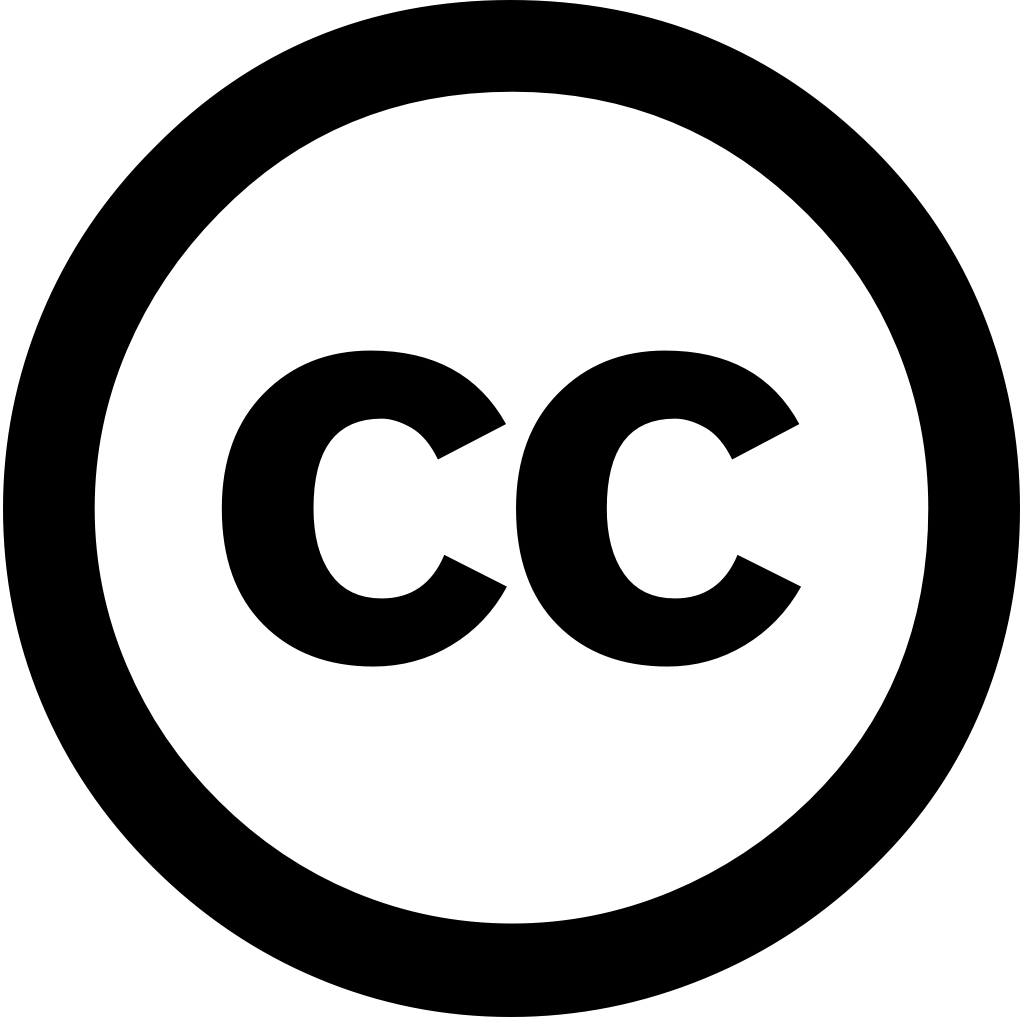
Ecological Informatics, Journal Year: 2024, Volume and Issue: unknown, P. 102964 - 102964
Published: Dec. 1, 2024
Language: Английский
Ecological Informatics, Journal Year: 2024, Volume and Issue: unknown, P. 102964 - 102964
Published: Dec. 1, 2024
Language: Английский
Ecological Informatics, Journal Year: 2024, Volume and Issue: 82, P. 102693 - 102693
Published: June 21, 2024
Crop pests cause significant losses to agricultural production. Pests can be detected and controlled over time using accurate effective methods, thereby reducing potential losses. However, there are challenges in realistic scenarios, such as diverse pest species complicated environments, which render manual recognition conventional machine learning methods insufficient. To address this issue, deep that automatically extract features have recently been widely used for identification. accurately recognizing images resemble complex real-world scenarios remains a challenging task single model. The ensemble method, combines multiple basic models, provides solution improving performance. In study, we proposed two weight-based VecEnsemble MatEnsemble, constructed from vector- matrix-based weights, respectively. weights combine models significantly influence the performance of methods. Therefore, effectively formulated weight design problem quadratic convex optimization whose has closed-form expression computed efficiently. Our method achieved highest accuracy 77.39% on large-scale complex-scene IP102 dataset, was competitive with those other state-of-the-art Furthermore, conducted comprehensive ablation experiments compare our voting-based approaches illustrate they applicable. These results highlight practical significance production provide foundation further research crop source code is available at https://github.com/shiguangqianmo/WBEnsemble.
Language: Английский
Citations
3Computers and Electronics in Agriculture, Journal Year: 2025, Volume and Issue: 232, P. 110066 - 110066
Published: Feb. 11, 2025
Language: Английский
Citations
0Ecological Informatics, Journal Year: 2024, Volume and Issue: unknown, P. 102925 - 102925
Published: Nov. 1, 2024
Language: Английский
Citations
2Ecological Informatics, Journal Year: 2024, Volume and Issue: unknown, P. 102905 - 102905
Published: Nov. 1, 2024
Language: Английский
Citations
1Agronomy, Journal Year: 2024, Volume and Issue: 14(11), P. 2605 - 2605
Published: Nov. 4, 2024
Accurate diagnosis of plant diseases is crucial for crop health. This study introduces the EDA–ViT model, a Vision Transformer (ViT)-based approach that integrates adaptive entropy-based data augmentation diagnosing custard apple (Annona squamosa) diseases. Traditional models like convolutional neural network and ViT face challenges with local feature extraction large dataset requirements. overcomes these by using multi-scale weighted aggregation interaction module, enhancing both global extraction. The method refines training process, boosting accuracy robustness. With 8226 images, achieved classification 96.58%, an F1 score 96.10%, Matthews Correlation Coefficient (MCC) 92.24%, outperforming other models. inclusion Deformable Multi-head Self-Attention (DMSA) mechanism further enhanced capture. Ablation studies revealed contributed to 0.56% improvement 0.34% increase in MCC. In summary, presents innovative solution disease diagnosis, potential applications broader agricultural detection, ultimately aiding precision agriculture health management.
Language: Английский
Citations
0Evolutionary Intelligence, Journal Year: 2024, Volume and Issue: 18(1)
Published: Nov. 26, 2024
Language: Английский
Citations
0Ecological Informatics, Journal Year: 2024, Volume and Issue: unknown, P. 102964 - 102964
Published: Dec. 1, 2024
Language: Английский
Citations
0