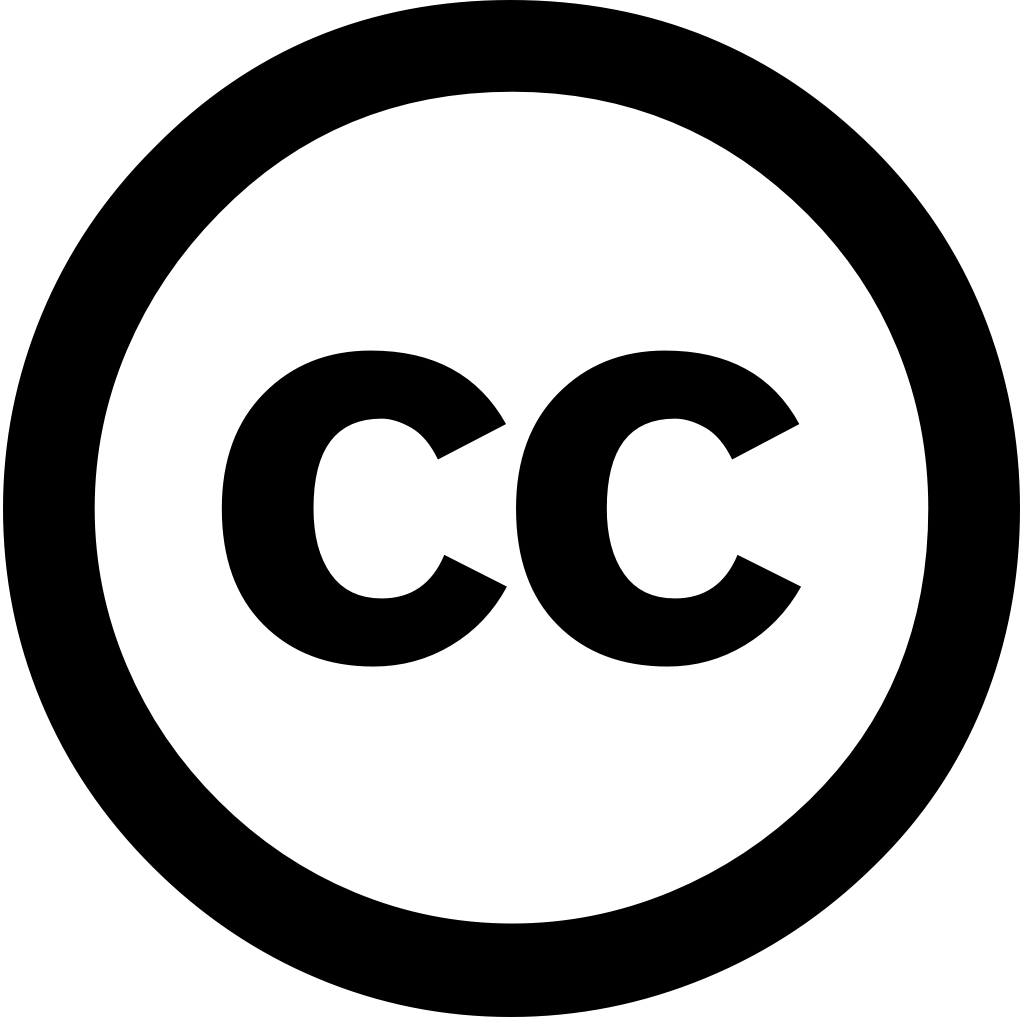
Ecological Informatics, Journal Year: 2025, Volume and Issue: unknown, P. 103135 - 103135
Published: April 1, 2025
Language: Английский
Ecological Informatics, Journal Year: 2025, Volume and Issue: unknown, P. 103135 - 103135
Published: April 1, 2025
Language: Английский
Drones, Journal Year: 2025, Volume and Issue: 9(3), P. 217 - 217
Published: March 18, 2025
Existing aerial forest fire monitoring data primarily consist of infrared or visible light images. However, there is a lack in-depth research on the ability models to perceive regions across different spectral To address this, we first constructed dataset and images captured in same scene, from perspective, at time, with pixel-level segmentation annotations flame In response issues poor performance current large number learnable parameters models, propose an improved model algorithm, SAMFA (Segmentation Anything Model, Fire, Adapter). Firstly, while freezing original model, only additionally incorporated Adapter module fine-tuned better adapt network specificities task. Secondly, enhance network’s perception edges, U-shaped mask decoder designed. Lastly, reduce training difficulty, progressive strategy combining self-supervised fully supervised learning employed optimize entire model. We compared five state-of-the-art image algorithms labeled public dataset, experimental results demonstrate that performs best. Compared SAM, improves IoU by 11.94% 6.45% images, respectively, reducing 11.58 M.
Language: Английский
Citations
0Remote Sensing in Earth Systems Sciences, Journal Year: 2025, Volume and Issue: unknown
Published: March 25, 2025
Language: Английский
Citations
0Ecological Informatics, Journal Year: 2025, Volume and Issue: unknown, P. 103135 - 103135
Published: April 1, 2025
Language: Английский
Citations
0