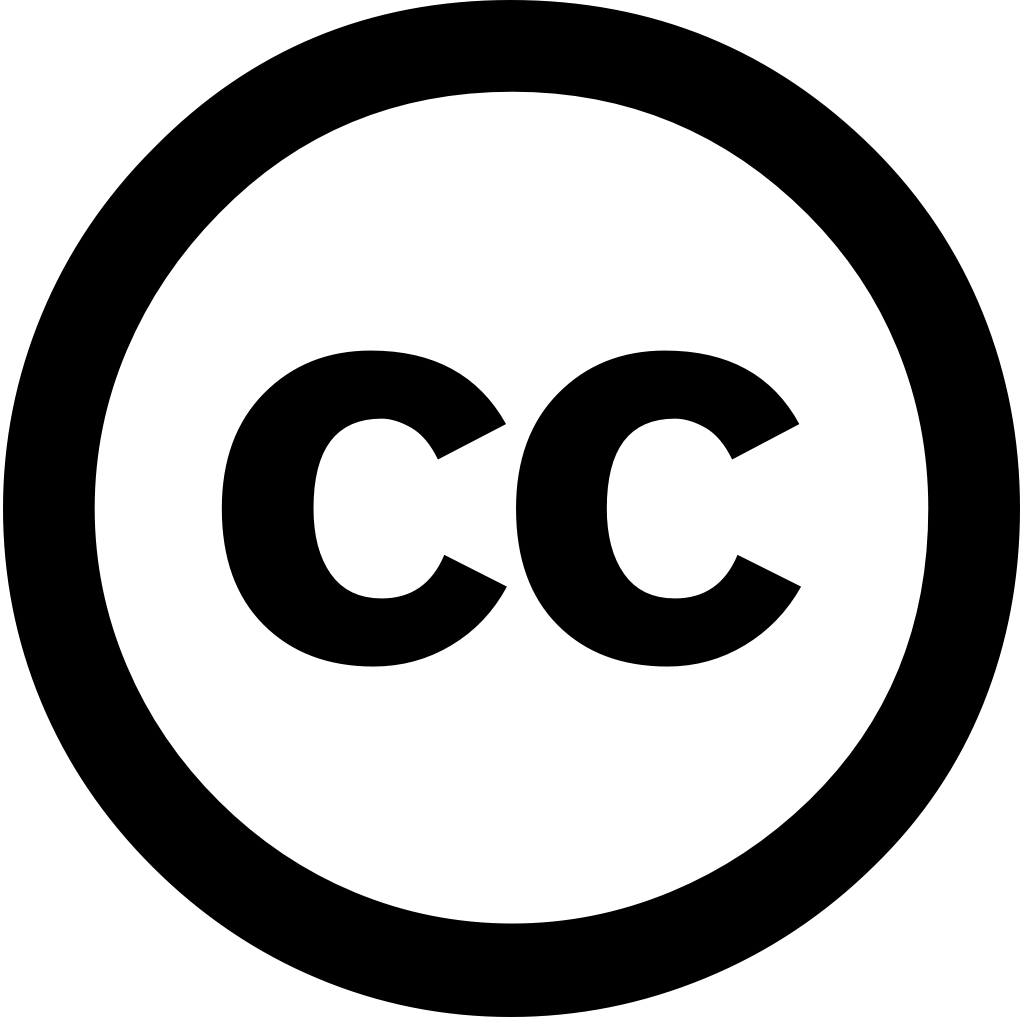
Ecological Informatics, Journal Year: 2024, Volume and Issue: unknown, P. 102966 - 102966
Published: Dec. 1, 2024
Language: Английский
Ecological Informatics, Journal Year: 2024, Volume and Issue: unknown, P. 102966 - 102966
Published: Dec. 1, 2024
Language: Английский
Ecological Informatics, Journal Year: 2025, Volume and Issue: unknown, P. 103067 - 103067
Published: Feb. 1, 2025
Language: Английский
Citations
1Applied Sciences, Journal Year: 2025, Volume and Issue: 15(7), P. 3778 - 3778
Published: March 30, 2025
To address the challenge posed by abundance of small objects with weak object features and little information in images underwater biomonitoring scenarios, added difficulty recognizing these due to light absorption scattering environment, this study proposes an improved RHS-YOLOv8 (Ref-Dilated-HBFPN-SOB-YOLOv8). Firstly, a combination hybrid inflated convolution RefConv is used redesign lightweight Ref-Dilated block, which reduces model computation. Second, new feature pyramid network fusion module, Hybrid Bridge Feature Pyramid Network (HBFPN), designed fuse deep high-level features, as well current layer, improve extraction capability for fuzzy objects. Third, Efficient Localization Attention (ELA) reduce interference irrelevant factors on prediction. Fourth, Involution module introduced effectively capture spatial long-range relationships recognition accuracy. Finally, detection branch incorporated into original architecture enhance model’s performance detecting Experiments based DUO dataset show that 9.95% computing power, while [email protected] [email protected]:0.95 are 2.54% 4.31%, respectively. Compared other cutting-edge algorithms, present algorithm improves accuracy lightweighting improvement, enhances detect
Language: Английский
Citations
1Ecological Informatics, Journal Year: 2024, Volume and Issue: 83, P. 102802 - 102802
Published: Aug. 28, 2024
Alligator sinensis is an extremely rare species that possesses excellent camouflage, allowing it to fit perfectly into its natural environment. The use of camouflage makes detection difficult for both humans and automated systems, highlighting the importance modern technologies animal monitoring. To address this issue, we present YOLO v8-SIM, innovative technique specifically developed significantly enhance identification precision. v8-SIM utilizes a sophisticated dual-layer attention mechanism, optimized loss function called inner intersection-over-union (IoU), slim-neck cross-layer hopping. results our study demonstrate model achieves accuracy rate 91 %, recall 89.9 mean average precision (mAP) 92.3 % IoU threshold 0.5. In addition, operates at frame 72.21 frames per second (FPS) excels accurately recognizing objects are partially visible or smaller in size. further improve initiatives, suggest creating open-source collection data showcases A. native environment while using techniques. These developments collectively ability detect disguised animals, thereby promoting monitoring protection biodiversity, supporting ecosystem sustainability.
Language: Английский
Citations
4Ecological Informatics, Journal Year: 2025, Volume and Issue: unknown, P. 103009 - 103009
Published: Jan. 1, 2025
Language: Английский
Citations
0Frontiers in Marine Science, Journal Year: 2025, Volume and Issue: 11
Published: Jan. 23, 2025
Underwater object detection plays a significant role in fisheries resource assessment and ecological environment protection. However, traditional underwater methods struggle to achieve accurate complex environments with limited computational resources. This paper proposes lightweight network called LightFusionNet-YOLO (LFN-YOLO). First, we introduce the reparameterization technique RepGhost reduce number of parameters while enhancing training inference efficiency. approach effectively minimizes precision loss even backbone network. Then, replaced standard depthwise convolution feature extraction SPD-Conv, which includes an additional pooling layer mitigate detail loss. modification enhances performance for small objects. Furthermore, We employed Generalized Feature Pyramid Network (GFPN) fusion network's neck, adaptability features varying scales. Finally, design new head, CLLAHead, reduces costs strengthens robustness model through cross-layer local attention. At same time, DFL function is introduced regression classification errors. Experiments conducted on public datasets, including URPC, Brackish, TrashCan, showed that [email protected] reached 74.1%, 97.5%, 66.2%, respectively, parameter sizes complexities 2.7M 7.2 GFLOPs, size only 5.9 Mb. Compared mainstream vision models, our demonstrates superior performance. Additionally, deployment NVIDIA Jetson AGX Orin edge computing device confirms its high real-time suitability applications, further showcasing exceptional capabilities LFN-YOLO.
Language: Английский
Citations
0Sensors, Journal Year: 2025, Volume and Issue: 25(6), P. 1846 - 1846
Published: March 16, 2025
In video-based fish surveys, species recognition plays a vital role in stock assessments, ecosystem analysis, production management, and protection of endangered species. However, implementing detection algorithms underwater environments presents significant challenges due to factors such as varying lighting conditions, water turbidity, the diverse appearances this work, transformer-enhanced YOLOv8 (YOLOv8-TF) is proposed for recognition. The YOLOv8-TF enhances performance by adjusting depth scales, incorporating transformer block into backbone neck, introducing class-aware loss function address class imbalance dataset. considers count instances within each assigns higher weight with fewer instances. This approach enables through object detection, encompassing classification localization estimate their position size an image. Experiments were conducted using 2021 Southeast Area Monitoring Assessment Program (SEAMAPD21) dataset, detailed extensive reef dataset from Gulf Mexico. experimental results on SEAMAPD21 demonstrate that model, mean Average Precision (mAP)0.5 87.9% mAP0.5–0.95 61.2%, achieves better compared state-of-the-art YOLO models. Additionally, publicly available datasets, Pascal VOC MS COCO datasets model outperforms existing approaches.
Language: Английский
Citations
0Journal of Real-Time Image Processing, Journal Year: 2025, Volume and Issue: 22(2)
Published: April 1, 2025
Language: Английский
Citations
0Journal of Real-Time Image Processing, Journal Year: 2025, Volume and Issue: 22(2)
Published: April 1, 2025
Language: Английский
Citations
0Ecological Informatics, Journal Year: 2025, Volume and Issue: unknown, P. 103185 - 103185
Published: May 1, 2025
Language: Английский
Citations
0Aquaculture International, Journal Year: 2025, Volume and Issue: 33(5)
Published: May 14, 2025
Language: Английский
Citations
0