 - 102797
Published: Aug. 26, 2024
Language: Английский
Ecological Informatics, Journal Year: 2024, Volume and Issue: 83, P. 102797 - 102797
Published: Aug. 26, 2024
Language: Английский
Ecological Informatics, Journal Year: 2024, Volume and Issue: 81, P. 102651 - 102651
Published: May 24, 2024
Wild Cervidae(deer and their relatives) play a crucial role in maintaining ecological balance are integral components of ecosystems. However, factors such as environmental changes poaching behaviors have resulted habitat degradation for Cervidae. The protection wild Cervidae has become urgent, monitoring is one the key means to ensure effectiveness protection. Object detection algorithms based on deep learning offer promising potential automatically detecting identifying animals. when those used inference unseen background environments, there will be significant decrease accuracy, especially situation that certain type images collected from single scene algorithm training. In this paper, two-stage localization classification pipeline proposed. effectively reduces interference enhances accuracy. first stage, YOLOv7 network designed locate UAV infrared images, while implementing improved bounding box regression through α-IoU loss function enables more accurately. Then, Cevdidae objects extracted eliminate information. second named CA-Hybrid, Convolutional Neural Networks(CNN) Vision Transformer(ViT), well Channel Attention Mechanism(CAM) expression features, constructed accurately identify categories. Experimental results indicate method achieves an Average Precision (AP) 95.9% location top-1 accuracy 77.73% identification. This research contributes comprehensive accurate Cervidae, provides valuable references subsequent UAV-based wildlife monitoring.
Language: Английский
Citations
5Computers and Electronics in Agriculture, Journal Year: 2024, Volume and Issue: 227, P. 109544 - 109544
Published: Oct. 20, 2024
Language: Английский
Citations
5Ecological Informatics, Journal Year: 2024, Volume and Issue: unknown, P. 102893 - 102893
Published: Nov. 1, 2024
Language: Английский
Citations
5Ecological Informatics, Journal Year: 2024, Volume and Issue: 82, P. 102705 - 102705
Published: June 29, 2024
Camera traps serve as a valuable tool for wildlife monitoring, generating vast collection of images ecologists to conduct ecological investigations, such species identification and population estimation. However, the sheer volume poses challenge, integration deep learning into automated investigation tasks remains complex, particularly when dealing with low-quality in long-term monitoring programs. Existing approaches often struggle strike balance between image enhancement tasks, thereby overlooking crucial information contained within images. This research introduces pioneering adaptive processing module (AIP) that seamlessly incorporates camera trap elevating performance activities. Specifically, differentiable (DIP) is presented enhance images, its parameters predicted by Non-local based parameter predictor (NLPP). Additionally, an end-to-end approach on hybrid data containing both original synthetic proposed, encompassing methods downstream traps, adaptable various scenarios. effectively reduces manual labor time required professional processing. When applied real-world datasets, our method achieves accuracy 92.26% 86.65% classifying wildlife, respectively, demonstrating robustness. By outperforming alternative under harsh conditions, application instills greater confidence applications complex environments.
Language: Английский
Citations
4Ecological Informatics, Journal Year: 2024, Volume and Issue: 80, P. 102527 - 102527
Published: Feb. 17, 2024
Transfer learning is extensively utilized for automatically recognizing and filtering out empty camera trap images that lack animal presence. Current research uses transfer identifying typically solely updates the fully connected layer of models, they usually select a pre-trained source model only based on its relevance to target task. However, do not consider optimization update selection, nor investigate effect sample size class number domain data set used construct performance model. Both these are issues worth exploring. We answered two using three different datasets ResNext-101 Our experimental results showed when 20,000 training samples from ImageNet dataset Snapshot Serengeti dataset, our proposed optimal layers improved accuracy 92.9% 95.5% (z = −7.087, p < 0.001, N 8118) compared existing method updating layer. A similar improvement was observed transferring Lasha Mountain dataset. Additionally, indicated increasing binary-class build 100,000 1 million, 90.4% 93.5% −3.869, 8948). Similar were obtained constructing ten classifications. Based results, we drew following conclusions: (1) instead commonly can significantly improve model's performance. (2) The varied transferred same (3) classes in did impact positively correlated with performance, there might be threshold effect.
Language: Английский
Citations
3Ecological Informatics, Journal Year: 2025, Volume and Issue: unknown, P. 103046 - 103046
Published: Jan. 1, 2025
Language: Английский
Citations
0Ecological Informatics, Journal Year: 2024, Volume and Issue: 83, P. 102805 - 102805
Published: Sept. 2, 2024
Camera trap imagery has become an invaluable asset in contemporary wildlife surveillance, enabling researchers to observe and investigate the behaviors of wild animals. While existing methods rely solely on image data for classification, this may not suffice cases suboptimal animal angles, lighting, or quality. This study introduces a novel approach that enhances classification by combining specific metadata (temperature, location, time, etc) with data. Using dataset focused Norwegian climate, our models show accuracy increase from 98.4% 98.9% compared methods. Notably, also achieves high metadata-only highlighting its potential reduce reliance work paves way integrated systems advance technology.
Language: Английский
Citations
1Lecture notes in computer science, Journal Year: 2024, Volume and Issue: unknown, P. 75 - 84
Published: Jan. 1, 2024
Language: Английский
Citations
0Animals, Journal Year: 2024, Volume and Issue: 14(23), P. 3353 - 3353
Published: Nov. 21, 2024
Poyang Lake is the largest freshwater lake in China and plays a significant ecological role. Deep-learning-based video surveillance can effectively monitor bird species on lake, contributing to local biodiversity preservation. To address challenges of multi-scale object detection against complex backgrounds, such as high density severe occlusion, we propose new model known YOLOv8-bird model. First, use Receptive-Field Attention convolution, which improves model's ability capture utilize image information. Second, redesign feature fusion network, termed DyASF-P2, enhances network's small features reduces target information loss. Third, lightweight head designed reduce size without sacrificing precision. Last, Inner-ShapeIoU loss function proposed localization challenge. Experimental results PYL-5-2023 dataset demonstrate that achieves precision, recall, [email protected], [email protected]:0.95 scores 94.6%, 89.4%, 94.8%, 70.4%, respectively. Additionally, outperforms other mainstream models terms accuracy. These indicate well-suited for counting tasks, enable it support monitoring environment Lake.
Language: Английский
Citations
0Insects, Journal Year: 2024, Volume and Issue: 15(12), P. 974 - 974
Published: Dec. 6, 2024
The honey bee (
Language: Английский
Citations
0