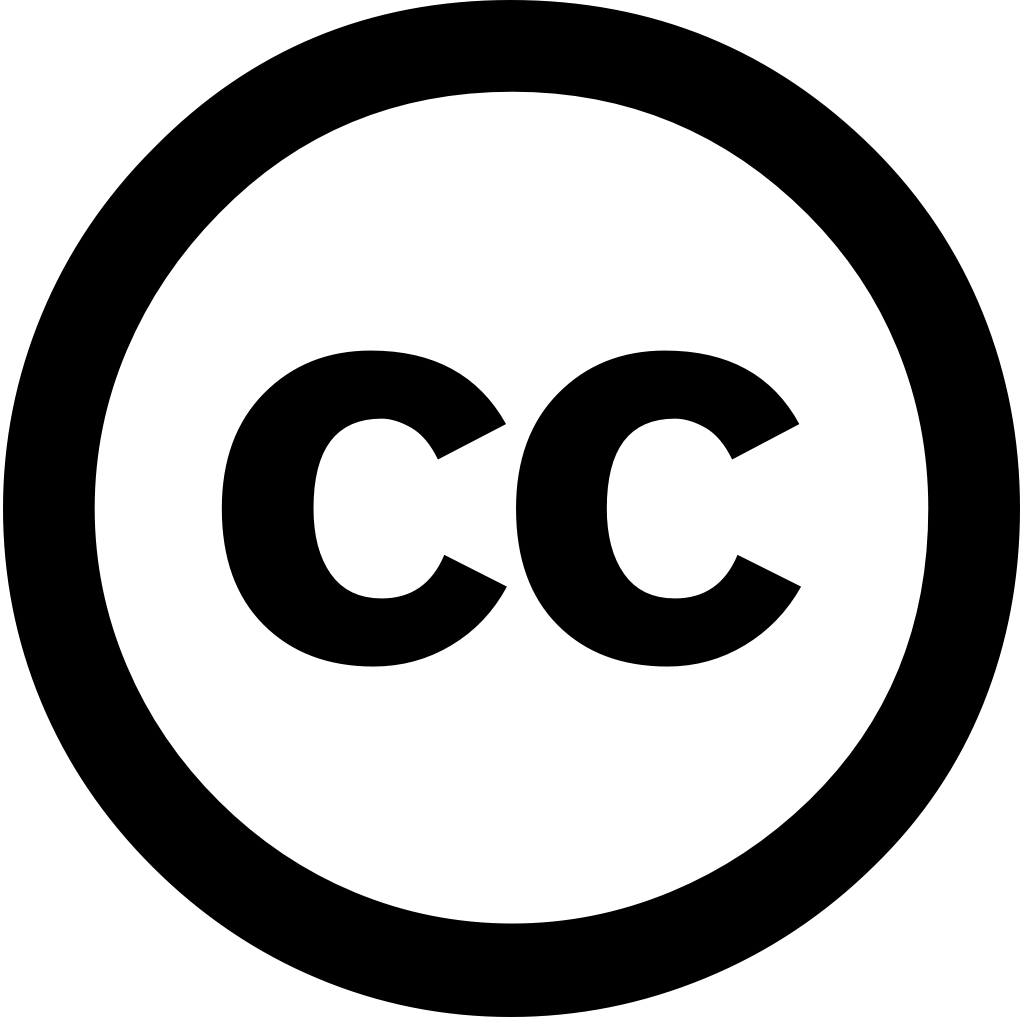
Smart Agricultural Technology, Journal Year: 2024, Volume and Issue: unknown, P. 100571 - 100571
Published: Sept. 1, 2024
Language: Английский
Smart Agricultural Technology, Journal Year: 2024, Volume and Issue: unknown, P. 100571 - 100571
Published: Sept. 1, 2024
Language: Английский
Atmosphere, Journal Year: 2023, Volume and Issue: 14(1), P. 172 - 172
Published: Jan. 13, 2023
Remote sensing is the technique of acquiring data from earth’s surface sensors installed on satellites or manned unmanned aircrafts. Its use common in dozens sectors science and technology, agriculture, atmosphere, soil, water, land surface, oceans coasts, snow ice, natural disasters, among others. This article focuses an in-depth literature review some most promising disciplines, which are asbestos–cement roof identification, vegetation oil gas industry, geology, with aim having clarity trends research these issues at international level. The relevant problems each sector have been highlighted, evidencing need for future area light technological advances multi- hyperspectral availability satellite images more precise spatial resolution. A bibliometric analysis proposed discipline network related keywords discussed. Finally, results suggest that policymakers, urban planners, mine, companies should consider remote as primary tool when planning comprehensive development strategies field parameter multitemporal analysis.
Language: Английский
Citations
23Remote Sensing, Journal Year: 2025, Volume and Issue: 17(3), P. 400 - 400
Published: Jan. 24, 2025
Grassland ecosystems provide a range of services in semi-arid and arid regions. However, they have significantly declined due to overgrazing desertification. In the current study, we employed Landsat time series data (TM, OLI, OLI-2) spanning from 1990 2024, combined with vegetation indices such as NDVI SAVI, along NDWI digital elevation models (DEMs), analyze land cover dynamics Ugii Lake watershed area, Mongolia. By integrating multisource remote sensing into advanced XGBoost (extreme gradient boosting) machine learning algorithm, achieved high classification accuracy, overall accuracies exceeding 94% Kappa coefficients greater than 0.92. The results revealed decline montane grasslands (−6.2%) an increase other grassland types, suggesting ecosystem redistribution influenced by climatic anthropogenic factors. Cropland exhibited resilience, recovering significant 1990s moderate growth 2024. Our findings highlight stability barren underscore pressures ecological degradation human activities. This study provides up-to-date statistical support decision-making conservation sustainable management Mongolia under changing conditions.
Language: Английский
Citations
1Ecological Indicators, Journal Year: 2023, Volume and Issue: 146, P. 109892 - 109892
Published: Jan. 13, 2023
Accurate estimation of aboveground biomass grasslands is key to sustainable grassland utilization. However, most satellites cannot provide high temporal and spatial resolution data. Patterns dynamics associated with variability in climate conditions across spatiotemporal scales are yet be adequately quantified. A fusion model offers the opportunity combine advantages different remote sensing data achieve a frequency precision monitoring vegetation. We test flexible (FSDAF) methodology generate synthetic normalized difference vegetation index (NDVI) from Moderate-Resolution Imaging Spectroradiometer (MODIS) Landsat sets. The tested for semi-arid Xilin River Basin, China. Based on NDVI field measured an established watershed. Exploring changes its relationship environmental factors. results show that: (1) FSDAF performs well (R2 = 0.75) has clear textural features. (2) Support Vector Machine Aboveground Biomass not only ensured accuracy 0.78, RMSE 15.43 g/m2), but also generated maps higher (30 m) (8 days). (3) this area decreases southeast northwest, reaches peak at end July. average order meadow > typical desert grassland. (4) increased linearly increasing water content, organic carbon total nitrogen, was sensitive soil content. During early growing rapid period, mainly affected by both air temperature precipitation, while effects human activities gradually dominate middle late periods. This study helps improve dynamic biomass, provides scientific basis protection management arid regions.
Language: Английский
Citations
19Agronomy, Journal Year: 2023, Volume and Issue: 13(12), P. 3040 - 3040
Published: Dec. 12, 2023
The agricultural sector is currently confronting multifaceted challenges such as an increased food demand, slow adoption of sustainable farming, a need for climate-resilient systems, resource inequity, and the protection small-scale farmers’ practices. These issues are integral to security environmental health. Remote sensing technologies can assist precision agriculture in effectively addressing these complex problems by providing farmers with high-resolution lenses. use vegetation indices (VIs) essential component remote sensing, which combines variability spectral reflectance value (derived from data) growth stage crops. A wide array VIs be used classify crops evaluate their state However, precisely this high number leads difficulty selecting best VI combination specific objectives. Without thorough documentation analysis appropriate VIs, users might find it difficult data or obtain results very low accuracy. Thus, objective review conduct critical existing art on effective discrimination monitoring several important (wheat, corn, sunflower, soybean, rape, potatoes, forage crops), grasslands meadows. This could highly useful all stakeholders involved activities. current has shown that appear suitable mapping crops, meadows pastures. Sentinel-1 Sentinel-2 were most utilized sources, while some frequently EVI, LAI, NDVI, GNDVI, PSRI, SAVI. In studies, needed employed achieve good prediction yields. main using related variation characteristics during period similarities signatures various semi-natural further studies establish models satellite would prove have greater accuracy provide more relevant information efficient
Language: Английский
Citations
19Ecological Indicators, Journal Year: 2023, Volume and Issue: 155, P. 110925 - 110925
Published: Sept. 15, 2023
Ecological degradation is a global issue that seriously threatens sustainable human development, and the evaluation of ecosystem health (EH) primary condition for regional ecological restoration work. In this study, we optimized classic vigor organization resilience (VOR) model analyzed spatial distribution pattern index (EHI) in Sichuan Province 2020 2005. We determined land zone (ESZ), (EDZ), improvement (EIZ) Province. The results indicate (1) kappa values VOR models 2005 were 33.84 32.74, respectively, which below 100. (2) ranges EHI 0.238–0.892 0.218–0.896, respectively. (3) areas EDZ EIZ 41781.84 26032 km2, accounting 8.58 5.35% total area Province, (4) ESZ accounts 36.46% This study beneficial eco-environmental management departments to develop strategies based on local ESZ, EDZ, EIZ.
Language: Английский
Citations
18International Journal of Applied Earth Observation and Geoinformation, Journal Year: 2023, Volume and Issue: 124, P. 103533 - 103533
Published: Oct. 22, 2023
Precision agriculture management with remote sensing big data provides a promising solution to monitor crops. Alfalfa is an important forage crop for various livestock around the world. Unlike corn and soybean, alfalfa growth difficult describe using typical phenological curve since it characterized by monthly harvest rapid regrowth. Limited availability of high spatio-temporal resolution from single satellite sensor, there have been few studies reported on remotely monitoring alfalfa. Fusion Landsat/Sentinel-2 optical Sentinel-1 Synthetic Aperture Radar (SAR) can improve data. However, form unified SAR image multisource satellites, are still four issues during fusion. To overcome these challenges, this study proposes improved fusion Landsat-7/8, Sentinel-2, The framework includes three models, namely Landsat2Sentinel-2, SAR2Optical, Optical2SAR. results indicate that random forest (RF) algorithm known spectral bands, Landsat2Sentinel-2 model improves Landsat-based surface red edge reflectance root mean square error (RMSE) reduced 28.22–31.16 % compared multiple linear regression models. In addition, RMSE was further 22.61–23.58 if considering solar angles in RF algorithm. Compared traditional model, more accurately fused vegetation indices (VIs) derived Landsat Sentinel-2 46.81–51.16 %. SAR2Optical VIs 32.18–34.59 benefited involved climate Optical2SAR generated optical-based data, proving be reciprocally fused. Moreover, developed models present accuracy fusing at both top-of-atmosphere (TOA) levels. On other hand, proposed used build across different types. Overall, demonstrated has great potential crops satellites.
Language: Английский
Citations
17Remote Sensing of Environment, Journal Year: 2023, Volume and Issue: 301, P. 113935 - 113935
Published: Dec. 12, 2023
Monitoring soil erosion in the Arctic tundra is complicated by highly fragmentated nature of landscape and limited spatial resolution even high-resolution satellite data. The expansion shrubs across has led to substantial changes vegetation composition that alter spectral reflectance directly affect indices such as normalized difference index (NDVI), which widely applied for environmental monitoring. This change can mask if datasets with too coarse resolutions are used, increases NDVI driven shrub obscure concurrent barren land cover. Here we created cover maps from a multispectral uncrewed aerial vehicle (UAV) survey assessed imagery PlanetScope, Sentinel-2 Landsat-8 several areas north-eastern Iceland. Additionally, used novel application Shannon evenness (SHEI) evaluate levels pixel mixing. Our results show lead confusion, processes emphasize importance considering when monitoring fragmented landscapes. We demonstrate remote sensing data < 3 m greatly improves amount information captured an Icelandic environment. Landsat (30 m) inadequate our study area. found best platform combination UAV acquisitions validation. potential improve capabilities introducing use SHEI assess mixing determine optimal resolutions. approach combined comparing different time scales significantly advances comprehension changes, including greening degradation, tundra.
Language: Английский
Citations
17Ecological Informatics, Journal Year: 2024, Volume and Issue: 80, P. 102472 - 102472
Published: Jan. 20, 2024
Language: Английский
Citations
8Ecological Informatics, Journal Year: 2024, Volume and Issue: 82, P. 102768 - 102768
Published: Aug. 10, 2024
Fractional Vegetation Cover (FVC) serves as a crucial indicator in ecological sustainability and climate change monitoring. While machine learning is the primary method for FVC inversion, there are still certain shortcomings feature selection, hyperparameter tuning, underlying surface heterogeneity, explainability. Addressing these challenges, this study leveraged extensive field data from Qinghai-Tibet Plateau. Initially, selection algorithm combining genetic algorithms XGBoost was proposed. This integrated with Optuna tuning method, forming GA-OP combination to optimize learning. Furthermore, comparative analyses of various models inversion alpine grassland were conducted, followed by an investigation into impact heterogeneity on performance using NDVI Coefficient Variation (NDVI-CV). Lastly, SHAP (Shapley Additive exPlanations) employed both global local interpretations optimal model. The results indicated that: (1) exhibited favorable terms computational cost accuracy, demonstrating significant potential tuning. (2) Stacking model achieved among seven (R2 = 0.867, RMSE 0.12, RPD 2.552, BIAS −0.0005, VAR 0.014), ranking follows: > CatBoost LightGBM RFR KNN SVR. (3) NDVI-CV enhanced result reliability excluding highly heterogeneous regions that tended be either overestimated or underestimated. (4) revealed decision-making processes perspectives. allowed deeper exploration causality between features targets. developed high-precision scheme, successfully achieving accurate proposed approach provides valuable references other parameter inversions.
Language: Английский
Citations
8Journal of Environmental Management, Journal Year: 2023, Volume and Issue: 350, P. 119694 - 119694
Published: Nov. 29, 2023
Language: Английский
Citations
16