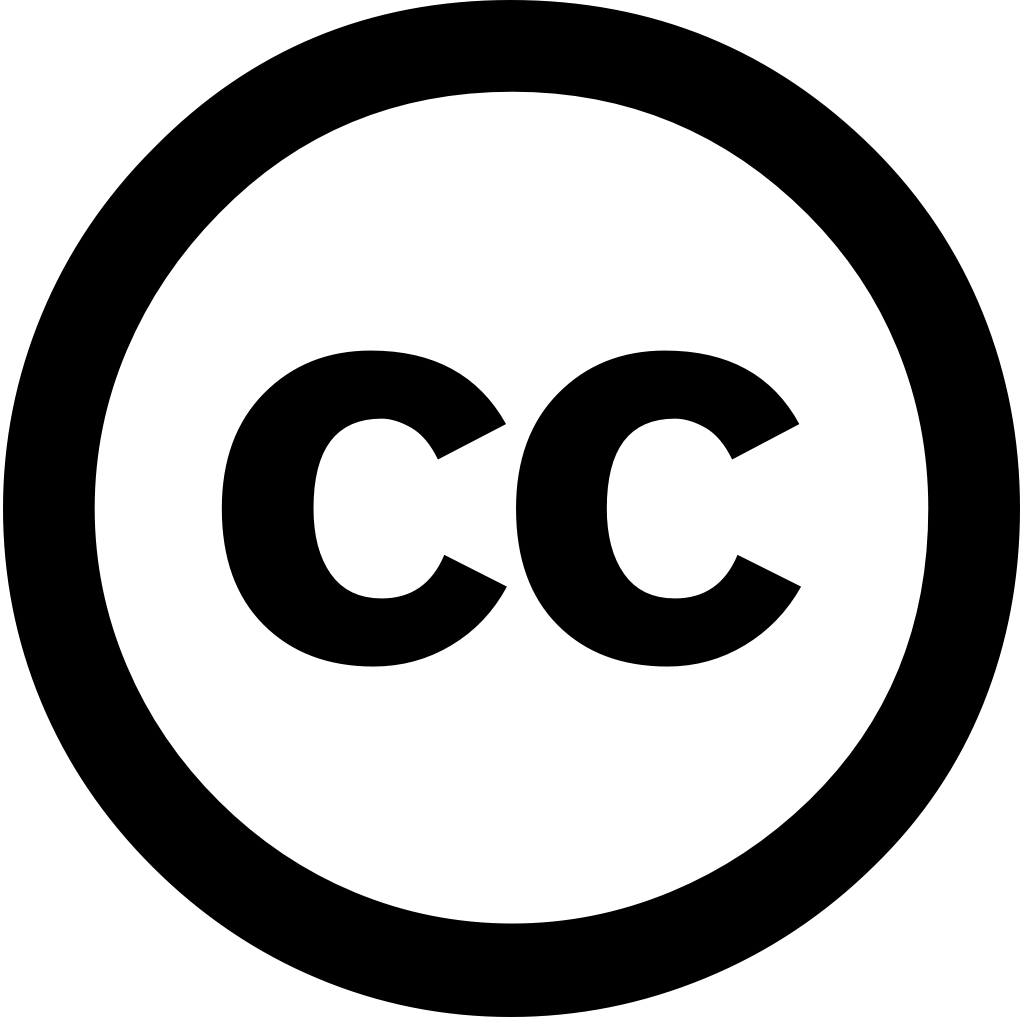
Ecological Informatics, Journal Year: 2024, Volume and Issue: unknown, P. 102964 - 102964
Published: Dec. 1, 2024
Language: Английский
Ecological Informatics, Journal Year: 2024, Volume and Issue: unknown, P. 102964 - 102964
Published: Dec. 1, 2024
Language: Английский
Scientific Reports, Journal Year: 2025, Volume and Issue: 15(1)
Published: Jan. 28, 2025
In agriculture, promptly and accurately identifying leaf diseases is crucial for sustainable crop production. To address this requirement, research introduces a hybrid deep learning model that combines the visual geometric group version 19 (VGG19) architecture features with transformer encoder blocks. This fusion enables accurate précised real-time classification of affecting grape, bell pepper, tomato plants. Incorporating blocks offers enhanced capability in capturing intricate spatial dependencies within images, promising agricultural sustainability food security. By providing farmers farming stakeholders reliable tool rapid disease detection, our facilitates timely intervention management practices, ultimately leading to improved yields mitigated economic losses. Through extensive comparative analyses on various datasets filed tests, proposed depth wise separable convolutional-TransNet (DSC-TransNet) has demonstrated higher performance terms accuracy (99.97%), precision (99.94%), recall (99.94), sensitivity F1-score AUC (0.98) Grpae leaves across different including pepper tomato. Furthermore, DSC layers enhances computational efficiency while maintaining expressive power, making it well-suited applications. The developed DSC-TransNet deployed NVIDIA Jetson Nano single board computer. contributes advancing field automated plant classification, addressing critical challenges modern agriculture promoting more efficient practices.
Language: Английский
Citations
1Ecological Informatics, Journal Year: 2024, Volume and Issue: 82, P. 102683 - 102683
Published: June 12, 2024
Poisonous plants are the third largest category of poisons known globally, which pose a risk poisoning and death to humans. Currently, identification medicinal poisonous is done by humans using experimental methods, not accurate associated with many errors, also use laboratory methods requires experts this method very costly time-consuming. Therefore, distinguishing between important emerging, non-destructive, fast such as computer vision artificial intelligence. In study, we propose robust generalized model spatial attention (SA) channel (CA) modules for classification different plants. A dataset containing 900 confirmed images three plant classes (oregano, weed) was used. The mechanisms enhance efficiency deep learning (DL) networks allowing them precisely focus on all relevant input elements. order performance proposed model, CA implemented based four pooling operations including global average pooling-based (GAP-CA), mixed (Mixed-CA), gated (Gated-CA), tree (Tree-CA) operations. results showed that DL Tree-CA had promising outperformed other state-of-the-art models, achieving values 99.63%, 99.38%, 99.52%, 99.74%, 99.42%, accuracy, precision, recall, specificity, F1-score, respectively. findings support our model's success in identifying from similar Recent advancements computer-based technologies intelligence enable automatic detection plants, revolutionizing traditional methods.
Language: Английский
Citations
8Engineering Science and Technology an International Journal, Journal Year: 2025, Volume and Issue: 61, P. 101940 - 101940
Published: Jan. 1, 2025
Language: Английский
Citations
0Ecological Informatics, Journal Year: 2025, Volume and Issue: unknown, P. 103063 - 103063
Published: Feb. 1, 2025
Language: Английский
Citations
0Ecological Informatics, Journal Year: 2025, Volume and Issue: unknown, P. 103088 - 103088
Published: March 1, 2025
Language: Английский
Citations
0Plant Methods, Journal Year: 2025, Volume and Issue: 21(1)
Published: March 18, 2025
Rapid and accurate identification of soybean leaf diseases is crucial for optimizing crop health yield. We propose a cell P system with membrane division dissolution rules (DDC-P system) disease identification. Among them, the designed Efficient feature attention (EFA) lightweight sandglass structure efficient (SGEFA) can focus on disease-specific information while reducing environmental interference. A fuzzy controller was developed to manage SGEFA membranes, allowing adaptive adjustments model avoiding redundancy. Experimental results homemade dataset show that DDC-P achieves recognition rate 98.43% an F1 score 0.9874, size only 1.41 MB. On public dataset, accuracy 94.40% 0.9425. The average time edge device 0.042857 s, FPS 23.3. These outstanding demonstrate not excels in generalization but also ideally suited deployment devices, revolutionizing approach management.
Language: Английский
Citations
0Applied Intelligence, Journal Year: 2025, Volume and Issue: 55(7)
Published: March 25, 2025
Language: Английский
Citations
0Scientific Reports, Journal Year: 2025, Volume and Issue: 15(1)
Published: April 9, 2025
Language: Английский
Citations
0Horticulturae, Journal Year: 2025, Volume and Issue: 11(4), P. 437 - 437
Published: April 19, 2025
Tea leaf diseases are among the most critical factors affecting yield and quality of tea harvests. Due to climate change widespread pesticide use in cultivation, these have become more prevalent. As demand for high-quality continues rise, has assumed an increasingly prominent role global economy, thereby rendering continuous monitoring essential maintaining crop ensuring sustainable production. In this context, developing innovative agricultural policies is vital. Integrating artificial intelligence (AI)-based techniques with practices presents promising solutions. Ensuring that outputs interpretable would also provide significant value decision-makers, enhancing their applicability practices. study, advanced deep learning architectures such as ResNet50, MobileNet, EfficientNetB0, DenseNet121 were utilized classify diseases. Since low-resolution images complex backgrounds caused challenges, ensemble approach was proposed combine strengths models. The generalization performance model comprehensively evaluated through statistical cross-validation. Additionally, Grad-CAM visualizations demonstrated a clear correspondence between diseased regions disease types on leaves. Thus, models could detect under varying conditions, highlighting robustness. achieved high predictive performance, precision, recall, F1-score values 95%, 94%, 94% across folds. overall classification accuracy reached 96%, maximum standard deviation 2% all dataset specific leaves, confirming ability conditions accurately
Language: Английский
Citations
0Expert Systems, Journal Year: 2025, Volume and Issue: 42(6)
Published: April 27, 2025
ABSTRACT Plant disease detection is able to control spread and help prevent significant food production losses. However, existing methods are still limited different target scales high model parameters. To this end, we develop a novel framework, that is, FPDD‐Net, for lightweight plant detection. It based on YOLOv8 with an adaptive multi‐scale (AMSM) relationship‐based knowledge distillation (RKD). More specifically, the original cross stage partial (CSP) bottleneck replaced by AMSM effectively fuse features. Next, Alpha‐IoU loss optimization adopted aligning predicted boxes more precisely ground truth, leading fewer localization errors. Finally, RKD introduced assist training further improve performance of evaluate our network, FPDD‐Net trained tested two typical datasets, village dataset plant‐doc dataset. Experimental results indicated has advantages over peer methods.
Language: Английский
Citations
0