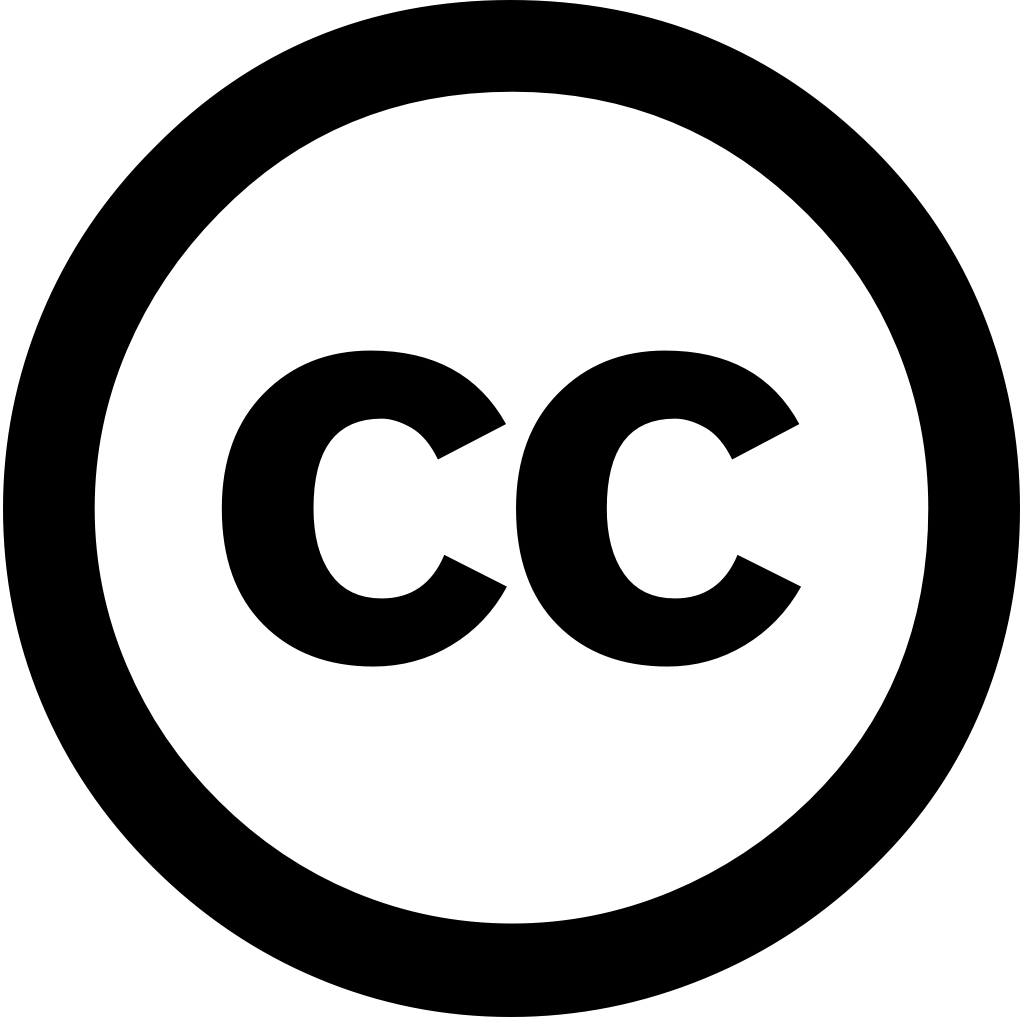
Ecological Informatics, Journal Year: 2024, Volume and Issue: unknown, P. 102939 - 102939
Published: Dec. 1, 2024
Language: Английский
Ecological Informatics, Journal Year: 2024, Volume and Issue: unknown, P. 102939 - 102939
Published: Dec. 1, 2024
Language: Английский
Journal of Animal Science and Technology, Journal Year: 2025, Volume and Issue: 67(1), P. 43 - 55
Published: Jan. 1, 2025
This review examines the application of video processing and convolutional neural network (CNN)-based deep learning for animal face recognition, identification, re-identification. These technologies are essential precision livestock farming, addressing challenges in production efficiency, welfare, environmental impact. With advancements computer technology, monitoring systems have evolved into sensor-based contact methods video-based non-contact methods. Recent developments enable continuous analysis accumulated data, automating conditions. By integrating with CNN-based learning, it is possible to estimate growth, identify individuals, monitor behavior more effectively. enhance management systems, leading improved outcomes, sustainability farming practices.
Language: Английский
Citations
1Ecological Informatics, Journal Year: 2024, Volume and Issue: 81, P. 102556 - 102556
Published: March 20, 2024
Procapra przewalskii, which inhabits plateau areas, faces the constant threat of poaching and unpredictable risks that impede its survival. The implementation a comprehensive, real-time monitoring tracking system for przewalskii using artificial intelligence unmanned aerial vehicle (UAV) technology is crucial to safeguard existence. Therefore, UAV multi-object-tracking (MOT) with global motion compensation (GMC) was proposed in this study. YOLOv7 Deep SORT were employed object detection tracking, respectively. Furthermore, Kalman filter (KF) optimized enhance accuracy object-tracking. Moreover, novel appearance feature-extraction network (FEN) introduced enable more effective multi-scale feature (MSF) extraction. In addition, GMC module align neighboring frames through matching. This facilitates correction position target subsequent frame, mitigating impact camera on tracking. results demonstrated remarkable system. Compared model, exhibited an increase 6.4% MOTA, 2.7% MOTP, 7.9% IDF1. Through comprehensive evaluation analysis real-world scenarios, study exhibits reliability complex scenes holds potential significantly protection from threats.
Language: Английский
Citations
7Ecological Informatics, Journal Year: 2024, Volume and Issue: 82, P. 102689 - 102689
Published: June 15, 2024
Currently, aquaculture methods tend to combine scale and intelligence, which saves manpower improves the survival rate of seafood at same time. High-precision high-efficiency fish individual recognition can provide key technical support for disease detection, feeding habits, body condition, etc. In realm intelligent aquaculture, it provides robust data precision farming. However, current research struggle maintain network model's focus on in real marine underwater complex environments (e.g., environmental background interference such as coral reefs, overlap between bodies, light noise, etc.), leading unsatisfactory results. To this end, paper proposes a method based video object segmentation, consists three parts, including segmentation detection module, an all-in-one visualization module. The work adopts combination deep learning algorithms solve problem low attention poor accuracy individuals environments, effectively efficiency recognition, analyzes discusses comparison effects using different weights. results simulation experiments show that metric Rank1 value achieves more than 96% public datasets DlouFish, WideFish, Fish-seg dataset produced paper, over state-of-the-art by 2.23%, 1.33%, 1.25%, respectively.
Language: Английский
Citations
6Ecological Informatics, Journal Year: 2025, Volume and Issue: unknown, P. 103009 - 103009
Published: Jan. 1, 2025
Language: Английский
Citations
0Ecological Informatics, Journal Year: 2025, Volume and Issue: 87, P. 103091 - 103091
Published: March 5, 2025
Language: Английский
Citations
0Ecological Informatics, Journal Year: 2024, Volume and Issue: 82, P. 102707 - 102707
Published: June 29, 2024
Using 51 orthomosaics of 11 breeding locations the Antarctic shag (Leucocarbo bransfieldensis), we propose a method for automating counting nests. This is achieved by training an object detection model based on "You Only Look Once" (YOLO) architecture and identifying nests sections orthomosaic, which are later combined with predictions entire orthomosaic. Our results show that current use Remotely Piloted Aircraft Systems (RPAS) to collect images areas colonies, machine learning algorithms, can provide reliable fast estimates nest counts (F1 score > 0.95). By using data from only two colonies training, models be obtained generalise well both spatially temporally distinct colonies. The proposed practical application opens possibility aerial imagery perform large-scale surveys islands in search undiscovered We discuss conditions optimal performance as its limitations. code, trained allowing full reproducibility available at https://github.com/Appsilon/Antarctic-nests.
Language: Английский
Citations
3Ecological Informatics, Journal Year: 2024, Volume and Issue: 83, P. 102802 - 102802
Published: Aug. 28, 2024
Alligator sinensis is an extremely rare species that possesses excellent camouflage, allowing it to fit perfectly into its natural environment. The use of camouflage makes detection difficult for both humans and automated systems, highlighting the importance modern technologies animal monitoring. To address this issue, we present YOLO v8-SIM, innovative technique specifically developed significantly enhance identification precision. v8-SIM utilizes a sophisticated dual-layer attention mechanism, optimized loss function called inner intersection-over-union (IoU), slim-neck cross-layer hopping. results our study demonstrate model achieves accuracy rate 91 %, recall 89.9 mean average precision (mAP) 92.3 % IoU threshold 0.5. In addition, operates at frame 72.21 frames per second (FPS) excels accurately recognizing objects are partially visible or smaller in size. further improve initiatives, suggest creating open-source collection data showcases A. native environment while using techniques. These developments collectively ability detect disguised animals, thereby promoting monitoring protection biodiversity, supporting ecosystem sustainability.
Language: Английский
Citations
3Expert Systems with Applications, Journal Year: 2025, Volume and Issue: unknown, P. 127525 - 127525
Published: April 1, 2025
Language: Английский
Citations
0Ecological Informatics, Journal Year: 2024, Volume and Issue: 83, P. 102794 - 102794
Published: Aug. 24, 2024
Language: Английский
Citations
1Sustainable Computing Informatics and Systems, Journal Year: 2024, Volume and Issue: unknown, P. 101070 - 101070
Published: Dec. 1, 2024
Language: Английский
Citations
1