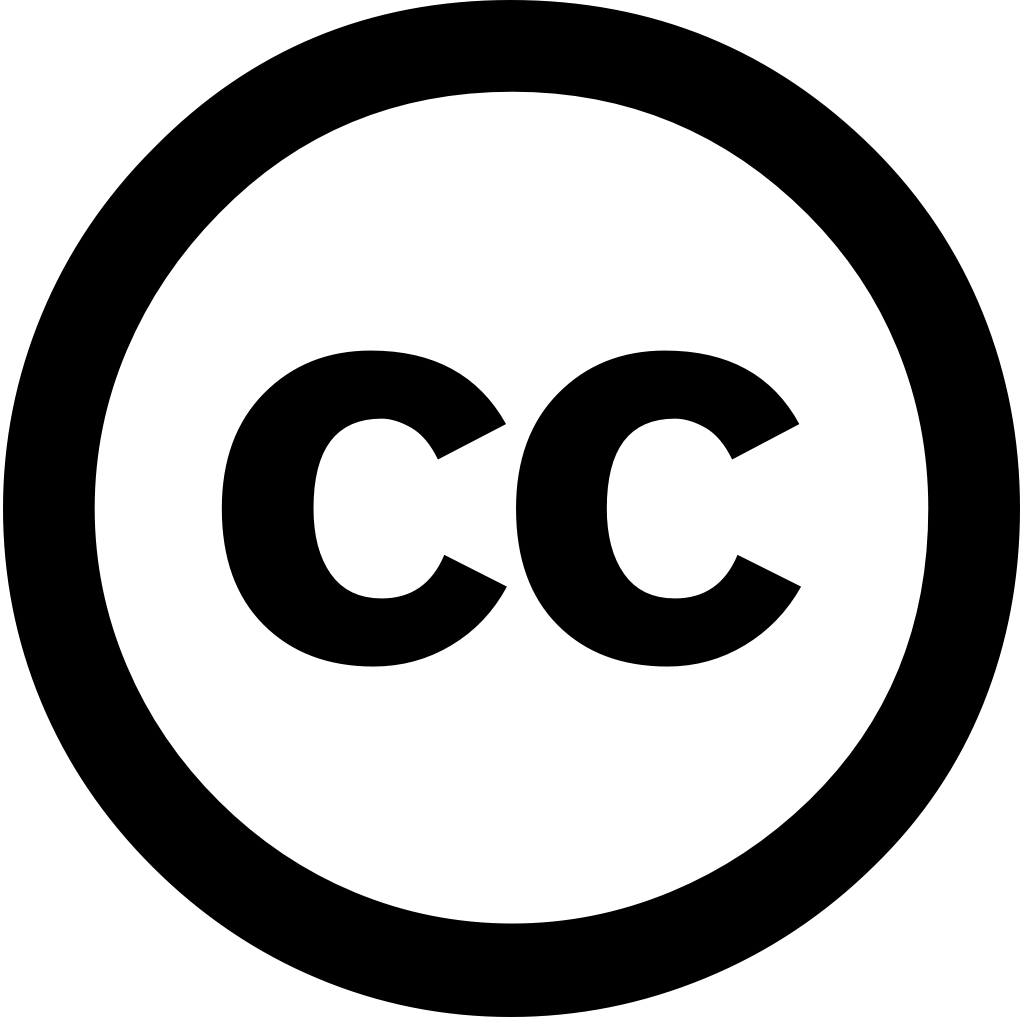
Artificial Intelligence Review, Journal Year: 2024, Volume and Issue: 57(12)
Published: Oct. 17, 2024
Language: Английский
Artificial Intelligence Review, Journal Year: 2024, Volume and Issue: 57(12)
Published: Oct. 17, 2024
Language: Английский
Ecological Informatics, Journal Year: 2024, Volume and Issue: 82, P. 102730 - 102730
Published: July 20, 2024
Language: Английский
Citations
6Nature Communications, Journal Year: 2024, Volume and Issue: 15(1)
Published: Dec. 19, 2024
Machine learning-based geospatial applications offer unique opportunities for environmental monitoring due to domains and scales adaptability computational efficiency. However, the specificity of data introduces biases in straightforward implementations. We identify a streamlined pipeline enhance model accuracy, addressing issues like imbalanced data, spatial autocorrelation, prediction errors, nuances generalization uncertainty estimation. examine tools techniques overcoming these obstacles provide insights into future AI developments. A big picture field is completed from advances processing general, including demands industry-related solutions relevant outcomes applied sciences. In this scoping review, authors explore challenges implementing data-driven models—namely machine learning deep algorithms—in research.
Language: Английский
Citations
5Ecological Informatics, Journal Year: 2024, Volume and Issue: 82, P. 102777 - 102777
Published: Aug. 23, 2024
Soil respiration (Rs), the second-largest flux in global carbon cycle, is a crucial but uncertain component. To improve understanding of Rs, we constructed single models, and specific models classified by climate type, land cover year data record, elevation range using random forest algorithm to predict Rs values explore associated uncertainty models. The results showed similar overall predictive performance for with an R-squared value greater than 0.63; however, significant differences were observed compared estimate (23 Pg C). All estimated larger model, mainly owing imbalances sample on which prediction based. One exception this result estimates smaller 2020 (95.1 Overall, model closer those obtained temperate zones training distribution, resulted other classification-specific Prediction observations before 2000 tend underestimate Rs. However, use proved helpful addressing persistent temporal spatial sampling. Expanding coverage records both temporally spatially updating database promptly would estimation accuracy while enhancing budget feedback soil regard warming.
Language: Английский
Citations
4Ecological Informatics, Journal Year: 2025, Volume and Issue: unknown, P. 103014 - 103014
Published: Jan. 1, 2025
Language: Английский
Citations
0Scientific Reports, Journal Year: 2025, Volume and Issue: 15(1)
Published: Feb. 25, 2025
Water quality indicators (WQI) reflect both the current state and changing trends of water quality. Extracting these from remote sensing data enables rapid efficient inversion conditions, providing a key step in predicting pollution. The low-precision results WQI limit understanding ecological safety resources. In this paper, Advanced Hyperspectral Imager (AHSI) GF-5 satellite was used to analyze Dianchi Lake. A spectrum processing method based on Savitzky-Golay Standard Normal Variate transformation (SG-SNV) developed, alongside optimal techniques, enhance accuracy predictions for 6 Dianchi, including CODcr, NH3-N, TP, TN, pH, Chl. indicated that (1) For all models, determination coefficients (R2) exceeded 0.85. Back Propagation Nondominated Sorting Genetic Algorithm-II (BP-NGA) model consistently yielded positive most WQI. (2) varied significantly by region season. (3) It recommended build wetlands parks southwest side improve sewage interception pipelines northeast lessen risk eutrophication reducing inflow nitrogen phosphorus.
Language: Английский
Citations
0Remote Sensing, Journal Year: 2025, Volume and Issue: 17(6), P. 1086 - 1086
Published: March 20, 2025
Accurate soil pH prediction is critical for management and ecological environmental protection. Machine learning (ML) models have been widely applied in the field of prediction. However, when using these models, spatial heterogeneity relationship between variables often not fully considered, which limits predictive capability especially large-scale regions with complex landscapes. To address challenges, this study collected data from 4335 surface points (0–20 cm) obtained China Soil System Survey, combined a multi-source covariate. This integrates Geographic Weighted Regression (GWR) three ML (Random Forest, Cubist, XGBoost) designs develops geographically weighted machine optimized by Genetic Algorithms to improve values. Compared GWR traditional R2 geographic random forest (GWRF), Cubist (GWCubist), extreme gradient boosting (GWXGBoost) increased 1.98% 14.29%, while RMSE decreased 1.81% 11.98%. Among GWRF model performed best effectively reduced uncertainty mapping. Mean Annual Precipitation Normalized Difference Vegetation Index are two key influencing pH, they significant negative impact on distribution pH. These findings provide scientific basis effective health implementation modeling programs.
Language: Английский
Citations
0Journal of Cleaner Production, Journal Year: 2025, Volume and Issue: unknown, P. 145616 - 145616
Published: April 1, 2025
Language: Английский
Citations
0Ecological Informatics, Journal Year: 2024, Volume and Issue: 85, P. 102944 - 102944
Published: Dec. 9, 2024
Language: Английский
Citations
3Ecological Informatics, Journal Year: 2025, Volume and Issue: unknown, P. 103057 - 103057
Published: Feb. 1, 2025
Language: Английский
Citations
0Spatial Statistics, Journal Year: 2025, Volume and Issue: 67, P. 100900 - 100900
Published: April 25, 2025
Language: Английский
Citations
0